Abstract
Epigenetic dysregulation contributes to the high cardiovascular disease burden in chronic kidney disease (CKD) patients. Although microRNAs (miRNAs) are central epigenetic regulators, which substantially affect the development and progression of cardiovascular disease (CVD), no data on miRNA dysregulation in CKD-associated CVD are available until now. We now performed high-throughput miRNA sequencing of peripheral blood mononuclear cells from ten clinically stable hemodialysis (HD) patients and ten healthy controls, which allowed us to identify 182 differentially expressed miRNAs (e.g., miR-21, miR-26b, miR-146b, miR-155). To test biological relevance, we aimed to connect miRNA dysregulation to differential gene expression. Genome-wide gene expression profiling by MACE (Massive Analysis of cDNA Ends) identified 80 genes to be differentially expressed between HD patients and controls, which could be linked to cardiovascular disease (e.g., KLF6, DUSP6, KLF4), to infection / immune disease (e.g., ZFP36, SOCS3, JUND), and to distinct proatherogenic pathways such as the Toll-like receptor signaling pathway (e.g., IL1B, MYD88, TICAM2), the MAPK signaling pathway (e.g., DUSP1, FOS, HSPA1A), and the chemokine signaling pathway (e.g., RHOA, PAK1, CXCL5). Formal interaction network analysis proved biological relevance of miRNA dysregulation, as 68 differentially expressed miRNAs could be connected to 47 reciprocally expressed target genes. Our study is the first comprehensive miRNA analysis in CKD that links dysregulated miRNA expression with differential expression of genes connected to inflammation and CVD. After recent animal data suggested that targeting miRNAs is beneficial in experimental CVD, our data may now spur further research in the field of CKD-associated human CVD.
Introduction
Patients with chronic kidney disease (CKD) face a tremendous burden of cardiovascular morbidity, which cannot adequately be lowered by traditional therapeutic strategies.Citation1 First evidence suggests that failure in epigenetic gene regulation may contribute to this high cardiovascular disease burden in patients with CKD.Citation2-Citation5
Among mechanisms of epigenetic regulation, miRNAs are centrally involved in the maintenance of cellular homeostasis and the control of inflammatory processes.Citation6,Citation7 miRNAs are small (~23 nt), endogenous and non-coding RNAs that regulate gene expression at the posttranscriptional level, either by degradation of target mRNAs or by inhibition of translation.
Importantly, one single miRNA can regulate a multitude of target genes and thus control specific pathways and signaling cascades such as inflammatory responses in endothelial cells and macrophages.Citation7,Citation8 Consequently, dysregulation of miRNA expression centrally contributes to the onset and development of diverse pathological conditions, including cardiovascular disease (CVD).Citation9,Citation10 In line, distinct miRNAs have been proposed as useful biomarkers for diagnosis and prognosis of CVD.Citation10-Citation15
Against this background, great expectations are placed in the development of synthetic anti-miRs as novel treatment strategies in cardiovascular medicine.Citation10,Citation16-Citation19 Such anti-miRs, which directly inhibit distinct miRNAs, have already been introduced as therapeutic agents in other disciplines of internal medicine.Citation20
However, before such therapeutic options can be transferred to patients suffering from cardiovascular disease in the context of CKD, more knowledge on regulation of inflammatory responses and atherogenesis by distinct miRNAs in uremia is needed.
Therefore, we aimed to characterize dysregulated miRNAs and associated signaling pathways in CKD, using high-throughput miRNA sequencing in combination with the recently introduced digital gene expression profiling technique MACE (Massive Analysis of cDNA Ends) as the latest advancement of tag-based gene expression analysis methods.
Results
Generation of MACE libraries
We performed genome-wide gene expression profiling of all HD patients (n = 10) and all control subjects (n = 10) by MACE in order to identify differentially expressed genes in HD patients. Therefore, RNA from PBMCs was used to generate 20 independent MACE libraries (10 “control libraries” and 10 “HD libraries”). In total 147 204 502 reads (= MACE tags) were generated across all libraries. After removal of duplicates, trimming of polyA-sequences and elimination of low-quality reads, of reads that were mapped to introns or intergenic regions, and of reads that could not be mapped at all, the total number of reads in coding regions was 51 167 601, comprising 21 308 090 reads from the control libraries and 29 859 511 reads from the HD libraries (Tables S1A and S1B). Across all libraries, these 51 167 601 reads accounted for 17 723 different genes (Table S2).
Differentially expressed genes in HD patients
Statistical data analysis (R) comprised hierarchical clustering of differentially expressed transcripts () as well as principal component analysis () and Pearson correlations () of all transcripts. We found HD patients and control subjects to cluster differentially; moreover, in line with their broad spectrum of comorbidity, HD patients displayed much higher heterogeneity in gene expression than control subjects.
Figure 1. Analysis of MACE results. (A) Unsupervised hierarchical cluster analysis with Euclidean distance measure of the 80 differentially expressed genes derived from MACE analysis. (B) Principle component analysis (PCA) of all expressed genes from the 20 examined samples. The first (x-axis) and second principal component (y-axis) accounted for 16% and 9%, respectively, of the total variation in the data. (C) Pearson product-moment correlation coefficient (PCC) for all samples compared within the control and patient group as well as between both groups.
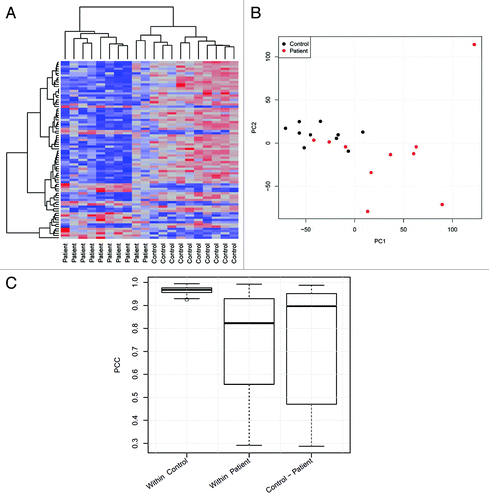
The DESeq R/Bioconductor package was used to estimate the biological variance between replicates and to test for differential expression. We identified 80 differentially expressed genes (P < 0.05) in HD patients compared with healthy controls, of which 60 were upregulated and 20 were downregulated. visualizes differences in gene expression between HD patients and control subjects, whereas and list the top 15 upregulated as well as the top 15 downregulated genes in HD patients (based on their FDR [False Discovery Rate] adjusted P value, according to Benjamini and HochbergCitation21).
Figure 2. Differences in gene expression between control subjects and hemodialysis patients. An MA plot was created to visualize the relation between log2 fold-change between controls and hemodialysis patients and log2 fold-average gene expression. Genes with a FDR (False Discovery Rate) adjusted P value < 0.05 are indicated in red.
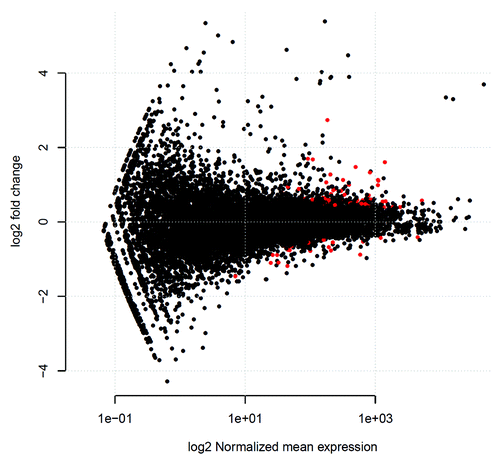
Table 1A. Top 15 upregulated genes in hemodialysis patients
Table 1B. Top 15 downregulated genes in hemodialysis patients
We next separately compared gene expression between HD patients with prevalent cardiovascular disease (CVD) and HD patients without prevalent CVD (Table S3). These subgroups differed in the expression of several genes. However, when applying the same strict statistical criteria as in the analysis of the total cohort, these differences lost statistical significance, given the small patient numbers in theses subgroups (n = 5).
Finally, we compared gene expression in healthy controls separately either to HD patients with CVD (Table S4), or to HD patients without prevalent CVD (Table S5). Differences in gene expression were more pronounced between healthy control subjects and HD patients with prevalent CVD (33 differentially expressed genes, Table S4), than between control subjects and HD patients without prevalent CVD (13 differentially expressed genes, Table S5).
Interaction network analyses
Next we generated interaction networks of gene products of differentially expressed genes in HD patients by using the String 9.0 software (http://string-db.org/; ). Thereby, differentially expressed genes could be annotated to distinct pathways, comprising the T cell receptor signaling pathway (PAK1, RHOA, FOS, CARD11, ZAP70), the MAPK signaling pathway (DUSP1, IL1B, FOS, JUND, HSPA1A, DUSP6, PAK1), the Toll-like receptor signaling pathway (FOS, IL1B, MYD88, TICAM2), the chemokine signaling pathway (RHOA, PAK1, CXCL5, GNG5), or the Jak-STAT signaling pathway (SOCS3, PIM1, IFNGR1, CSF2RB). Notably, several differentially expressed genes are coding for transcription regulators such as bzip transcription factors (FOSB, JUNB, FOS), zinc finger proteins (KLF4, PLAGL2, EGR1) or proteins belonging to the small GTPase superfamily, Rho type (RHOA, RHOB).
Gene ontology analysis for biological and functional differences
To confirm these associations, we performed gene ontology (GO) enrichment analyses in order to annotate differential gene expression in HD patients to biological and functional alterations of immune cells: within the GO term “biological process,” the most significant differences were found for the immune system process (P = 0.003) including the subcategories immune system development (e.g., JUNB, EGR1, KLF4), regulation of immune system process (e.g., ZFP36, FOS, LMO2), and immune response (e.g., DUSP6, SOCS3, EGR1). Within the GO term “molecular function,” the most significant differences were found for nucleic acid binding transcription factor activity (P = 0.005) with the subcategory sequence-specific DNA binding transcription factor activity (e.g., JUNB, FOSB, KLF4).
Dysregulated atherosclerosis-related genes in HD patients
We next analyzed whether those 80 genes differentially expressed between HD patients and controls could be linked to cardiovascular disease and—given the central role of inflammation in CKD-associated atherogenesis—to infection / immune disease. The Genetic Association Database (accessible from the National Institutes of Health; http://geneticassociationdb.nih.gov) linked 22 out of these 80 genes to cardiovascular disease (e.g., KLF6, DUSP6, VIM, CXCL5, KLF4), and 34 genes to infection / immune disease (e.g., ZFP36, SOCS3, FOS, JUND, ZAP70) (Table S6A and B).
Generation of miRNA expression libraries
In order to analyze whether these changes in gene expression among HD patients can be linked to differential miRNA expression, we performed ultra-deep miRNA sequencing with pooled small RNA from HD patients and controls. Thus, we generated two independent miRNA expression libraries (“control library” and “HD library”). After eliminating low-quality reads and tags which were detected only once, the total number of reads was 10 967 102, comprising 5 964 297 reads (109 834 unique sequences, “UniTags”) from the control library and 5 002 805 reads (89 794 UniTags) from the HD library. Applying the bioinformatics pipeline omiRas, UniTags from the control library were mapped to 287 342 genomic loci, whereas UniTags from the HD library were mapped to 244 072 loci. The distribution of mapping regions and the relative frequency of non-coding RNA (ncRNA) classes is depicted in Figure S1.
Differentially expressed miRNAs in HD patients
In total, 650 different miRNAs were identified in the combined libraries, 182 of which were differentially expressed (P < 0.001) between HD patients and control subjects (Table S7). Of these 182 differentially expressed miRNAs, 75 were upregulated in HD patients, whereas 107 were downregulated. The top 15 upregulated and the top 15 downregulated (both based on p-value) miRNAs in HD patients are presented in and . Notably, several of these highly differentially expressed miRNAs, have previously been linked with cardiovascular disease such as miR-21, miR-26b, miR-146b, or miR-155. Table S8 provides an overview of current study findings in the context of earlier data on miRNA expression in human cardiovascular and kidney disease.
Table 2A. Top 15 upregulated miRNAs in hemodialysis patients
Table 2B. Top 15 downregulated miRNAs in hemodialysis patients
Regulation of differentially expressed genes by miRNAs
Finally, we aimed to analyze whether CKD-specific miRNA dysregulation may explain differences in gene expression between HD patients and controls. Using the module for combined analysis of miRNA and mRNA data of omiRas, which integrates information of 8 miRNA-mRNA interaction databases, we found 155 interactions between 68 differentially expressed miRNAs and 47 differentially expressed target genes (Table S9). The interaction networks are presented in . Importantly, genes that were upregulated in HD patients could be linked to miRNAs of which the expression was downregulated (); in line, genes that were downregulated in HD patients could be linked to miRNAs with upregulated expression (). Moreover, among those genes differentially expressed between HD patients and controls, 13 out of 22 genes linked to cardiovascular disease, and 20 out of 34 genes linked to infection / immune disease by Genetic Association Database analysis, could be connected to dysregulated miRNA expression (Tables S7 and S9).
Discussion
Chronic kidney disease (CKD) patients suffer from a dramatically elevated cardiovascular event rate, which is mainly driven by accelerated arteriosclerosis. Traditional cardiovascular risk factors cannot fully explain this disease burden, and the implication of CKD specific risk factors is widely acknowledged.Citation1
WeCitation4,Citation5 and othersCitation2,Citation3 recently claimed that failure in epigenetic gene regulation centrally contributes to this high cardiovascular disease burden in CKD patients. However, earlier studies in this field were constrained to DNA methylation analysis, and the impact of miRNA dysregulation in CKD-associated atherosclerosis has not been analyzed until now, even though miRNAs are central regulators of virtually all processes in human (patho)physiology, including cardiovascular health and disease.Citation6-Citation15,Citation22
Against this background, we now aimed to identify dysregulated miRNAs in CKD by high-throughput sequencing and quantification of miRNAs. This allowed us to characterize 182 miRNAs differentially expressed between CKD patients and age- and gender-matched controls. Of note, this is the first report that applied next-generation technology for miRNA analysis in CKD. Compared with more conventional techniques such as qPCR and microarray platforms, next-generation sequencing has the advantage of not being biased by thermodynamics and thus allowing exact and digital quantification of miRNA expression.
To analyze the implications of miRNA dysregulation in the context of cardiovascular disease, we performed genome-wide gene expression analysis of all study participants by Massive Analysis of cDNA Ends (MACE). This allowed us to link miRNA dysregulation to differential gene expression, given that one single miRNA can simultaneously regulate a multitude of target genes and as one gene can be regulated by many different miRNAs.Citation23
MACE has recently been introduced as a digital gene expression method, in which each cDNA molecule is represented by one cDNA fragment (tag) of 94 bps, originating from a region 100–500 bps from the 3′ (poly-A) end of the transcript. High throughput sequencing of tags provides high resolution gene expression values and reveals differential expression of low-abundant transcripts beyond the scope of microarrays and RNaseq, if sequenced at similar depth.Citation24 Moreover, the applied TrueQuant method for elimination of PCR copies ensured correct quantification of the low-complexity sequences.Citation25
Thereby, analysis of 147 204 502 reads identified 80 genes differentially expressed in CKD patients. Interaction and gene ontology analyses allowed us to specifically identify dysregulation within pathways linked to immune system processes as well as to inflammation (e.g., the Toll-like receptor signaling pathway, the MAPK signaling pathway, and the Chemokine signaling pathway). Additionally, according to the Genetic Association Database, many of these differentially expressed genes could be linked to cardiovascular disease and also to infection/immune disease. Finally, interaction network analysis of dysregulated miRNAs and target transcripts connected 68 differential expressed miRNAs with 47 reciprocal expressed target genes thus pointing toward a central role of miRNA dysregulation in CKD-associated inflammation and/or arteriosclerosis.
Beyond the field of nephrology, a substantial contribution of miRNA dysregulation to cardiovascular disease has widely been acknowledged in recent years. Here, most studies focused on arteriosclerosis, as the single most important pathophysiological cause of cardiovascular disease in humans. Arteriosclerosis has been characterized as an inflammatory disease, which is mainly driven by monocytes and monocyte-derived macrophages.Citation26 Of note, miRNA networks centrally regulate the response of these cells during inflammation, and distinct miRNAs are differentially expressed in macrophages in response to certain inflammatory stimuli such as pro-inflammatory cytokines, TLR ligands, or oxLDL.Citation6-Citation8,Citation27-Citation33 Among these, miR-21, which is related to key processes in atherogenesis and which is highly overexpressed in human atherosclerotic plaques compared with non-atherosclerotic arteries,Citation22,Citation34 has been identified in the present study as one of the most upregulated miRNAs in CKD. Similar, in our CKD patients, we found upregulation of miR-34a, miR-146b, miR-26b, and miR-30e, all of which have been found to be overexpressed in atherosclerotic tissues from CAD patients in earlier studies.Citation22,Citation34,Citation35
Two earlier studies compared miRNA profiles of blood cells from patients with prevalent cardiovascular disease to healthy controls subjects (neither of which included patients with overt kidney disease). By real-time PCR profiling, Hoekstra and colleaguesCitation13 found an increased expression of miR-135a and a reduced expression of miR-147 in coronary artery disease (CAD) patients. A miRNA microarray approach by Taurino and colleaguesCitation14 identified upregulation of miR-140–3p and miR-182 among CAD patients. Notably, in the current analysis, we found upregulation of miR-182, but downregulation of miR-140–3p, among CKD patients.
Given their high stability, miRNAs can be detected and analyzed in any body fluid such as plasma or urine. Thus, circulating miRNA levels entered the field of emerging biomarkers in cardiovascular medicine, and a first epidemiologic study recently identified three miRNAs (miR-126, miR-223, and miR-197) as predictors of myocardial infarction.Citation36
In murine studies, Donners and colleaguesCitation37 recently demonstrated that hematopoietic deficiency of miR-155 enhances the development of atherosclerotic plaques in hyperlipidemic mice by limiting leukocyte recruitment to the atherosclerotic lesions. In this context, they found a skewed monocyte subset distribution with an increase in the Ly6C(hi) monocyte subset, which are considered the murine counterpart of human classical and intermediate monocytes. Interestingly, patients with CKD are characterized by profound shifts of monocyte subsets with high counts of intermediate monocytes (CD14++CD16+ monocytes),Citation38,Citation39 which are discussed as major cellular contributors to atherosclerotic disease.Citation40-Citation43 Notably, in our present study, miR-155 expression was strongly reduced in CKD patients, and further clinical studies will have to discern in how far distinct miRNA expression may contribute to monocyte subset biology in man.
Very few data on miRNA expression in CKD patients have been available before, which again mainly focused on circulating miRNAs. Neal and colleaguesCitation44 found levels of circulating miRNAs—among them the miR-155—to be strongly reduced in CKD patients. In patients with acute kidney injury, elevated plasma levels of miR-210 were characterized as independent predictor of mortality.Citation45 Of note, due to different underlying mechanisms of biogenesis, data on circulating miRNA should not uncritically be compared with cellular miRNA expression.Citation22 Moreover, although circulating miRNAs may undoubtedly serve as valuable biomarkers for distinct pathological conditions,Citation46 the characterization of cellular miRNA profiles may allow a more comprehensive understanding of epigenetic pathways in pathophysiological conditions such as atherosclerosis. Such understanding is further amplified by interaction network analysis, as done in the present manuscript, which provides first evidence that miRNA networks coordinate the expression of inflammatory related target genes in CKD. These network analyses underscore the notion that single miRNAs regulate multiple genes within specific signaling pathways, leading to a profound dysregulation of downstream gene networks when miRNA expression is disturbed.Citation23
In summary, we provide a first comprehensive miRNA analysis in CKD which allows to link dysregulated miRNA expression with differential expression of genes connected to inflammation and atherosclerosis. Since targeting of distinct miRNAs has been successfully applied in other fields of internal medicineCitation20 and since animal studies suggested similar therapy options against atherosclerotic vascular disease,Citation16,Citation18,Citation19 our data may hopefully spur further research on epigenetic dysregulation in CKD-associated cardiovascular disease.
Patients and Methods
Study participants
Ten clinically stable male CKD patients (56.1 ± 3.9 y) undergoing standard hemodialysis (HD) therapy thrice a week were recruited from the Department of Internal Medicine IV, Nephrology and Hypertension of the Saarland University Medical Center. From each patient, 20 ml EDTA (EDTA) anticoagulated blood was drawn before a dialysis session after the long-interdialytic interval. Five CKD patients had prevalent cardiovascular disease, defined as the presence of coronary artery disease (prior myocardial infarction or coronary revascularization), cerebrovascular disease (prior stroke or carotid revascularization), and / or peripheral artery disease (prior revascularization of lower-limb arteries). The remaining five CKD patients had no prevalent cardiovascular disease, which allowed post-hoc comparisons of gene expression between subgroups of CKD patients.
Ten healthy sex- and age-matched employees from Saarland University Medical Center served as controls. Mean age of controls was 53.5 ± 2.4 y, and their mean estimated glomerular filtration rate was 86.0 ± 14.3 ml/min/1.73 m2. No control subject had prevalent cardiovascular disease.
All participants gave written informed consent in accordance with the Declaration of Helsinki. The study protocol was approved by the local Ethics Committee.
RNA isolation and preparation of MACE (massive analysis of cDNA ends) and miRNA libraries
Peripheral blood mononuclear cells (PBMCs) were immediately isolated from anticoagulated blood by Ficoll-Paque (Lymphocyte Separation Medium; PAA) gradient density centrifugation. Total RNA was isolated from peripheral blood mononuclear cells using the NucleoSpin® miRNA kit (Macherey Nagel), which allows to separate the large and small fraction of the total RNA. The large fraction of the total RNA was used for the preparation of the MACE libraries, the small RNA fraction for miRNA library preparation.
For preparation of MACE libraries, poly-adenylated mRNA was isolated from 1 µg of the large fraction of total RNA using Dynabeads® mRNA Purification Kit (Life Technologies GmbH) and cDNA was produced by first and second strand synthesis using the SuperScript® III First-Strand Synthesis System (Life Technologies GmbH), but with modified, barcoded poly-T adapters that are suitable to bind on the Illumina Hiseq2000 flow cell and that are biotinylated at the very 5′ end. After cDNA production, the biotinylated cDNA was random-fragmented to reach an average size of 250 bps, the 3′ends were captured by streptavidin beads and GenXPro’s TrueQuant adapters were ligated. Ten barcoded samples were sequenced simultaneously in one lane of an Illumina Hiseq2000 with 1 × 100 bps.
For preparation of miRNA libraries, small RNA (17–30 bps) was size-selected from total RNA using the flashPAGE™ Small Nucleic Acid Purification System (Life Technologies). TrueQuant Adapters were ligated directly to the small RNA, basically as described by Hafner et al.Citation47 The miRNA population containing flanking p5 and p7 adapters was sequenced on a Hiseq2000 machine (Illumina). The MACE and miRNA library preparation was performed by GenXPro GmbH.
Data Analysis
Quantification of mRNA expression
MACE reads in each patient library were PolyA-trimmed and the marked quality region was removed. Mapping of reads to the human genome (hg19) was performed with novoalign (http://novocraft.com/). The mapping coordinates of each read were overlapped with the refseq annotation track from the UCSC table browser (http://genome.ucsc.edu/cgi-bin/hgTables?command=start) to quantify mature mRNA expression. Normalization and test for differential expression was performed in the statistical programming language R (www.r-project.org/) with the DEseq package.Citation48
GO-enrichment analysis was performed using the GO-enrichment toolkit from http://genxpro.ath.cx, which is based on the Fisher’s exact test among transcripts that are differentially expressed at a P value of 0.05.
Quantification of miRNA expression
Small RNA-Seq libraries were analyzed with omiRas (detailed workflow is presented at http://tools.genxpro.net/omiras).Citation49 For each small RNA-Seq library, data processing started with 3′ adaptor clipping by a local alignment of the adaptor sequence to each read. Subsequently, Illumina's marked quality region was removed and the reads were summarized to UniTags. Singletons were removed from the data set and the remaining tags were mapped to the human genome (hg19) with bowtie.Citation50 The mapped tags were annotated with the help of various models of coding and non-coding RNAs retrieved from the UCSC table browser. Tags mapping to exonic regions of coding genes were excluded from further analysis. Non-coding RNAs were quantified in each library. For tags mapping to multiple genomic loci the number of reads corresponding to the tag was divided by the number of mapping loci.
To account for differences in sequencing depth, tag-counts were normalized (NEV, normalized expression value) and differential expression was detected with the DEGseq bioconductor package.Citation51
Additional material
Download Zip (5.2 MB)Disclosure of Potential Conflicts of Interest
No potential conflicts of interest were disclosed.
Acknowledgments
This work was funded by a grant from the Else Kröner-Fresenius-Stiftung.
References
- Herzog CA, Asinger RW, Berger AK, Charytan DM, Díez J, Hart RG, Eckardt KU, Kasiske BL, McCullough PA, Passman RS, et al. Cardiovascular disease in chronic kidney disease. A clinical update from Kidney Disease: Improving Global Outcomes (KDIGO). Kidney Int 2011; 80:572 - 86; http://dx.doi.org/10.1038/ki.2011.223; PMID: 21750584
- Dwivedi RS, Herman JG, McCaffrey TA, Raj DS. Beyond genetics: epigenetic code in chronic kidney disease. Kidney Int 2011; 79:23 - 32; http://dx.doi.org/10.1038/ki.2010.335; PMID: 20881938
- Stenvinkel P, Ekström TJ. Epigenetics--a helpful tool to better understand processes in clinical nephrology?. Nephrol Dial Transplant 2008; 23:1493 - 6; http://dx.doi.org/10.1093/ndt/gfn056; PMID: 18308770
- Zawada AM, Rogacev KS, Heine GH. Clinical relevance of epigenetic dysregulation in chronic kidney disease-associated cardiovascular disease. Nephrol Dial Transplant 2013; 28:1663 - 71; http://dx.doi.org/10.1093/ndt/gft042; PMID: 23512108
- Zawada AM, Rogacev KS, Hummel B, Grün OS, Friedrich A, Rotter B, Winter P, Geisel J, Fliser D, Heine GH. SuperTAG methylation-specific digital karyotyping reveals uremia-induced epigenetic dysregulation of atherosclerosis-related genes. Circ Cardiovasc Genet 2012; 5:611 - 20; http://dx.doi.org/10.1161/CIRCGENETICS.112.963207; PMID: 23074332
- Liu G, Abraham E. MicroRNAs in immune response and macrophage polarization. Arterioscler Thromb Vasc Biol 2013; 33:170 - 7; http://dx.doi.org/10.1161/ATVBAHA.112.300068; PMID: 23325473
- Nazari-Jahantigh M, Wei Y, Schober A. The role of microRNAs in arterial remodelling. Thromb Haemost 2012; 107:611 - 8; http://dx.doi.org/10.1160/TH11-12-0826; PMID: 22371089
- Nazari-Jahantigh M, Wei Y, Noels H, Akhtar S, Zhou Z, Koenen RR, Heyll K, Gremse F, Kiessling F, Grommes J, et al. MicroRNA-155 promotes atherosclerosis by repressing Bcl6 in macrophages. J Clin Invest 2012; 122:4190 - 202; http://dx.doi.org/10.1172/JCI61716; PMID: 23041630
- Small EM, Olson EN. Pervasive roles of microRNAs in cardiovascular biology. Nature 2011; 469:336 - 42; http://dx.doi.org/10.1038/nature09783; PMID: 21248840
- Wei Y, Nazari-Jahantigh M, Neth P, Weber C, Schober A. MicroRNA-126, -145, and -155: a therapeutic triad in atherosclerosis?. Arterioscler Thromb Vasc Biol 2013; 33:449 - 54; http://dx.doi.org/10.1161/ATVBAHA.112.300279; PMID: 23324496
- Creemers EE, Tijsen AJ, Pinto YM. Circulating microRNAs: novel biomarkers and extracellular communicators in cardiovascular disease?. Circ Res 2012; 110:483 - 95; http://dx.doi.org/10.1161/CIRCRESAHA.111.247452; PMID: 22302755
- Fichtlscherer S, De Rosa S, Fox H, Schwietz T, Fischer A, Liebetrau C, Weber M, Hamm CW, Röxe T, Müller-Ardogan M, et al. Circulating microRNAs in patients with coronary artery disease. Circ Res 2010; 107:677 - 84; http://dx.doi.org/10.1161/CIRCRESAHA.109.215566; PMID: 20595655
- Hoekstra M, van der Lans CA, Halvorsen B, Gullestad L, Kuiper J, Aukrust P, van Berkel TJ, Biessen EA. The peripheral blood mononuclear cell microRNA signature of coronary artery disease. Biochem Biophys Res Commun 2010; 394:792 - 7; http://dx.doi.org/10.1016/j.bbrc.2010.03.075; PMID: 20230787
- Taurino C, Miller WH, McBride MW, McClure JD, Khanin R, Moreno MU, Dymott JA, Delles C, Dominiczak AF. Gene expression profiling in whole blood of patients with coronary artery disease. Clin Sci (Lond) 2010; 119:335 - 43; http://dx.doi.org/10.1042/CS20100043; PMID: 20528768
- van Empel VP, De Windt LJ, da Costa Martins PA. Circulating miRNAs: reflecting or affecting cardiovascular disease?. Curr Hypertens Rep 2012; 14:498 - 509; http://dx.doi.org/10.1007/s11906-012-0310-7; PMID: 22996205
- Lovren F, Pan Y, Quan A, Singh KK, Shukla PC, Gupta N, Steer BM, Ingram AJ, Gupta M, Al-Omran M, et al. MicroRNA-145 targeted therapy reduces atherosclerosis. Circulation 2012; 126:Suppl 1 S81 - 90; http://dx.doi.org/10.1161/CIRCULATIONAHA.111.084186; PMID: 22965997
- van Rooij E, Purcell AL, Levin AA. Developing microRNA therapeutics. Circ Res 2012; 110:496 - 507; http://dx.doi.org/10.1161/CIRCRESAHA.111.247916; PMID: 22302756
- Wei Y, Nazari-Jahantigh M, Chan L, Zhu M, Heyll K, Corbalán-Campos J, Hartmann P, Thiemann A, Weber C, Schober A. The microRNA-342-5p fosters inflammatory macrophage activation through an Akt1- and microRNA-155-dependent pathway during atherosclerosis. Circulation 2013; 127:1609 - 19; http://dx.doi.org/10.1161/CIRCULATIONAHA.112.000736; PMID: 23513069
- Rotllan N, Ramírez CM, Aryal B, Esau CC, Fernández-Hernando C. Therapeutic silencing of microRNA-33 inhibits the progression of atherosclerosis in Ldlr-/- mice--brief report. Arterioscler Thromb Vasc Biol 2013; 33:1973 - 7; http://dx.doi.org/10.1161/ATVBAHA.113.301732; PMID: 23702658
- Janssen HL, Reesink HW, Lawitz EJ, Zeuzem S, Rodriguez-Torres M, Patel K, van der Meer AJ, Patick AK, Chen A, Zhou Y, et al. Treatment of HCV infection by targeting microRNA. N Engl J Med 2013; 368:1685 - 94; http://dx.doi.org/10.1056/NEJMoa1209026; PMID: 23534542
- Hochberg Y, Benjamini Y. More powerful procedures for multiple significance testing. Stat Med 1990; 9:811 - 8; http://dx.doi.org/10.1002/sim.4780090710; PMID: 2218183
- Goettsch C, Hutcheson JD, Aikawa E. MicroRNA in cardiovascular calcification: focus on targets and extracellular vesicle delivery mechanisms. Circ Res 2013; 112:1073 - 84; http://dx.doi.org/10.1161/CIRCRESAHA.113.300937; PMID: 23538277
- Fernández-Hernando C, Ramírez CM, Goedeke L, Suárez Y. MicroRNAs in metabolic disease. Arterioscler Thromb Vasc Biol 2013; 33:178 - 85; http://dx.doi.org/10.1161/ATVBAHA.112.300144; PMID: 23325474
- Asmann YW, Klee EW, Thompson EA, Perez EA, Middha S, Oberg AL, Therneau TM, Smith DI, Poland GA, Wieben ED, et al. 3′ tag digital gene expression profiling of human brain and universal reference RNA using Illumina Genome Analyzer. BMC Genomics 2009; 10:531; http://dx.doi.org/10.1186/1471-2164-10-531; PMID: 19917133
- Lenz TL, Eizaguirre C, Rotter B, Kalbe M, Milinski M. Exploring local immunological adaptation of two stickleback ecotypes by experimental infection and transcriptome-wide digital gene expression analysis. Mol Ecol 2013; 22:774 - 86; http://dx.doi.org/10.1111/j.1365-294X.2012.05756.x; PMID: 22971109
- Ross R. Atherosclerosis--an inflammatory disease. N Engl J Med 1999; 340:115 - 26; http://dx.doi.org/10.1056/NEJM199901143400207; PMID: 9887164
- Ismail N, Wang Y, Dakhlallah D, Moldovan L, Agarwal K, Batte K, Shah P, Wisler J, Eubank TD, Tridandapani S, et al. Macrophage microvesicles induce macrophage differentiation and miR-223 transfer. Blood 2013; 121:984 - 95; http://dx.doi.org/10.1182/blood-2011-08-374793; PMID: 23144169
- Mayr M, Zampetaki A, Willeit P, Willeit J, Kiechl S. MicroRNAs within the continuum of postgenomics biomarker discovery. Arterioscler Thromb Vasc Biol 2013; 33:206 - 14; http://dx.doi.org/10.1161/ATVBAHA.112.300141; PMID: 23325478
- Zhuang G, Meng C, Guo X, Cheruku PS, Shi L, Xu H, Li H, Wang G, Evans AR, Safe S, et al. A novel regulator of macrophage activation: miR-223 in obesity-associated adipose tissue inflammation. Circulation 2012; 125:2892 - 903; http://dx.doi.org/10.1161/CIRCULATIONAHA.111.087817; PMID: 22580331
- O’Connell RM, Rao DS, Chaudhuri AA, Baltimore D. Physiological and pathological roles for microRNAs in the immune system. Nat Rev Immunol 2010; 10:111 - 22; http://dx.doi.org/10.1038/nri2708; PMID: 20098459
- O’Connell RM, Taganov KD, Boldin MP, Cheng G, Baltimore D. MicroRNA-155 is induced during the macrophage inflammatory response. Proc Natl Acad Sci U S A 2007; 104:1604 - 9; http://dx.doi.org/10.1073/pnas.0610731104; PMID: 17242365
- Sheedy FJ, Palsson-McDermott E, Hennessy EJ, Martin C, O’Leary JJ, Ruan Q, Johnson DS, Chen Y, O’Neill LA. Negative regulation of TLR4 via targeting of the proinflammatory tumor suppressor PDCD4 by the microRNA miR-21. Nat Immunol 2010; 11:141 - 7; http://dx.doi.org/10.1038/ni.1828; PMID: 19946272
- Taganov KD, Boldin MP, Chang KJ, Baltimore D. NF-kappaB-dependent induction of microRNA miR-146, an inhibitor targeted to signaling proteins of innate immune responses. Proc Natl Acad Sci U S A 2006; 103:12481 - 6; http://dx.doi.org/10.1073/pnas.0605298103; PMID: 16885212
- Raitoharju E, Lyytikäinen LP, Levula M, Oksala N, Mennander A, Tarkka M, Klopp N, Illig T, Kähönen M, Karhunen PJ, et al. miR-21, miR-210, miR-34a, and miR-146a/b are up-regulated in human atherosclerotic plaques in the Tampere Vascular Study. Atherosclerosis 2011; 219:211 - 7; http://dx.doi.org/10.1016/j.atherosclerosis.2011.07.020; PMID: 21820659
- Bidzhekov K, Gan L, Denecke B, Rostalsky A, Hristov M, Koeppel TA, Zernecke A, Weber C. microRNA expression signatures and parallels between monocyte subsets and atherosclerotic plaque in humans. Thromb Haemost 2012; 107:619 - 25; http://dx.doi.org/10.1160/TH11-09-0607; PMID: 22370758
- Zampetaki A, Willeit P, Tilling L, Drozdov I, Prokopi M, Renard JM, Mayr A, Weger S, Schett G, Shah A, et al. Prospective study on circulating MicroRNAs and risk of myocardial infarction. J Am Coll Cardiol 2012; 60:290 - 9; http://dx.doi.org/10.1016/j.jacc.2012.03.056; PMID: 22813605
- Donners MM, Wolfs IM, Stöger LJ, van der Vorst EP, Pöttgens CC, Heymans S, Schroen B, Gijbels MJ, de Winther MP. Hematopoietic miR155 deficiency enhances atherosclerosis and decreases plaque stability in hyperlipidemic mice. PLoS One 2012; 7:e35877; http://dx.doi.org/10.1371/journal.pone.0035877; PMID: 22558252
- Heine GH, Ulrich C, Seibert E, Seiler S, Marell J, Reichart B, Krause M, Schlitt A, Köhler H, Girndt M. CD14(++)CD16+ monocytes but not total monocyte numbers predict cardiovascular events in dialysis patients. Kidney Int 2008; 73:622 - 9; http://dx.doi.org/10.1038/sj.ki.5002744; PMID: 18160960
- Rogacev KS, Seiler S, Zawada AM, Reichart B, Herath E, Roth D, Ulrich C, Fliser D, Heine GH. CD14++CD16+ monocytes and cardiovascular outcome in patients with chronic kidney disease. Eur Heart J 2011; 32:84 - 92; http://dx.doi.org/10.1093/eurheartj/ehq371; PMID: 20943670
- Heine GH, Ortiz A, Massy ZA, Lindholm B, Wiecek A, Martínez-Castelao A, Covic A, Goldsmith D, Süleymanlar G, London GM, et al, European Renal and Cardiovascular Medicine (EURECA‑m) working group of the European Renal Association-European Dialysis and Transplant Association (ERA-EDTA). Monocyte subpopulations and cardiovascular risk in chronic kidney disease. Nat Rev Nephrol 2012; 8:362 - 9; http://dx.doi.org/10.1038/nrneph.2012.41; PMID: 22410492
- Rogacev KS, Cremers B, Zawada AM, Seiler S, Binder N, Ege P, Große-Dunker G, Heisel I, Hornof F, Jeken J, et al. CD14++CD16+ monocytes independently predict cardiovascular events: a cohort study of 951 patients referred for elective coronary angiography. J Am Coll Cardiol 2012; 60:1512 - 20; http://dx.doi.org/10.1016/j.jacc.2012.07.019; PMID: 22999728
- Zawada AM, Rogacev KS, Schirmer SH, Sester M, Böhm M, Fliser D, Heine GH. Monocyte heterogeneity in human cardiovascular disease. Immunobiology 2012; 217:1273 - 84; http://dx.doi.org/10.1016/j.imbio.2012.07.001; PMID: 22898391
- Zawada AM, Rogacev KS, Rotter B, Winter P, Marell RR, Fliser D, Heine GH. SuperSAGE evidence for CD14++CD16+ monocytes as a third monocyte subset. Blood 2011; 118:e50 - 61; http://dx.doi.org/10.1182/blood-2011-01-326827; PMID: 21803849
- Neal CS, Michael MZ, Pimlott LK, Yong TY, Li JY, Gleadle JM. Circulating microRNA expression is reduced in chronic kidney disease. Nephrol Dial Transplant 2011; 26:3794 - 802; http://dx.doi.org/10.1093/ndt/gfr485; PMID: 21891774
- Lorenzen JM, Kielstein JT, Hafer C, Gupta SK, Kümpers P, Faulhaber-Walter R, Haller H, Fliser D, Thum T. Circulating miR-210 predicts survival in critically ill patients with acute kidney injury. Clin J Am Soc Nephrol 2011; 6:1540 - 6; http://dx.doi.org/10.2215/CJN.00430111; PMID: 21700819
- Khella HW, Bakhet M, Lichner Z, Romaschin AD, Jewett MA, Yousef GM. MicroRNAs in kidney disease: an emerging understanding. Am J Kidney Dis 2013; 61:798 - 808; http://dx.doi.org/10.1053/j.ajkd.2012.09.018; PMID: 23219107
- Hafner M, Landgraf P, Ludwig J, Rice A, Ojo T, Lin C, Holoch D, Lim C, Tuschl T. Identification of microRNAs and other small regulatory RNAs using cDNA library sequencing. Methods 2008; 44:3 - 12; http://dx.doi.org/10.1016/j.ymeth.2007.09.009; PMID: 18158127
- Anders S, Huber W. Differential expression analysis for sequence count data. Genome Biol 2010; 11:R106; http://dx.doi.org/10.1186/gb-2010-11-10-r106; PMID: 20979621
- Müller S, Rycak L, Winter P, Kahl G, Koch I, Rotter B. omiRas: a Web server for differential expression analysis of miRNAs derived from small RNA-Seq data. Bioinformatics 2013; 29:2651 - 2; http://dx.doi.org/10.1093/bioinformatics/btt457; PMID: 23946503
- Li H, Durbin R. Fast and accurate short read alignment with Burrows-Wheeler transform. Bioinformatics 2009; 25:1754 - 60; http://dx.doi.org/10.1093/bioinformatics/btp324; PMID: 19451168
- Wang L, Feng Z, Wang X, Wang X, Zhang X. DEGseq: an R package for identifying differentially expressed genes from RNA-seq data. Bioinformatics 2010; 26:136 - 8; http://dx.doi.org/10.1093/bioinformatics/btp612; PMID: 19855105