Abstract
Multiple reports now describe changes to the DNA methylome in rheumatoid arthritis and in many cases have analyzed methylation in mixed cell populations from whole blood. However, these approaches may preclude the identification of cell type-specific methylation, which may subsequently bias identification of disease-specific changes. To address this possibility, we conducted genome-wide DNA methylation profiling using HumanMethylation450 BeadChips to identify differences within matched pairs of T-lymphocytes and B-lymphocytes isolated from the peripheral blood of 10 healthy females. Array data were processed and differential methylation identified using NIMBL software. Validation of array data was performed by bisulfite pyrosequencing. Genome-wide DNA methylation was initially determined by analysis of LINE-1 sequences and was higher in B-lymphocytes than matched T-lymphocytes (69.8% vs. 65.2%, P ≤ 0.01). Pairwise analysis identified 679 CpGs, representing 250 genes, which were differentially methylated between T-lymphocytes and B-lymphocytes. The majority of sites (76.6%) were hypermethylated in B-lymphocytes. Pyrosequencing of selected candidates confirmed the array data in all cases. Hierarchical clustering revealed perfect segregation of samples into two distinct clusters based on cell type. Differentially methylated genes showed enrichment for biological functions/pathways associated with leukocytes and T-lymphocytes. Our work for the first time shows that T-lymphocytes and B-lymphocytes possess intrinsic differences in DNA methylation within a restricted set of functionally related genes. These data provide a foundation for investigating DNA methylation in diseases in which these cell types play important and distinct roles.
Introduction
DNA methylation, one of several prototypical epigenetic mechanisms, regulates gene expression without imposing alterations to the underlying nucleotide sequence. It is important in a variety of fundamental biological processesCitation1-Citation3 and in humans occurs almost exclusively on cytosines in the context of cytosine-guanine dinucleotides (CpGs). These dinucleotides are found to cluster at high density in regions of DNA termed CpG islands, and are often associated with gene promoters.Citation4,Citation5 With particular exceptions, they are typically not methylated in transcriptionally competent genes.Citation6 Conversely, methylation of promoter-associated CpGs is frequently associated with transcriptional repression and gene silencing.Citation6
There is now compelling evidence that aberrant DNA methylation is involved in a variety of human diseases, most notably in cancer etiology and, more recently, a growing spectrum of autoimmune disorders that include rheumatoid arthritis (RA). Distinct leukocyte populations, particularly lymphocytes and their subsets, play a pivotal role in RA pathogenesis, driving autoimmunity and chronic inflammation. Levels of global DNA methylation have been found to be altered in lymphocytes and peripheral blood mononuclear cells from patients with RA.Citation7-Citation9 Moreover, others have also reported aberrant methylation in RA, but in these cases have examined individual genes and using DNA samples derived from whole blood or the mononuclear cell fraction.Citation10-Citation15 Although these studies have identified genes potentially involved in RA pathogenesis, they are not able to consider the distinct roles of individual leukocyte populations. Furthermore, analysis of mixed cell samples may preclude the identification of important cell type-specific and disease-specific methylation changes due to the inherent cellular heterogeneity. Indeed, this view is supported by recent evidence indicating that leukocyte heterogeneity may be a confounder for DNA methylation analyses on whole blood,Citation16 and that individual leukocyte populations show evidence of differences in methylation.Citation17 In this context, a recent genome-wide study in RA by Liu et al.Citation18 utilized a statistical algorithm to estimate and adjust for blood leukocyte proportions, concluding this to be a critical step for downstream bioinformatic analyses.
It is clear therefore that the examination of individual leukocyte populations that drive disease is an important step toward better understanding the role of DNA methylation in conditions such as RA. A prerequisite to this goal must first be to define the intrinsic differences in methylation between individual leukocyte populations. In the current work, we have taken a stringent methodological approach to address these issues by defining genome-wide DNA methylation profiles in highly purified matched pairs of T-lymphocytes and B-lymphocytes isolated from the blood of healthy individuals.
Results
450K array technical validation
Genome-wide DNA methylation at 485 577 CpG sites (99% of RefSeq genes) was examined using the HumanMethylation450 BeadChip (henceforth referred to as “450K array” or “array”; Illumina Inc.) to identify differences in methylation between matched pairs of T-lymphocytes and B-lymphocytes isolated from the blood of ten healthy females. We first performed a technical validation of the array data using an independent technique, in this case, sodium bisulfite pyrosequencing. To this end, five CpGs were selected at random, from three separate genes (AMN, HMOX2, and PM20D1), which represented the spectrum of possible array β-values (0 to 1). These sites, which were analyzed in paired samples from seven of the ten individuals chosen randomly, revealed excellent correlation between array β-values and methylation determined by pyrosequencing (Spearman’s r = 0.952, P < 0.00001) (). Since the correlation was apparent across the spectrum of β-values it is unlikely to be consequent to the dominance of extreme values.Citation19
Figure 1. Technical validation of 450K array data by sodium bisulfite pyrosequencing. Correlation of DNA methylation as measured by array β-value and by bisulfite pyrosequencing for 70 individual sites in T-lymphocytes and B-lymphocytes from seven of the ten healthy individuals (5 CpGs were selected at random, from three separate genes: HMOX2: cg14951292, AMN: cg09616556, PM20D1: cg24503407, cg07167872, cg11965913). T- and B-lymphocytes are shown as circles and crosses, respectively. Spearman’s r = 0.952, P < 0.000 01.
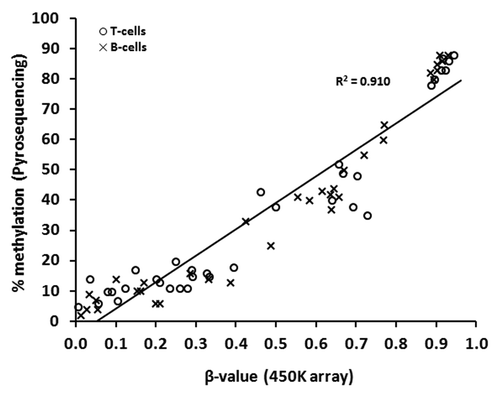
Assessment of global DNA methylation
Methylation of the LINE-1 retrotransposon is frequently used as a surrogate measure of global DNA methylation levels.Citation20,Citation21 Using sodium bisulfite pyrosequencing we quantified methylation across 3 adjacent CpG sites within the LINE-1 sequence. Mean LINE-1 methylation was similar in each individual for each specific cell type; however, a subtle difference between the cell types was observed, 65.2% in T-lymphocytes and 69.8% in B-lymphocytes (). Similar levels of methylation have been reported for peripheral blood leukocytes.Citation22 We noted that LINE-1 methylation was consistently higher in B-lymphocytes for each of the three CpGs inspected. Although these differences were subtle they were statistically significant at individual sites and also collectively (P ≤ 0.01, Wilcoxon Signed Rank Test; ). A more gene-focused assessment of global methylation by calculating the average β-value across all array CpGs revealed no significant difference between the cell types (β = 0.562 and 0.558 in T-lymphocytes and B-lymphocytes, respectively, P > 0.2).
Figure 2. LINE-1 DNA methylation in matched pairs of T-lymphocytes and B-lymphocytes. Sodium bisulfite pyrosequencing was used to quantify methylation at three CpG sites within LINE-1 repetitive sequences in purified T-lymphocytes and B-lymphocytes from ten healthy individuals. T- and B-lymphocytes are shown as circles and crosses, respectively. The mean level of methylation for each CpG site in each cell population is shown by the short black horizontal bar in each case. * P ≤ 0.01 (Wilcoxon Signed-Rank Test).
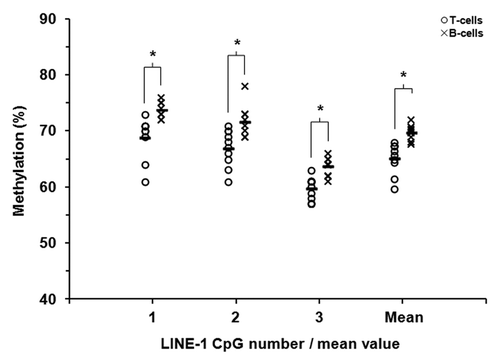
A restricted set of differentially methylated CpGs distinguish T-lymphocytes and B-lymphocytes
After initial array processing (described in the Methods), the remaining data set comprised 484 616 CpGs. For robust identification of CpG loci displaying differential methylation between the two cell types, we first performed a series of filtering steps in which only those CpGs meeting stringent criteria were retained. The filtering criteria and number of CpGs remaining at each of the steps are presented in . As a first step to improve the power of subsequent statistics, non-variable sites (CpGs for which β-values were either ≤ 0.2, or ≥ 0.8, in all 20 samples) were removed, an approach we and others have previously employed.Citation23-Citation25 For initial identification of CpGs showing a difference in methylation, we calculated the difference in β-value between B-lymphocytes and T-lymphocytes for each of the ten paired samples. We next calculated the mean β-value difference and retained only those CpGs with a mean difference ≥ 0.2 (step 2; ). Implementation of the subsequent steps resulted in the identification of 679 gene-associated CpGs displaying significant differences in methylation between T-lymphocytes and B-lymphocytes from the ten healthy individuals examined.
Figure 3. Criteria for filtering and identification of CpGs differentially methylated between paired T-lymphocyte and B-lymphocyte samples. The starting number of 484,616 CpGs was derived through removal of CpGs with high detection P values (P > 0.05) and those with missing β-values, as described in the Methods. †, “change” refers to a methylation difference that fulfilled all preceding filtering steps; ‡, “change in the same direction” refers to multiple CpGs from one gene all showing either hyper- or hypo-methylation in one cell type as compared with the other cell type.
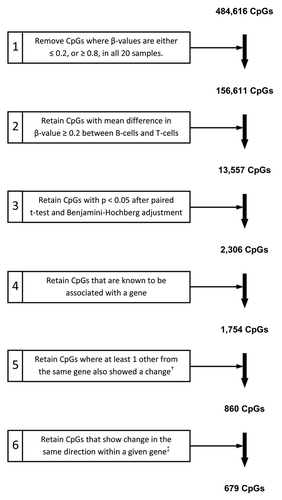
Hierarchical clustering and validation of candidate CpGs
We next used hierarchical clustering to establish whether the β-value methylation profile for the 679 CpGs identified above was able to distinguish between the two cell types across the ten individuals. As presented in the heatmap and dendrograms in , a perfect dichotomy was observed, with T-lymphocyte and B-lymphocyte samples segregating into two distinct clusters (indicated in the heatmap by green and red bars, respectively). Moreover, we observed this dichotomy when clustering was performed on the number of CpGs identified at each successive stage of the filtering process, from step 3 onward and identifying in this case 2306 statistically significant CpGs (Fig. S1). The data therefore clearly show that the observed differences in methylation are highly consistent between the T- and B-lymphocytes in each of the ten individuals examined.
Figure 4. Heatmap and clustering for the 679 CpGs identified as differentially methylated. CpG sites identified by 450K array analysis as differentially methylated between paired T-lymphocytes (green bar) and B-lymphocytes (red bar) were analyzed by hierarchical clustering. Each row represents an individual CpG site and each column a different sample (listed beneath the heatmap). Branches of the dendrograms indicate similarity between CpGs (rows) and samples (columns). Color gradation from yellow to blue represents low to high DNA methylation respectively, with β-values ranging from 0 (no methylation; yellow) to 1 (complete methylation; blue). Sample order, left-to-right: B-lymphocytes: HC03, 10, 04, 09, 11, 14, 08, 12, 16, 17; and T-lymphocytes: HC03, 08, 09, 11, 12, 04, 14, 16, 10, 17.
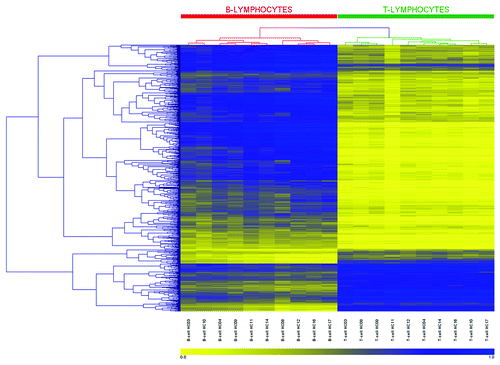
Closer inspection of the 679 CpGs revealed that the majority (520 CpGs; 76.6%) showed higher methylation in B-lymphocytes (summary details are provided in ). The observed differences in mean β-value were in many cases pronounced (maximum difference of 0.86) and approximately half of the CpGs identified demonstrated a β-value difference of at least 0.5 (326; 48.0%) and/or were associated with a bona fide CpG island (326; 48.0%).Citation26 In total, 250 genes were represented by the 679 CpGs, with a maximum of 18 CpGs in any one gene (a complete list of CpGs/genes and associated β-values is provided in Table S1). identifies the 20 genes displaying the largest methylation differences between the cell types (top 10 hypermethylated genes in each cell type). Of particular note, numerous genes showing high methylation in B-lymphocytes and low methylation in T-lymphocytes were those with known T-lymphocyte-specific expression/function, such as the CD3D and CD3G genes (subunits of the CD3 T-cell co-receptor).
Table 1. Summary characteristics of the 679 CpGs identified as differentially methylated between paired T-lymphocyte and B-lymphocyte samples by genome-wide DNA methylation analysis using 450K arrays
Table 2. Annotation for the top 10 hypermethylated genes in T-lymphocytes and top 10 hypermethylated genes in B-lymphocytes, as determined by genome-wide DNA methylation analysis
For independent validation of the candidate genes identified in our genome-wide approach, we selected eight which showed large methylation differences between the cell types and which also contained at least 2 differentially methylated CpGs that could be sequenced simultaneously; four genes that were hypermethylated in B-lymphocytes (CD3G/D, DENND2D, GIMAP7, and PITPNC1) and four that were hypermethylated in T-lymphocytes (CLPTM1L, DDAH2, RNH1, and SLC7A5). Validation was performed by sodium bisulfite pyrosequencing (assay details are provided in Table S2). displays data for one representative CpG from each gene examined. In each case, the large statistically significant differences observed between the cell types by array β-values were accurately replicated by pyrosequencing (P < 0.01 for each CpG/gene). This was also true for each of the CpGs analyzed in each gene, as exemplified by the multiple CpGs in RNH1 and PITPNC1 (P < 0.01; ). Validation across these eight candidate genes reinforces the robustness of the array data and our approach to identify sites differentially methylated between T-lymphocytes and B-lymphocytes.
Figure 5. Validation of differentially methylated CpG candidates identified by 450K array analysis. Sodium bisulfite pyrosequencing was used to confirm candidate genes/CpGs that were differentially methylated between T-lymphocytes and B-lymphocytes in all ten healthy individuals. CpG sites in four genes that were hypermethylated in T-lymphocytes (A) and four genes that were hypermethylated in B-lymphocytes (B) were selected from the array candidates. T- and B-lymphocytes are shown by circles and crosses, respectively. Gene names are shown on the x-axis, where for each gene methylation values are shown for the array (left) and pyrosequencing (right). The mean values are defined by the horizontal black bar in each case. The methylation differences observed between T-lymphocytes and B-lymphocytes by pyrosequencing analysis were statistically significant for each of the eight CpGs/genes examined (P < 0.01, Wilcoxon Signed-Rank Test). Abbreviations: Pyro., bisulfite pyrosequencing.
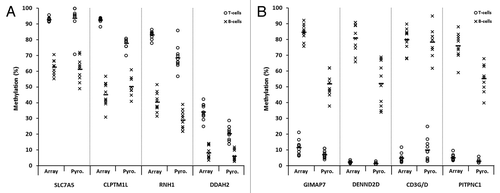
Figure 6. Representative plots displaying validation of multiple array CpGs within two candidate genes by sodium bisulfite pyrosequencing analysis. Four CpGs within the RNH1 gene (A) and three within PITPNC1 (B), all of which demonstrated significant differences in methylation between T-lymphocytes and B-lymphocytes by array analysis, were examined. T- and B-lymphocytes are shown as circles and crosses, respectively. CpG identifiers are shown on the x-axis. In each case, the first site shown for RNH1 (A) and PITPNC1 (B) is the site depicted in , respectively. For each site, methylation values are shown for the array (left) and pyrosequencing (right). The mean values are defined by the horizontal black bar in each case. The CpG sites are displayed in the 5′ to 3′ direction, with the four sites in RNH1 covering 20-bp and three sites in PITPNC1 covering 45-bp. The methylation differences observed between T-lymphocytes and B-lymphocytes by pyrosequencing analysis were statistically significant for each of the CpGs in each gene examined (P < 0.01, Wilcoxon Signed-Rank Test). Abbreviations: Pyro., bisulfite pyrosequencing.
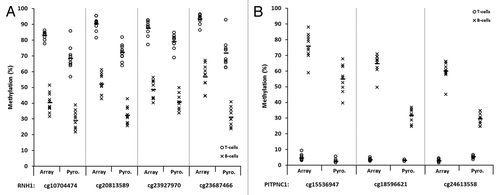
Differentially methylated genes show enrichment for immune and leukocyte-specific characteristics
To explore the functional characteristics of genes identified as differentially methylated between T-lymphocytes and B-lymphocytes, we examined gene ontologies and biological pathways using the online software DAVID (Database for Annotation, Visualization and Integrated Discovery).Citation27,Citation28 Results from these analyses are provided in Tables S3–S5. Analysis of the 250 candidate genes revealed significant enrichment for multiple ontology terms (Table S3). Thirty-two terms remained significant following adjustment for multiple comparisons and, of note, half of these (16/32) specifically related to “T-cell,” “Lymphocyte,” or “Leukocyte” categories. Similar results identifying T-lymphocyte, leukocyte and immune related categories were obtained when we performed clustering on annotation terms (for example “T-cell receptor complex” and “T-cell activation”; Table S4), and also when we interrogated the gene set for enrichment of biological pathways (for example “T-cell receptor signaling pathway” and “T helper cell surface molecules”; Table S5). Genes displaying disparate methylation between the cell types are thus enriched for lymphocyte-related functions.
Discussion
DNA methylation represents one of several principal targets for epigenetic change and acts in concert with other epigenetic modifications to regulate gene expression. A burgeoning literature describes these types of change in multiple diseases, including those with an autoimmune component such as RA, in which leukocytes are known to play a pivotal role. The majority of previous studies examining methylation in leukocytes from RA patients have employed DNA extracted from whole blood or the mononuclear cell fraction.Citation7-Citation15,Citation18 However, in these cases cellular heterogeneity may be a confounding factor. To determine if intrinsic differences in methylation are a feature of individual leukocyte populations, we compared genome-wide DNA methylation profiles in matched pairs of T-lymphocytes and B-lymphocytes isolated from the blood of healthy individuals. Through this approach, our unequivocal observation is that a restricted set of CpGs in functionally-related genes show differential methylation between T-lymphocytes and B-lymphocytes, and define a DNA methylation signature that accurately distinguishes the two lymphocyte types.
We identified a set of 679 gene-associated CpGs, representing 250 unique genes, which displayed altered methylation between T-lymphocytes and B-lymphocytes. For many of these sites striking differences in methylation were observed (β-value differences up to 0.864), resulting in the perfect segregation of T- and B-lymphocytes into two distinct clusters. In common with other tissues/cell types, the majority of CpG islands in T-lymphocytes and B-lymphocytes are unmethylated.Citation34,Citation35 There is also evidence from a variety of different tissues and cell types, including leukocytes, that some CpG islands show tissue-specific methylation.Citation36,Citation37 In our study, a small proportion (9%) of the 679 CpGs differentially methylated between T- and B-lymphocytes were located within a bona fide CpG island (as defined by Takai and JonesCitation26). Of interest however, approximately three-times as many CpGs (25.3%) resided within the associated CpG island shores, with a further 13.7% within island shelves. Thus, our data indicate that the majority of intrinsic differences in methylation between T-lymphocytes and B-lymphocytes occur not within CpG islands per se but within the shores surrounding these islands (≤ 2 kb from the island). Of particular note, the importance of these CpG island “associated regions” in the context of gene expression has recently been describedCitation38 and is worthy of further investigation. Interestingly, analysis of global DNA methylation, using methylation of the LINE-1 retrotransposon as a surrogate measure, revealed marginal, yet statistically significant higher methylation in B-lymphocytes. To our knowledge this comparative increase has not previously been identified, and it is currently unclear as to its significance.
Those genes identified as unmethylated in T-lymphocytes but hypermethylated in B-lymphocytes included a significant number with known T-lymphocyte expression and functionality, such as ITK (a T-lymphocyte kinase), CD2 (early T-lymphocyte marker), and the genes for the delta, gamma, and epsilon subunits of the CD3 T-cell co-receptor (CD3D, CD3G, and CD3E, respectively) which is expressed exclusively on T-lymphocytes. Although not experimentally addressed in this study, our data suggest methylation-based epigenetic regulation of the CD3 locus, a finding that has recently been reported for leukocyte subsets.Citation17 Analysis of functional enrichment by examining gene ontologies and pathways confirmed the predominance of genes associated with lymphocyte and leukocyte functioning among those differentially methylated between the lymphocyte types. Similar enrichment of leukocyte/lymphocyte genes was previously reported by Reinius and colleagues,Citation17 but in individual comparisons of each cell type with methylation in whole blood.
In our investigation and for the purpose of defining intrinsic genome-wide differences in methylation between T-lymphocytes and B-lymphocytes, we adopted a stringent methodological approach. First, we isolated the individual cell populations using a validated technique that achieved mean purity in excess of 99% and 93% for each cell type respectively. Second, we implemented a series of stringent filtering criteria and independent validation to robustly identify those sites/genes displaying the most significant differences in methylation between the lymphocyte types. Finally, we only considered a gene to show altered methylation between the cell types if at least two CpGs from the same gene demonstrated statistically significant β-value differences ≥ 0.2 and in the same direction (i.e., higher/lower in the same cell type), as used with success in a previous investigation from our laboratory.Citation23 Independent validation of 19 CpGs from eight selected candidate genes confirmed the large differences observed, and emphasizes the robustness of our data.
Previous studies have reported subtle but significant differences in DNA methylation between tissue-types and between individuals,Citation25 and additional reports provide evidence for associations of this epigenetic change with demographical factors such as age, gender and ethnicity,Citation39,Citation40 and lifestyle factors including smoking.Citation41,Citation42 Herein, and partly prompted by this literature, we focused our analyses on two lymphocyte populations in a carefully selected and closely matched, albeit small, cohort of healthy individuals (Caucasian, non-smoking females, approximately 50–55 y of age). With regard to the limited variation in subject age, inspection of the results from hierarchical clustering revealed no evidence to suggest that younger and older individuals clustered separately. We are confident therefore, that our findings are not unduly influenced by potentially confounding variables such as those discussed above.
The important issue of cellular heterogeneity as a confounder for DNA methylation analyses of blood-derived DNA has previously been recognized.Citation16-Citation18 Indeed, in the context of disease, underlying changes in the proportion of the different leukocyte populations may bias the identification of disease-specific changes in methylation. To address this issue a statistical algorithm has recently been describedCitation43 and provides an accurate method to estimate and adjust for leukocyte proportions in genome-wide DNA methylation studies.Citation18,Citation43 However, although this approach represents a significant advance, it is not able to determine the particular leukocyte populations in which disease-specific changes in DNA methylation occur. In these cases these changes represent an important question for diseases where different, specific, leukocyte populations play central roles in the disease process. In our own study, we have first set out to establish the intrinsic differences in methylation between matched pairs of purified T- and B-lymphocytes from healthy individuals. In this way we provide a foundation from which disease-specific changes of DNA methylation in these cell types may be determined.
In summary, we have presented a unique genome-wide DNA methylation signature that accurately distinguishes healthy T-lymphocytes from B-lymphocytes. We recognize that it will be important for future efforts to define methylation levels and disease-specific aberrations in these cell populations in RA patients, and to perhaps extend these types of analyses to individual cell subsets. The different T-helper subsets, for example, will be of particular interest given their distinct and multifaceted role in RA.
Materials and Methods
Patients
Peripheral blood samples (30 ml, EDTA) were collected from 10 healthy female Caucasians. Donors were recruited from the hospital staff population at the Haywood Rheumatology Centre, Stoke-on-Trent, and gave prior written informed consent. Each donor completed a short questionnaire to establish basic demographical information and to provide information on their current health status. The average age of the group was 52.1 ± 5.0 y (mean ± SD) and none were current smokers. Individuals who reported any musculoskeletal or inflammatory-related conditions were excluded. The study was approved by the East Midlands (Derby) Research Ethics Committee.
Isolation of individual lymphocyte populations
Peripheral blood mononuclear cells were isolated from fresh whole blood samples by density gradient centrifugation using Histopaque-1077 (Sigma-Aldrich). CD19+ B-lymphocytes were purified from the mononuclear cell fraction using anti-CD19 magnetic microbeads, with subsequent isolation of CD3+ T-lymphocytes from the negative portion using anti-CD3 magnetic microbeads (MiniMACS® separation system; Miltenyi Biotec). We chose to use positive selection as this has previously been demonstrated to be superior for array-based analyses of leukocytesCitation44 and has minimal effects on leukocyte activation.Citation45-Citation48 All separations were performed according to the manufacturer’s instructions (Miltenyi Biotec). Cell preparations were immediately pelleted, lysed and homogenized (QIAShredder columns; Qiagen). Lysates were stored at –80 °C.
Antibody staining and flow cytometry
CD3+ T-lymphocytes and CD19+ B-lymphocytes from the ten donors were assessed for purity using flow cytometry. T-lymphocytes were stained with a murine phycoerythrin (PE)-labeled anti-CD3 IgG monoclonal antibody, and B-lymphocytes with a murine PE-anti-CD20 IgG monoclonal antibody. Each sample was separately stained with the appropriate PE-labeled isotype-matched control antibody. Antibodies were purchased from Miltenyi Biotec. Samples were analyzed on a FACSort flow cytometer and raw data collected using CellQuest software (BD Biosciences). A forward scatter threshold of 180 was set and 10 000 events/sample were counted. Data were analyzed using Cyflogic software (version 1.2.1; CyFlo Ltd). Representative histograms of cell purity for each cell type are provided in Fig. S2. The mean ± SD purity for T-lymphocytes and B-lymphocytes from the ten donors was 99.6 ± 0.4% and 93.6 ± 3.4%, respectively.
Genome-wide DNA methylation analysis by Infinium 450K array
Genomic DNA was extracted from thawed lysates using an AllPrep DNA/RNA Mini kit (Qiagen) according to the manufacturer’s instructions. Further purification and concentration of DNA was performed using ethanol-based precipitation reactions. DNA concentration was assessed using a NanoDrop ND-1000 spectrophotometer (Thermo Scientific).
Quantification of genome-wide DNA methylation was conducted using Infinium-based HumanMethylation450 BeadChips (Illumina Inc.). Each array enables simultaneous quantification of methylation at 485 577 unique CpGs across the genome, representing 21 231 RefSeq genes (99% coverage), in 12 independent samples in parallel.Citation49 Array hybridizations were performed by Gen-Probe Life Sciences Ltd according to Illumina recommended protocols. Briefly, 650 ng of genomic DNA was sodium bisulfite converted using an EZ DNA Methylation Kit (Zymo Research). Subsequently, 4 µl of bisulfite-converted DNA was hybridized to 450K arrays following the Illumina Infinium HD Methylation protocol. Each sample was arrayed once. Arrays were scanned using an Illumina iScan and image intensities extracted using the GenomeStudio Methylation Module (version 1.8.5). The methylation status of each CpG/probe was reported as a methylation β-value, where β is defined as the ratio of the methylated signal intensity over the summed intensity of the methylated and unmethylated signals + 100.Citation49 β-values were reported on a continuous linear scale ranging from 0 (unmethylated) to 1 (completely methylated).
Whole genome amplification and touchdown PCR
Sodium bisulfite-converted DNA samples used on the arrays were separately used for validation of array data and to confirm the methylation status of identified candidate CpGs/genes. To increase template quantity, samples were first subjected to whole genome amplification according to a protocol described by Mill et al.Citation50 Briefly, 1 µl bisulfite-converted DNA was amplified by polymerase chain reaction (PCR) using GoTaq Flexi DNA polymerase and buffer (Promega), dNTPs (Bioline), and random 15-mer oligonucleotide primers (200 pmoles; Life Technologies). Thermal cycling conditions were: 98 °C for 3 min, followed by 50 cycles of 98 °C for 1 min, 37 °C for 2 min and 55 °C for 4 min, with a ramping rate of 0.1 °C/second prior to each denaturation and extension step.
PCR amplicons that encompassed CpGs of interest were generated from whole genome amplified DNA using touchdown PCR.Citation51,Citation52 Forward, reverse and sequencing primers specific for bisulfite-converted DNA were designed using PyroMark Assay Design software (version 2.0; Qiagen) and were purchased from Biomers.net (primer sequences are provided in Table S2). In each 25 µl PCR reaction, 2–4 µl whole genome-amplified DNA was amplified with the appropriate forward and reverse primers, as we have described previously.Citation53
Validation by sodium bisulfite pyrosequencing
Methylation at individual CpG sites was quantified by pyrosequencing of bisulfite-converted PCR-amplified DNA using a PyroMark Q24 instrument (Qiagen). Pyrosequencing was performed according to the manufacturer’s instructions and as we have previously described.Citation23,Citation53 The sequence to analyze for each CpG/gene is provided in Table S2 and all assays included one or more control dispensations to confirm sequence identity and completeness of bisulfite conversion. Data were analyzed using PyroMark Q24 software (v 2.0.6., build 20; Qiagen).
Data analysis
Array data were analyzed using NIMBL (Numerical Identification of Methylation) software.Citation54 Each array passed initial quality control assessment based on the performance of internal array controls, and the distribution of β-values across all 485 577 CpGs was found to be similar in each sample. All CpGs for which one or more of the 20 samples displayed detection P values > 0.05 (indicating an unreliable site) or presented with missing β-values were excluded. As we have described previously, we considered it a more robust approach to remove from the data set CpGs that failed in any one of the samples rather than excluding individual failed CpGs from specific samples.Citation24 To adjust for the observation that β-values derived from Infinium II probes on the 450K array can be less sensitive than those generated from Infinium I probes, NIMBL was used to perform ‘peak-based correction’ as devised by Dedeurwaerder et al.Citation55
Criteria for identification of differentially methylated CpGs are described in detail in the Results. Statistical analysis of DNA methylation at individual CpG sites was compared between T-lymphocytes and B-lymphocytes using paired t-tests. Correction for multiple testing was performed using the false discovery rate (FDR) adjustment of Benjamini and Hochberg,Citation29 with adjusted P values < 0.05 considered significant. Euclidian-based hierarchical clustering of differentially methylated CpGs was performed using Genesis software (v1.7.6).Citation56 Differentially methylated genes were assessed for enrichment of gene ontology terms and biological pathways using DAVID online software.Citation27,Citation28 Additional statistical analyses were performed using NCSS 2000 (NCSS LCC). P values < 0.05 were considered significant.
Abbreviations: | ||
CpG | = | cytosine-guanine dinucleotide |
RA | = | rheumatoid arthritis |
PCR | = | polymerase chain reaction |
450K array/array | = | HumanMethylation450 BeadChip |
NIMBL | = | numerical identification of methylation biomarker lists |
DAVID | = | database for annotation, visualization and integrated discovery |
Additional material
Download Zip (1.1 MB)Disclosure of Potential Conflicts of Interest
No potential conflicts of interest were disclosed.
Acknowledgments
The authors wish to thank Cuong V Duong and Dr Mark Kitchen for assistance with pyrosequencing assays. This work was supported by funding provided by the Haywood Rheumatism Research and Development Foundation.
Supplemental Materials
Supplemental materials may be found here: www.landesbioscience.com/journals/epigenetics/article/26265
References
- Li E, Bestor TH, Jaenisch R. Targeted mutation of the DNA methyltransferase gene results in embryonic lethality. Cell 1992; 69:915 - 26; http://dx.doi.org/10.1016/0092-8674(92)90611-F; PMID: 1606615
- Li E, Beard C, Jaenisch R. Role for DNA methylation in genomic imprinting. Nature 1993; 366:362 - 5; http://dx.doi.org/10.1038/366362a0; PMID: 8247133
- Mohandas T, Sparkes RS, Shapiro LJ. Reactivation of an inactive human X chromosome: evidence for X inactivation by DNA methylation. Science 1981; 211:393 - 6; http://dx.doi.org/10.1126/science.6164095; PMID: 6164095
- Bird AP. CpG-rich islands and the function of DNA methylation. Nature 1986; 321:209 - 13; http://dx.doi.org/10.1038/321209a0; PMID: 2423876
- Antequera F, Bird A. Number of CpG islands and genes in human and mouse. Proc Natl Acad Sci U S A 1993; 90:11995 - 9; http://dx.doi.org/10.1073/pnas.90.24.11995; PMID: 7505451
- Bird A. DNA methylation patterns and epigenetic memory. Genes Dev 2002; 16:6 - 21; http://dx.doi.org/10.1101/gad.947102; PMID: 11782440
- Richardson B, Scheinbart L, Strahler J, Gross L, Hanash S, Johnson M. Evidence for impaired T cell DNA methylation in systemic lupus erythematosus and rheumatoid arthritis. Arthritis Rheum 1990; 33:1665 - 73; http://dx.doi.org/10.1002/art.1780331109; PMID: 2242063
- Corvetta A, Della Bitta R, Luchetti MM, Pomponio G. 5-Methylcytosine content of DNA in blood, synovial mononuclear cells and synovial tissue from patients affected by autoimmune rheumatic diseases. J Chromatogr 1991; 566:481 - 91; PMID: 1939459
- Liu CC, Fang TJ, Ou TT, Wu CC, Li RN, Lin YC, Lin CH, Tsai WC, Liu HW, Yen JH. Global DNA methylation, DNMT1, and MBD2 in patients with rheumatoid arthritis. Immunol Lett 2011; 135:96 - 9; http://dx.doi.org/10.1016/j.imlet.2010.10.003; PMID: 20937307
- Schwab J, Illges H. Silencing of CD21 expression in synovial lymphocytes is independent of methylation of the CD21 promoter CpG island. Rheumatol Int 2001; 20:133 - 7; http://dx.doi.org/10.1007/s002960000090; PMID: 11411956
- Fu LH, Cong B, Zhen YF, Li SJ, Ma CL, Ni ZY, Zhang GZ, Zuo M, Yao YX. [Methylation status of the IL-10 gene promoter in the peripheral blood mononuclear cells of rheumatoid arthritis patients]. Yi Chuan 2007; 29:1357 - 61; http://dx.doi.org/10.1360/yc-007-1357; PMID: 17989045
- Nile CJ, Read RC, Akil M, Duff GW, Wilson AG. Methylation status of a single CpG site in the IL6 promoter is related to IL6 messenger RNA levels and rheumatoid arthritis. Arthritis Rheum 2008; 58:2686 - 93; http://dx.doi.org/10.1002/art.23758; PMID: 18759290
- Kitamura T, Kabuyama Y, Kamataki A, Homma MK, Kobayashi H, Aota S, Kikuchi S, Homma Y. Enhancement of lymphocyte migration and cytokine production by ephrinB1 system in rheumatoid arthritis. Am J Physiol Cell Physiol 2008; 294:C189 - 96; http://dx.doi.org/10.1152/ajpcell.00314.2007; PMID: 17942634
- Fu LH, Ma CL, Cong B, Li SJ, Chen HY, Zhang JG. Hypomethylation of proximal CpG motif of interleukin-10 promoter regulates its expression in human rheumatoid arthritis. Acta Pharmacol Sin 2011; 32:1373 - 80; http://dx.doi.org/10.1038/aps.2011.98; PMID: 21986577
- Ishida K, Kobayashi T, Ito S, Komatsu Y, Yokoyama T, Okada M, Abe A, Murasawa A, Yoshie H. Interleukin-6 gene promoter methylation in rheumatoid arthritis and chronic periodontitis. J Periodontol 2012; 83:917 - 25; http://dx.doi.org/10.1902/jop.2011.110356; PMID: 22122521
- Adalsteinsson BT, Gudnason H, Aspelund T, Harris TB, Launer LJ, Eiriksdottir G, Smith AV, Gudnason V. Heterogeneity in white blood cells has potential to confound DNA methylation measurements. PLoS One 2012; 7:e46705; http://dx.doi.org/10.1371/journal.pone.0046705; PMID: 23071618
- Reinius LE, Acevedo N, Joerink M, Pershagen G, Dahlén SE, Greco D, Söderhäll C, Scheynius A, Kere J. Differential DNA methylation in purified human blood cells: implications for cell lineage and studies on disease susceptibility. PLoS One 2012; 7:e41361; http://dx.doi.org/10.1371/journal.pone.0041361; PMID: 22848472
- Liu Y, Aryee MJ, Padyukov L, Fallin MD, Hesselberg E, Runarsson A, Reinius L, Acevedo N, Taub M, Ronninger M, et al. Epigenome-wide association data implicate DNA methylation as an intermediary of genetic risk in rheumatoid arthritis. Nat Biotechnol 2013; 31:142 - 7; http://dx.doi.org/10.1038/nbt.2487; PMID: 23334450
- Roessler J, Ammerpohl O, Gutwein J, Hasemeier B, Anwar SL, Kreipe H, Lehmann U. Quantitative cross-validation and content analysis of the 450k DNA methylation array from Illumina, Inc. BMC Res Notes 2012; 5:210; http://dx.doi.org/10.1186/1756-0500-5-210; PMID: 22546179
- Yang AS, Estécio MR, Doshi K, Kondo Y, Tajara EH, Issa JP. A simple method for estimating global DNA methylation using bisulfite PCR of repetitive DNA elements. Nucleic Acids Res 2004; 32:e38; http://dx.doi.org/10.1093/nar/gnh032; PMID: 14973332
- Weisenberger DJ, Campan M, Long TI, Kim M, Woods C, Fiala E, Ehrlich M, Laird PW. Analysis of repetitive element DNA methylation by MethyLight. Nucleic Acids Res 2005; 33:6823 - 36; http://dx.doi.org/10.1093/nar/gki987; PMID: 16326863
- Zhu ZZ, Hou L, Bollati V, Tarantini L, Marinelli B, Cantone L, Yang AS, Vokonas P, Lissowska J, Fustinoni S, et al. Predictors of global methylation levels in blood DNA of healthy subjects: a combined analysis. Int J Epidemiol 2012; 41:126 - 39; http://dx.doi.org/10.1093/ije/dyq154; PMID: 20846947
- Duong CV, Emes RD, Wessely F, Yacqub-Usman K, Clayton RN, Farrell WE. Quantitative, genome-wide analysis of the DNA methylome in sporadic pituitary adenomas. Endocr Relat Cancer 2012; 19:805 - 16; http://dx.doi.org/10.1530/ERC-12-0251; PMID: 23045325
- Fryer AA, Emes RD, Ismail KM, Haworth KE, Mein C, Carroll WD, Farrell WE. Quantitative, high-resolution epigenetic profiling of CpG loci identifies associations with cord blood plasma homocysteine and birth weight in humans. Epigenetics 2011; 6:86 - 94; http://dx.doi.org/10.4161/epi.6.1.13392; PMID: 20864804
- Byun HM, Siegmund KD, Pan F, Weisenberger DJ, Kanel G, Laird PW, Yang AS. Epigenetic profiling of somatic tissues from human autopsy specimens identifies tissue- and individual-specific DNA methylation patterns. Hum Mol Genet 2009; 18:4808 - 17; http://dx.doi.org/10.1093/hmg/ddp445; PMID: 19776032
- Takai D, Jones PA. Comprehensive analysis of CpG islands in human chromosomes 21 and 22. Proc Natl Acad Sci U S A 2002; 99:3740 - 5; http://dx.doi.org/10.1073/pnas.052410099; PMID: 11891299
- Huang W, Sherman BT, Lempicki RA. Systematic and integrative analysis of large gene lists using DAVID bioinformatics resources. Nat Protoc 2009; 4:44 - 57; http://dx.doi.org/10.1038/nprot.2008.211; PMID: 19131956
- Huang W, Sherman BT, Lempicki RA. Bioinformatics enrichment tools: paths toward the comprehensive functional analysis of large gene lists. Nucleic Acids Res 2009; 37:1 - 13; http://dx.doi.org/10.1093/nar/gkn923; PMID: 19033363
- Benjamini Y, Hochberg Y. Controlling the false discovery rate: a practical and powerful approach to multiple testing. J R Stat Soc, B 1995; 57:289 - 300
- Kanehisa M, Goto S. KEGG: kyoto encyclopedia of genes and genomes. Nucleic Acids Res 2000; 28:27 - 30; http://dx.doi.org/10.1093/nar/28.1.27; PMID: 10592173
- Kanehisa M, Goto S, Hattori M, Aoki-Kinoshita KF, Itoh M, Kawashima S, Katayama T, Araki M, Hirakawa M. From genomics to chemical genomics: new developments in KEGG. Nucleic Acids Res 2006; 34:Database issue D354 - 7; http://dx.doi.org/10.1093/nar/gkj102; PMID: 16381885
- Kanehisa M, Araki M, Goto S, Hattori M, Hirakawa M, Itoh M, Katayama T, Kawashima S, Okuda S, Tokimatsu T, et al. KEGG for linking genomes to life and the environment. Nucleic Acids Res 2008; 36:Database issue D480 - 4; http://dx.doi.org/10.1093/nar/gkm882; PMID: 18077471
- Mi H, Muruganujan A, Thomas PD. PANTHER in 2013: modeling the evolution of gene function, and other gene attributes, in the context of phylogenetic trees. Nucleic Acids Res 2013; 41:Database issue D377 - 86; http://dx.doi.org/10.1093/nar/gks1118; PMID: 23193289
- Rauch TA, Wu X, Zhong X, Riggs AD, Pfeifer GP. A human B cell methylome at 100-base pair resolution. Proc Natl Acad Sci U S A 2009; 106:671 - 8; http://dx.doi.org/10.1073/pnas.0812399106; PMID: 19139413
- Hughes T, Webb R, Fei Y, Wren JD, Sawalha AH. DNA methylome in human CD4+ T cells identifies transcriptionally repressive and non-repressive methylation peaks. Genes Immun 2010; 11:554 - 60; http://dx.doi.org/10.1038/gene.2010.24; PMID: 20463746
- Eckhardt F, Lewin J, Cortese R, Rakyan VK, Attwood J, Burger M, Burton J, Cox TV, Davies R, Down TA, et al. DNA methylation profiling of human chromosomes 6, 20 and 22. Nat Genet 2006; 38:1378 - 85; http://dx.doi.org/10.1038/ng1909; PMID: 17072317
- Illingworth R, Kerr A, Desousa D, Jørgensen H, Ellis P, Stalker J, Jackson D, Clee C, Plumb R, Rogers J, et al. A novel CpG island set identifies tissue-specific methylation at developmental gene loci. PLoS Biol 2008; 6:e22; http://dx.doi.org/10.1371/journal.pbio.0060022; PMID: 18232738
- Irizarry RA, Ladd-Acosta C, Wen B, Wu Z, Montano C, Onyango P, Cui H, Gabo K, Rongione M, Webster M, et al. The human colon cancer methylome shows similar hypo- and hypermethylation at conserved tissue-specific CpG island shores. Nat Genet 2009; 41:178 - 86; http://dx.doi.org/10.1038/ng.298; PMID: 19151715
- Liu J, Morgan M, Hutchison K, Calhoun VD. A study of the influence of sex on genome wide methylation. PLoS One 2010; 5:e10028; http://dx.doi.org/10.1371/journal.pone.0010028; PMID: 20386599
- Zhang FF, Cardarelli R, Carroll J, Fulda KG, Kaur M, Gonzalez K, Vishwanatha JK, Santella RM, Morabia A. Significant differences in global genomic DNA methylation by gender and race/ethnicity in peripheral blood. Epigenetics 2011; 6:623 - 9; http://dx.doi.org/10.4161/epi.6.5.15335; PMID: 21739720
- Breitling LP, Yang R, Korn B, Burwinkel B, Brenner H. Tobacco-smoking-related differential DNA methylation: 27K discovery and replication. Am J Hum Genet 2011; 88:450 - 7; http://dx.doi.org/10.1016/j.ajhg.2011.03.003; PMID: 21457905
- Zöchbauer-Müller S, Lam S, Toyooka S, Virmani AK, Toyooka KO, Seidl S, Minna JD, Gazdar AF. Aberrant methylation of multiple genes in the upper aerodigestive tract epithelium of heavy smokers. Int J Cancer 2003; 107:612 - 6; http://dx.doi.org/10.1002/ijc.11458; PMID: 14520700
- Houseman EA, Accomando WP, Koestler DC, Christensen BC, Marsit CJ, Nelson HH, Wiencke JK, Kelsey KT. DNA methylation arrays as surrogate measures of cell mixture distribution. BMC Bioinformatics 2012; 13:86; http://dx.doi.org/10.1186/1471-2105-13-86; PMID: 22568884
- Lyons PA, Koukoulaki M, Hatton A, Doggett K, Woffendin HB, Chaudhry AN, Smith KG. Microarray analysis of human leucocyte subsets: the advantages of positive selection and rapid purification. BMC Genomics 2007; 8:64; http://dx.doi.org/10.1186/1471-2164-8-64; PMID: 17338817
- Semple JW, Allen D, Chang W, Castaldi P, Freedman J. Rapid separation of CD4+ and CD19+ lymphocyte populations from human peripheral blood by a magnetic activated cell sorter (MACS). Cytometry 1993; 14:955 - 60; http://dx.doi.org/10.1002/cyto.990140816; PMID: 7507026
- Zahler S, Kowalski C, Brosig A, Kupatt C, Becker BF, Gerlach E. The function of neutrophils isolated by a magnetic antibody cell separation technique is not altered in comparison to a density gradient centrifugation method. J Immunol Methods 1997; 200:173 - 9; http://dx.doi.org/10.1016/S0022-1759(96)00206-2; PMID: 9005956
- Lea T, Smeland E, Funderud S, Vartdal F, Davies C, Beiske K, Ugelstad J. Characterization of human mononuclear cells after positive selection with immunomagnetic particles. Scand J Immunol 1986; 23:509 - 19; http://dx.doi.org/10.1111/j.1365-3083.1986.tb03083.x; PMID: 2422741
- Funderud S, Erikstein B, Asheim HC, Nustad K, Stokke T, Blomhoff HK, Holte H, Smeland EB. Functional properties of CD19+ B lymphocytes positively selected from buffy coats by immunomagnetic separation. Eur J Immunol 1990; 20:201 - 6; http://dx.doi.org/10.1002/eji.1830200129; PMID: 1689662
- Bibikova M, Barnes B, Tsan C, Ho V, Klotzle B, Le JM, Delano D, Zhang L, Schroth GP, Gunderson KL, et al. High density DNA methylation array with single CpG site resolution. Genomics 2011; 98:288 - 95; http://dx.doi.org/10.1016/j.ygeno.2011.07.007; PMID: 21839163
- Mill J, Yazdanpanah S, Gückel E, Ziegler S, Kaminsky Z, Petronis A. Whole genome amplification of sodium bisulfite-treated DNA allows the accurate estimate of methylated cytosine density in limited DNA resources. Biotechniques 2006; 41:603 - 7; http://dx.doi.org/10.2144/000112266; PMID: 17140118
- Don RH, Cox PT, Wainwright BJ, Baker K, Mattick JS. ‘Touchdown’ PCR to circumvent spurious priming during gene amplification. Nucleic Acids Res 1991; 19:4008; http://dx.doi.org/10.1093/nar/19.14.4008; PMID: 1861999
- Hecker KH, Roux KH. High and low annealing temperatures increase both specificity and yield in touchdown and stepdown PCR. Biotechniques 1996; 20:478 - 85; PMID: 8679209
- Haworth KE, Farrell WE, Emes RD, Ismail KM, Carroll WD, Borthwick HA, Yates AM, Hubball E, Rooney A, Khanam M, et al. Combined influence of gene-specific cord blood methylation and maternal smoking habit on birth weight. Epigenomics 2013; 5:37 - 49; http://dx.doi.org/10.2217/epi.12.72; PMID: 23414319
- Wessely F, Emes RD. Identification of DNA methylation biomarkers from Infinium arrays. Front Genet 2012; 3:161; http://dx.doi.org/10.3389/fgene.2012.00161; PMID: 22936948
- Dedeurwaerder S, Defrance M, Calonne E, Denis H, Sotiriou C, Fuks F. Evaluation of the Infinium Methylation 450K technology. Epigenomics 2011; 3:771 - 84; http://dx.doi.org/10.2217/epi.11.105; PMID: 22126295
- Sturn A, Quackenbush J, Trajanoski Z. Genesis: cluster analysis of microarray data. Bioinformatics 2002; 18:207 - 8; http://dx.doi.org/10.1093/bioinformatics/18.1.207; PMID: 11836235