Abstract
Epigenetic modifications introduce an additional layer of regulation that drastically expands the instructional capability of the human genome. The regulatory consequences of DNA methylation is context dependent; it can induce, enhance, and suppress gene expression, or have no effect on gene regulation. Therefore, it is essential to account for the genomic location of its occurrence and the protein factors it associates with to improve our understanding of its function and effects. Here, we use ENCODE ChIP-seq and DNase I hypersensitivity data, along with large-scale breast cancer genomic data from The Cancer Genome Atlas (TCGA) to computationally dissect the intricacies of DNA methylation in regulation of cancer transcriptomes. In particular, we identified a relationship between estrogen receptor α (ERα) activity and DNA methylation patterning in breast cancer. We found compelling evidence that methylation status of DNA sequences at ERα binding sites is tightly coupled with ERα activity. Furthermore, we predicted several transcription factors including FOXA1, GATA1, and SUZ12 to be associated with breast cancer by examining the methylation status of their binding sites in breast cancer. Lastly, we determine that methylated CpGs highly correlated with gene expression are enriched in regions 1kb or more downstream of TSSs, suggesting more significant regulatory roles for CpGs distal to gene TSSs. Our study provides novel insights into the role of ERα in breast cancers.
Introduction
DNA methylation is a biochemical process that modifies the cytosine nucleotides in the context of CpG dinucleotides (CpGs) by the addition of a methyl group to the fifth carbon position. DNA methylation plays critical roles in many important biological processes including genomic imprinting,Citation1 X-chromosome inactivation,Citation2 transposable elements silencing,Citation3 stem cell differentiation,Citation4 embryonic development,Citation5 and inflammation.Citation6 In humans, DNA methylation patterns are precisely regulated to maintain a delicate balance between stability and plasticity. Alterations in DNA methylation have been demonstrated to interact with genetic events and to be involved in human carcinogenesis for nearly all cancer types.Citation7,Citation8 A global shift in DNA hypomethylation in cancer cells has been reported, which is implicated in the development and progression of cancer.Citation9,Citation10 More specifically, genome wide DNA methylation profiling has been performed in breast cancer to identify genes associated with tumorigenesis.Citation11-Citation16 DNA methylation signatures or markers have been defined to classify breast cancer subtypesCitation17-Citation19 and to predict prognostic outcomes, e.g., patient survival.Citation10,Citation20
DNA methylation may affect gene expression by directly impacting the binding of transcription factors (TFs).Citation21 It has been suspected that DNA methylation physically impedes the binding of transcription factors to their binding sites.Citation22-Citation24 While this might be the case for most transcription factors, exceptions have been encountered in several studies. For example, Holler et al. showed that Sp1 is capable of binding DNA and activating transcription even when the binding site is methylated.Citation25 In addition, Guillaume et al. showed that a family of zinc finger proteins can bind methylated DNA and repress gene transcription.Citation26
Alternatively, DNA methylation may also regulate transcription by modifying local chromatin structure; however, the exact mechanisms by which this occurs are unclear.Citation27 There is convincing evidence demonstrating a linkage between DNA methylation and chromatin structure mediated by methylcytosine-binding proteins (MBPs).Citation28 A subset of these MBPs contain conserved methylcytosine-binding domains (MBD) that recognize and bind methylated cytosines and recruit additional chromatin remodeling factors such as histone deacetylases and histone methylases, leading to compacted inactive local chromatin structure. Another set of proteins that have been shown to function as methylcytosine-binding proteins contain SET- and Ring-associated (SRA) domains such as UHRF1.Citation29,Citation30 Furthermore, Kaiso-like zinc finger motifs have been shown to bind single methylated CpGs.Citation31 The large variety of protein motifs capable of binding methylated DNA is indicative of the complex interplay involving protein factors that couple DNA methylation to chromatin structure.
Although both of the above two mechanisms imply a repressive effect of DNA methylation on gene transcription, studies have shown a more complicated relationship between DNA methylation and gene expression.Citation32 Generally, methylation in the immediate vicinity of the TSS blocks initiation, but methylation in the gene body does not block and might even stimulate transcription elongation.Citation32 Thurman et al. examined the correlation between methylation levels at transcription factor binding sites (TFBS) and transcription factor abundance within DNase I hypersensitive (DHS) sites. They observed that 70% of transcription factors were negatively correlated with DNA methylation, whereas only a few transcription factors exhibited significant positive correlations. In general, CpG methylation within transcription factor binding sites is negatively correlated with the expression level of the corresponding transcription factor. Furthermore, they argue that a negative correlation between CpG methylation and transcription factor gene expression indicates that DNA methylation is a passive process, i.e., methylation fills in the voids left by vacating transcription factors.Citation33
In breast cancer, estrogen receptor (ESR1) activity status is a critical biomarker for subtype classification and is widely used to determine whether or not a patient should receive hormone therapy such as Tamoxifen treatment.Citation34 Consequently, DNA methylation predictors have been proposed as a clinical marker for ESR1 activity.Citation34 Differential methylation of ESR1 in breast carcinomas was first described by Piva et al. using the methylation–sensitive endonuclease HpaII.Citation35 More recently, several studies have applied high-throughput technologies including deep sequencing and microarrays to study DNA methylation at a genome-wide level. Li et al. identified 5 genes that were significantly differentially methylated between 12 ER+ and 12 ER- breast tumors using the Infinium Methylation Assay.Citation36 Similarly, Fackler et al. interrogated 27 578 CpG loci to deduce which genes were most associated with ER status in 103 breast tumor samples.Citation14 Another approach used MethyLight to measure the methylation levels of 35 gene markers to classify 148 primary breast carcinomas.Citation37 All of these studies performed 2-dimensional unsupervised hierarchical cluster analysis to identify the most differentially methylated CpGs between ER+ and ER- breast cancer.Citation14,Citation36,Citation37 To understand the spatial distribution of aberrant CpG methylation, Ruike et al. used methyl-DNA immunoprecipitation followed by high-throughput sequencing to identify genomic regions in breast cancer cell lines that exhibit hyper- or hypomethylation.Citation38 However, these studies did not investigate the association of DNA methylation with breast cancer by interrogating >485 000 CpG sites at the level of specific transcription factor binding using methylation and gene expression data from 222 TCGA-derived breast tumor samples.Citation11
In this report, we conducted a detailed study of the relationship between the binding of a sequence-specific transcription factor and the methylation level at its corresponding binding sites, using the well-studied estrogen receptor in breast cancer as a model system. Our study revealed that methylation level of ESR1 binding sites is negatively correlated with ESR1 expression levels, and ESR1 binding sites tend to be methylated in ER- breast cancers. In addition, our results indicate that ESR1 exerts its effect on DNA methylation within its binding regions in a localized fashion. Based on this conjecture, we further predicted FOXA1 and GATA3 to be overactive in ER+ breast cancers. In addition, we determined CTBP2 and PRC2 family member SUZ12 to be positively associated with DNA methylation. Finally, we found that CpGs in DNase I hypersensitive regions are more likely to be negatively correlated with expression of corresponding genes, which is consistent with the findings that most transcription factors are trans-activating. This analysis bridges a comprehensive and high-resolution portrait of the breast cancer DNA methylome to the regulatory processes responsible for breast cancer classification. Specifically, by integrating ENCODE and TCGA data sets, we link DNA methylation to transcription factor binding to chromatin state; all of which are integral in determining a final gene expression output.
Results
Correlation of DNA methylation with ESR1 expression
An overview of our analysis strategy is provided in . We focused on determining whether or not genomic features of CpG sites (those which are bound by ER α or other TFs, or located in DNase I hypersensitive sites) impact their methylation levels and their correlation with gene expression. To achieve high-resolution, this analysis was conducted by considering CpGs specifically located in TF binding sites and in DHS regions. In our first analysis, we operate under the assumption that ESR1 (gene that encodes ERα) expression is a proxy for ERα activity and correlated the DNA methylation level of all CpGs with ESR1 expression levels across all TCGA breast cancer samples stratified on ER status (see for an example). On average, the Spearman correlation coefficient (SCC) between overall CpG methylation (across the whole genome) and ESR1 expression is -0.056. As part of the ENCODE blueprint, ChIP-seq data was generated for >100 TFs in various cell lines incubated under different treatments.Citation39 Using TCGA and ENCODE data sets, we defined a CpG set consisting of CpGs located in genomic regions not bound by ERα and determined that the average correlation between non-ERα binding CpGs and ESR1 expression was –0.083. Conversely, we correlated methylation of CpGs in genomic regions bound by ERα with ESR1 expression and obtained a striking correlation coefficient as extreme as –0.20 (). Among CpGs not in ERα binding regions, 5.8% yield r > 0.4 and 2.8% yield r < –0.4 in their correlation with ESR1 expression (). Contrastingly, in the case of CpGs in ERα binding regions, 0.55% yield r > 0.4 and 24% yield r < –0.4 (, all SCCs are based on 222 samples; a correlation coefficient of r > 0.4 or r < –0.4 corresponds to a p-value of P < 3e-10). This suggests a strong enrichment of methylated CpGs in ERα binding sites that are negatively correlated with ESR1 expression. Moreover, for each CpG in an ERα binding region we calculated and compared its average DNA methylation level between ER+ and ER- breast cancer samples. As expected, the majority of CpGs in ERα binding regions exhibit lower average methylation levels in ER+ than in ER- samples ().
Figure 1. The schematic diagram of our analysis. We combined DNA methylation and gene expression data in breast cancer samples from TCGA, and TF binding and DNase I hypersensitivity data from ENCODE. We identified the CpG sites with differential methylation levels between ER+ and ER- breast cancer samples, and examined the correlation of their methylation levels with expression of associated genes. Blue double arrows denote comparative analysis of regions of interest to outside regions.
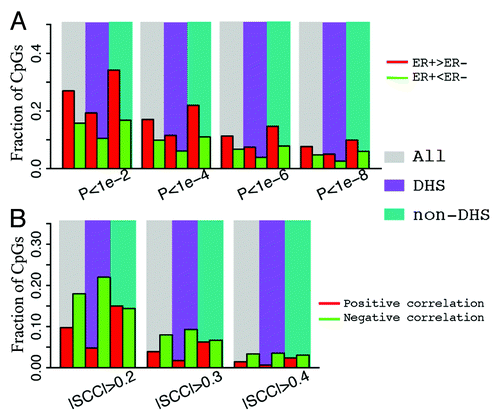
Figure 2. Correlation between CpG methylation and ESR1 expression levels. (A) An example- correlation between methylation level of cg03387103 (corresponding to LETM1) and ESR1 expression level in all breast cancer samples. (B) CpGs in ER binding peaks have larger negative correlations with ESR1 expression in their methylation levels. (C) Fraction of CpGs highly correlated with ESR1 expression. There is a higher fraction of anti-correlated CpGs in ERα binding sites compared with non-ERα binding sites at ± 0.4 SCC cutoff. (D) CpGs in ER binding peaks have higher average methylation levels in ER- than ER+ samples. Each point is a CpG. SCC: Spearman correlation coefficient.
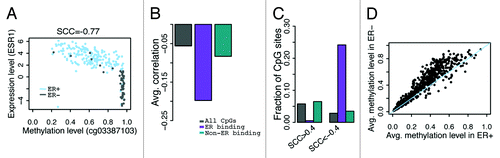
Distribution of differential DNA methylation between ER+ and ER
The DNA methylation levels of many CpGs in breast cancer samples are dependent on ER status. Some CpGs demonstrate higher methylation levels in ER+ than in ER- samples (), while others show the opposite trend (). We systematically investigated the distribution of CpGs with significant differential methylation levels between ER+ and ER- samples. Specifically, we calculated the position of each significant CpG relative to the transcription start site (TSS) of the gene it is associated with (). As shown in , the distribution of significant CpGs is centered at the TSS of genes, suggesting that there is a greater number proximal to gene TSS. However, this provides no indication as to how probable any CpG selected at random from all CpGs will be significantly differentially methylated because there is an inherent enrichment of significant and non-significant CpGs vicinal to gene TSS. Therefore, by calculating the fraction of significant CpGs to the total number of CpGs at each genomic coordinate we account for the non-uniformity of CpGs distributed across genes. Consequently, we observe that CpGs nearby TSS have a lower likelihood (relative frequency) of exhibiting significant differential methylation than those in distal DNA regions (). Overall, this result suggests that CpGs at locations distal from TSS might be equally or even more functionally relevant. Moreover, we observed that, at the same significance level, the fraction of hypermethylated CpGs (, red) is higher than that of hypomethylated CpGs in ER+ samples (hypermethylated CpGs in ER- samples.) (, green).
Figure 3. Distribution of CpGs with differential methylation levels between ER+ and ER- breast samples. (A) Examples- CpG may have higher methylation in ER+ (cg05846044) or ER- (cg05859267). (B) Relationship between differential methylation and CpG position relative to transcription start site (from –1500 upstream to 4500 downstream of TSS). (C) Distribution of CpGs with significant (P < 1e-6) differential methylation between ER+ and ER- samples. (D) Fraction of CpGs with significant differential methylation levels at different positions. The fraction is the ratio of the number of significant CpGs to the total number of CpGs in a DNA window. CpGs with significantly higher methylation levels in ER+ (red) and in ER- (green) samples are examined separately. The relative frequency of significantly differentially methylated CpGs increases as the distance from TSS increases.
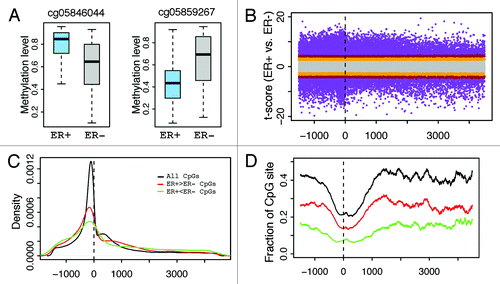
Impact of ERα binding on DNA methylation
We next investigated the relationship between DNA methylation and transcription factor (TF) binding based on ENCODE ChIP-seq data. First, we considered the question: Are CpGs in ERα binding regions more likely to be differentially methylated between ER+ and ER- breast cancer samples? Based on the ERα binding peaks in T47d cell line, we defined an ERα-binding region CpG set and a non-ERα-binding CpG set as a control. The former consists of CpGs that fall precisely within an ERα binding peak. The latter contains CpGs that do not fall directly within ERα binding peaks, but do fall in gene regions that contain these binding peaks. Overall, CpGs included in the analysis are selected from regions within the genes that are bound by ERα (i.e., has a binding peak in their gene body or promoter).
We find that CpGs that are localized within ERα binding peaks exhibit lower DNA methylation in ER+ than in ER- samples (), suggesting a negative correlation between ERα binding and site-specific DNA methylation. For example, if ER+ samples are analyzed at a significance level α = 1e-6, 31% of CpGs in ERα binding regions exhibit lower methylation levels whereas only 1.1% exhibit higher methylation levels (Table S1). In contrast, if CpGs from all genomic locations are considered, only 11.3% of CpGs have higher methylation levels and 6.7% of CpGs have lower methylation levels in ER+ compared with ER- samples. Likewise, similar fractions were observed with non-ERα binding CpGs. This trend remains stable when different significance thresholds are used ().
Figure 4. CpGs in ER binding sites tend to have lower methylation levels in ER+ breast samples. (A) The fraction of CpGs with significant differential methylation levels between ER+ and ER- samples. Note that CpGs in ER binding regions tend to have higher methylation levels in ER- samples, while CpGs not in ER binding regions tend to have higher methylation levels in ER+ samples. Four different thresholds are used to determine differential methylated CpGs. (B) Distribution of t-scores (ER+ vs. ER-) of methylation levels for CpGs. Genes are divided into 3 classes based on their expression levels in ER+ vs. ER- samples: ER+ > ER- (red), ER+ < ER- (green), and ER+ = ER- (white). Distributions of CpGs associated with the three gene classes are shown separately. (C) CpGs in ER binding regions tend to have lower methylation levels in ER+ samples (lower t-scores) compared with thosenot in ER binding regions, which is the case for CpGs associated with all three gene classes.
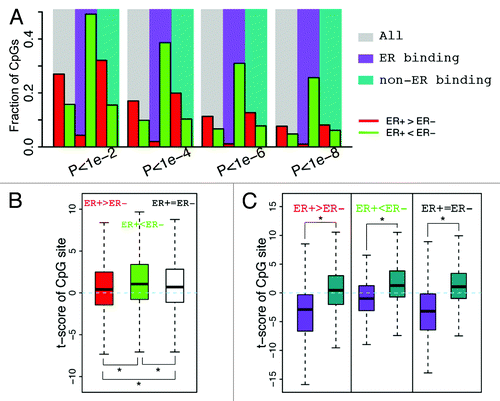
Because promoter DNA methylation is generally negatively correlated with gene expression status, we compared expression levels of genes between ER+ and ER- samples. First, we defined 3 gene categories: upregulated, downregulated, and non-differentially expressed genes in ER+ vs. ER- samples. To quantify the difference in methylation levels between ER+ and ER- samples, we calculated the t-scores of β values for each gene category (ER+ vs. ER-). As shown in , CpGs in ER+ upregulated genes tend to have lower t-scores (i.e., ER+ is hypomethylated) as compared with CpGs in ER+ downregulated genes. In spite of this trend, the ERα binding CpGs demonstrated significantly lower methylation t-scores than non-ERα binding CpGs in all three of the gene categories ().
To further investigate the impact of ERα binding on DNA methylation, we calculated the CpG methylation levels as a function of its distance to the center of an ERα binding peak (). Strikingly, we find that CpGs closer to the center are more likely to have larger negative t-scores, namely, are more likely to have lower DNA methylation levels in ER+ than in ER- samples (). Consistent with the function of SUZ12, CpGs closer to the center of SUZ12 binding peaks are more likely to have larger positive t-scores ().
Figure 5. Relationship between differential methylation of CpGs and TF binding. (A) Binding of some TFs is correlated with reduced methylation level of CpGs in ER+ relative to ER- samples, while binding of others (SUZ12 and CTBP2) is correlated with increased methylation level. (B) CpGs proximal to ER binding center are more likely to have lower methylation levels in ER+ (smaller t-scores for ER+ vs. ER- comparison). (C) CpGs proximal to SUZ12 binding center are more likely to have higher methylation levels in ER+ (larger t-scores for ER+ vs. ER- comparison).
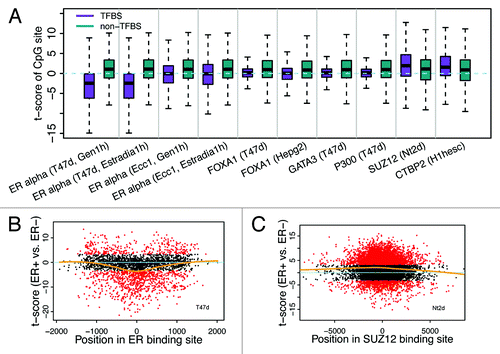
Taken together, our results indicate that the impact of ERα binding on DNA methylation is restricted to a local genomic region. The methylation level of ERα binding region CpGs is determined mainly by the ER status of samples (ER+ or ER-) rather than by the transcriptional status of genes (upregulated or downregulated in ER+).
Impact of other TF binding on DNA methylation
We next extended the analysis to other TF binding sites by defining a TFBS CpG set and a non-TFBS CpG set for all TF binding data from ENCODE, and compared methylation levels of TFBS CpG sets between ER+ and ER- samples. Although ERα seems to be the TF with the most significant impact on differential DNA methylation between ER+ and ER- samples, there are some other TFs that also exhibit influence (). For instance, the CpGs located in FOXA1 and GATA3 TF binding sites are significantly hypomethylated in ER+ than in ER- as compared with the corresponding non-TFBS control CpGs. Consistently, these two TFs have been reported to function upstream of ER and mediate ER binding in breast cancer.Citation40,Citation41
From the ENCODE data, four data sets containing ERα binding peaks were generated, which includes ERα binding analysis performed under treatment with two different steroid hormones (Gen1h and Estradia1h) in two cell lines responsive to primary steroid hormone treatment (T47d and Ecc1). Interestingly, the methylation difference (t-scores in ER+ vs. ER-) between ERα binding CpGs and non-ERα binding CpGs is much more obvious for peaks identified in T47d than those identified in Ecc1 (). Given the fact that T47d is a breast epithelial-derived and Ecc1 is an endometrium epithelial-derived cell line, this result, as expected, likely indicates that T47d better reflects the ERα binding events in human breast cancer tissue than Ecc1.
Moreover, we also identified a number of TFs whereby the TFBS CpGs had larger t-scores than the non-TFBS CpGs, implying that binding of these TFs would enhance DNA methylation. One of these TFs is SUZ12, a component of the polycomb repressive complex 2 (PRC2), which catalyzes methylation of H3K7.Citation42 Considering the chromatin-silencing role of PRC2,Citation43 it may not be surprising to observe the enhanced DNA methylation in the SUZ12 binding CpGs. Another example is CtBP2, which also show higher DNA methylation in its binding sites. Interestingly, CTBP2 has been reported to function as a transcriptional repressor.Citation44
Correlation between DNA methylation and gene expression
Depending on the genomic position and other factors, methylation of a CpG can be positively () or negatively () correlated with the expression levels of its associated genes. Therefore, for each CpG with a unique gene assignment, we calculated the Spearman correlation coefficient between its methylation level and the gene expression levels. shows the relationship between correlation and relative position (the distance from CpG to the TSS of its associated gene) of CpGs. As shown, there are significantly more instances of negative correlation than positive correlation and a large number of negative correlations occur at the DNA region proximal to the TSS. More clearly, the distributions of CpGs with r > 0.4 (the red line) or r < –0.4 (the green line) are shown in . As shown, most of the CpGs negatively correlated with expression are located in a DNA region upstream of TSS, whereas the CpGs with positive correlations exhibit two peaks, one in the gene body and the other in the promoter region. However, after taking into account the biased distribution of CpGs interrogated by the Illumina 450k DNA methylation array (the black line in ), the fraction of high correlation CpGs (r > 0.4 or r < –0.4) is maximal in the DNA region more than 1kb downstream of TSS () rather than in the TSS region.
Figure 6. Correlation of CpG methylation level with expression level of the associated genes. (A) Methylation level of cg06228260 is positively correlated with its associated gene PTPRN2. (B) Methylation level of cg01586506 is negatively correlated with its associated gene SOX10. (C) Relationship between methylation-expression correlation and CpG position relative to transcription start site (from –1500 upstream to 4500 downstream of TSS). (D) Distribution of CpGs with strong correlations in methylation with expression level of the associated genes. Positive correlation (red, r > 0.4) and negative correlation (green, r < –0.4) are examined separately. (E) Fraction of CpGs strongly correlated with expression of the associated genes at different positions. (F) CpGs in ER binding regions tend to have negative correlation in their methylation with expression of their associated genes.
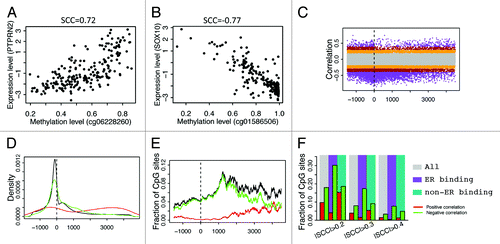
In parallel to what we have done in differential DNA methylation analysis, we compared the correlations with gene expression levels between ERα binding region CpGs and non-ERα binding region CpGs. The results indicate that CpGs in ERα binding peaks are more likely to be negatively correlated with gene expression levels (). Overall, 1.4% and 3.3% of all CpGs have positive (>0.4) and negative (<–0.4) correlations, respectively. Similarly, the fraction of high correlations is just slightly higher than the overall fraction in the non-ERα binding CpGs. However, 0.4% of ERα binding CpGs are positively correlated with gene expression and 7.7% are negatively correlated when the same significance threshold is maintained. This implies that CpGs specifically in ERα binding regions are more likely to exert their influence on gene expression regulation in breast cancers.
CpGs in DNase hypersensitive sites
TF binding data from ChIP-seq capture the binding events of a single TF in each experiment. The DNase I hypersensitivity data, however, identify the DNA regions enriched for all DNA regulatory elements.Citation33 Based on the DNase hypersensitivity data in T47d, we defined a DHS CpG set and a non-DHS CpG set. First we compared the fraction of differentially methylated CpGs (ER+ vs. ER-) in these two sets. Interestingly, we found that differentially methylated CpGs are depleted in DHS (). This observation is consistent with the fact that DHS is enriched for all types of regulatory elements, among which only a small fraction (e.g., ER binding sites, FOXA1 binding sites) are correlated with ER status. Most regulatory elements should show similar activities between ER+ and ER- samples. Consequently, these elements should have similar DNA methylation states in ER+ and ER- samples.
Figure 7. Comparison of CpGs in and not in DNase hypersensitive sites. (A) CpGs in DHS and non-DHS exhibit no significant difference in differential methylation between ER+ and ER- breast cancer samples. (B) CpGs in DHS tend to have negative correlation in their methylation level with the expression of their associated genes.
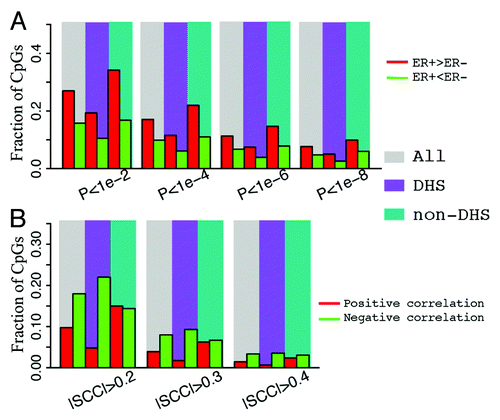
We also compared DHS CpGs and non-DHS CpGs in the correlation of their methylation levels with gene expression. As shown in , CpGs with high negative correlations are enriched in DHS sites, whereas CpGs with high positive correlations are depleted in DHS sites. This suggests that CpGs involved in gene expression regulation is enriched in DHS regions. Most of such regulation might be mediated by the binding of positive regulators, e.g., transcription activators, which leads to reduced DNA methylation and thus a negative correlation with gene expression.
Discussion
In this study, we investigated the relationship of TF binding regions with DNA methylation using ERα binding activity as a model. We found that CpGs in ERα binding peaks were more likely to be hypomethylated in ER+ than in ER- breast cancer samples. Furthermore, methylation of these CpGs had a greater likelihood of being negatively correlated with gene expression. These results indicate that CpG methylation in distinct ERα binding sites may be dependent on ERα activity and that physical binding of ERα to its cognate DNA sequence has the potential to inhibit methylation of these CpGs. Moreover, we showed that such an effect was restricted to a local DNA region proximal to the center of ERα binding peaks (). Lastly, by increasing the resolution of our analysis by considering CpGs in DHS regions, we observed that these regions harbor a large fraction of CpGs negatively correlated with gene expression. This result suggests that these CpGs have a higher probability of being functionally relevant since DHS regions are more accessible to protein regulators. Overall, this suggests a model whereby TF binding events impact the methylation status of local CpGs, and the final effect of DNA methylation on gene expression is determined by the overall output of each neighboring CpG’s methylation status. Additionally, the methylation status of CpGs might be determined by the binding of many different TFs cooperatively or competitively. Instead of acting as the readout of gene expression, DNA methylation may participate in transcriptional regulation of genes in a more active and delicate manner than has been expected; different CpGs independently read in binding signals of different TFs and integrate them.
Limited by the data source, we focused on the CpGs that were included in HumanMethylation450k array, which contained probes that mainly targeted CpGs in the transcribed region of genes or that were nearby gene TSSs. This most likely reflects an inherent genetic bias that may or may not be intensified by the array platform. After correcting for this bias, our results indicate that genomic coordinates localized more than 1kb downstream of gene TSS tend to have a higher fraction of significantly methylated CpGs. This challenges the notion that methylated CpGs proximal to gene TSSs are the major players in gene expression regulation. A recent paper by Aran et al. explored the relationship between the DNA methylation of distal regulatory sites and the dysfunctional regulation of cancer genes. They showed that hypomethylated enhancer sites correlated with upregulation of cancer-related genes and hypermethylated sites with downregulation. Moreover, the association between enhancer methylation and gene deregulation in cancer was significantly stronger than the association of promoter methylation with gene deregulation.Citation45 It would be interesting to investigate the effect on TF binding on methylation of CpGs located in enhancers.
Thurman et al. observed in ENCODE cell lines that the methylation levels of TF binding sites were correlated with the expression levels of the corresponding TFs, and proposed that DNA methylation might be a passive reflection of transcription factor binding, i.e., filling in the voids left by vacating transcription factors. Here we validated their observations in tumor samples from patients with breast cancer. We found that the methylation levels of ERα binding CpGs tended to be lower in ER+ than in ER- samples. Compared with the ER- samples, ER+ samples have significantly higher ERα activity. Additionally, we also confirmed that binding of some TFs (e.g., ERα and FOXA1) were associated with reduced methylation levels, while binding of other TFs (such as SUZ12 and CtBP2) were associated with enhancer methylation levels.
Overall, our study integrates multiple large-scale data sets from TCGA and ENCODE to construe the association of DNA methylation patterning with the underlying transcriptional machinery within specific regions of the genome, in particular TF-binding sites and DHS regions. We expand on prior studies by providing a high-resolution analysis that illustrates the potential mechanistic relationship between CpG methylation and TF binding and describe how it affects differential gene expression observed between ER+ and ER- breast carcinomas. More specifically, we are able to assess the CpG methylation patterning at specific binding sites and show how it can influence cancer phenotype via its interaction with transcription factors. To our knowledge, this is the most comprehensive analysis of DNA methylation in breast cancer since we have used data from the latest HM450K technology coupled with gene expression data from 222 TCGA primary breast carcinoma samples, along with ENCODE ChIP-seq TF profiles. By understanding how DNA methylation patterning affects the activity of specific transcription factors, we can better determine molecular characteristics of patients with tumors. For example, if we can dissect the “methylation code” of a transcription factor, we can use the information to understand the transcriptional aberrations implicated in tumor types. This may aid in the development of biomarkers and/or targeted therapy.
Materials and Methods
Data sets
The gene expression and DNA methylation data for breast cancer patients were downloaded from the TCGA (The Cancer Genome Atlas) project at https://tcga-data.nci.nih.gov/tcga/. Expression levels of genes were quantified using the two-channel Agilent microarrays. Methylation levels of CpG were measured using the HumanMethylation450 arrays, and represented as β values. The β value is a quantitative measure of DNA methylation levels of specific CpGs, which ranges from 0 for completely unmethylated to 1 for completely methylated.
The genome wide TF binding data were generated by ENCODE (The Encyclopedia of DNA Elements) project based on ChIP-seq experiments. We downloaded the binding peaks of TFs from UCSC Genome browser at http://genome.ucsc.edu/ENCODE/downloads.html. We used the binding peaks identified by the peak calling algorithm, PeakSeq.Citation13 The data set contains TF binding data in a number of different cell lines, from which we only select the data in breast epithelial cell lines (MCF7 and T47D) for our analysis to achieve the best match with data from TCGA.
The DNase hypersensitivity data were generated by ENCODE project based on DNase-seq experiments and were downloaded from UCSC Genome browser. The data provide a complete list of DNA regions that are sensitive to DNase I treatment, also known as DNase hypersensitive sites. Again, to match TCGA data, we only selected DNase data obtained from MCF7 and T47d cell lines.
Differential DNA methylation between ER+ and ER- breast cancer samples
The DNA methylation data from TCGA contains methylation levels of 485 577 CpGs in 630 ER+ and 187 ER- breast cancer samples. Most of the CpGs can be associated with a gene based on their localization: in the transcribed region or proximal to the transcription start site of a gene. For each CpG, we compared its β values in ER+ with respect to ER- samples by using the Student t test. Given a significance cut-off (e.g., P < 0.001), we identified a hypermethylated CpG set and a hypo-methylated CpG set in ER+ samples with respect to ER- samples. We examined a number of different cut-off values for significance.
Differential gene expression between ER+ and ER- breast cancer samples
The gene expression data from TCGA contains expression levels of 17 814 human genes in 401 ER+ and 118 ER- breast cancer samples. We compared the expression levels of genes between ER+ and ER- samples using the Student i test. By setting a cut-off value of P < 0.001, we divided genes into three classes: upregulated in ER+, downregulated in ER+, and non-differentially expressed genes.
Relating CpGs with ER binding, other TF binding, and DNase I hypersensitive sites
Given the complete list of ERα binding peaks in a cell line (e.g., T47d), we can determine whether a CpG is located within an ER binding peak. We defined ER binding CpGs as those falling into an ERα binding peak. In general, a gene is associated with multiple CpGs in HumanMethylation450k array. In this study, we aim to investigate the local effect of ERα binding on DNA methylation, thus we defined non-ERα binding CpGs as those not in any ERα binding peak, but were associated a gene with at least one ERα binding CpG. Since both ERα binding CpGs and non-ERα binding CpGs are from genes associated with at least one ERα binding peak, for which we expect comparable global effect (i.e., effect of ERα binding on genes) of ERα binding. This enables us to investigate the local effect of ERα binding on DNA methylation by comparing ERα binding CpGs and non-ERα binding CpGs.
In a similar way, we defined the DHS-associated CpGs and non-DHS associated CpGs. Based on the binding data of other TFs, we defined TFBS associated and non-TFBS associated CpG sites separately for each TF with ChIP-seq data.
Correlation of DNA methylation with gene expression
Gene expression data and DNA methylation data are available for 222 of the TCGA breast cancer samples. In this data, we investigate the correlation between DNA methylation of CpGs with gene expression. For each CpG, we calculated the Spearman correlation coefficient of its β values with the expression levels of its associated gene across all the samples. The correlation between methylation level of a CpG and the expression level of ESR1 was calculated in the same way.
Additional material
Download Zip (129.9 KB)Disclosure of Potential Conflicts of Interest
No potential conflicts of interest were disclosed.
Acknowledgments
This work was supported by the American Cancer Society Research Grant, #IRG-82-003-27, and by the start-up funding package provided to C.C. by the Geisel School of Medicine at Dartmouth College.
References
- Barlow DP. Genomic imprinting: a mammalian epigenetic discovery model. Annu Rev Genet 2011; 45:379 - 403; http://dx.doi.org/10.1146/annurev-genet-110410-132459; PMID: 21942369
- Riggs AD. X inactivation, differentiation, and DNA methylation. Cytogenet Cell Genet 1975; 14:9 - 25; http://dx.doi.org/10.1159/000130315; PMID: 1093816
- Yoder JA, Walsh CP, Bestor TH. Cytosine methylation and the ecology of intragenomic parasites. Trends Genet 1997; 13:335 - 40; http://dx.doi.org/10.1016/S0168-9525(97)01181-5; PMID: 9260521
- Meissner A. Epigenetic modifications in pluripotent and differentiated cells. Nat Biotechnol 2010; 28:1079 - 88; http://dx.doi.org/10.1038/nbt.1684; PMID: 20944600
- Reik W. Stability and flexibility of epigenetic gene regulation in mammalian development. Nature 2007; 447:425 - 32; http://dx.doi.org/10.1038/nature05918; PMID: 17522676
- Martin M, Herceg Z. From hepatitis to hepatocellular carcinoma: a proposed model for cross-talk between inflammation and epigenetic mechanisms. Genome Med 2012; 4:8; http://dx.doi.org/10.1186/gm307; PMID: 22293089
- Baylin SB, Jones PA. A decade of exploring the cancer epigenome - biological and translational implications. Nat Rev Cancer 2011; 11:726 - 34; http://dx.doi.org/10.1038/nrc3130; PMID: 21941284
- Jaenisch R, Bird A. Epigenetic regulation of gene expression: how the genome integrates intrinsic and environmental signals. Nat Genet 2003; 33:Suppl 245 - 54; http://dx.doi.org/10.1038/ng1089; PMID: 12610534
- Berman BP, Weisenberger DJ, Aman JF, Hinoue T, Ramjan Z, Liu Y, Noushmehr H, Lange CP, van Dijk CM, Tollenaar RA, et al. Regions of focal DNA hypermethylation and long-range hypomethylation in colorectal cancer coincide with nuclear lamina-associated domains. Nat Genet 2012; 44:40 - 6; http://dx.doi.org/10.1038/ng.969; PMID: 22120008
- Hartmann O, Spyratos F, Harbeck N, Dietrich D, Fassbender A, Schmitt M, Eppenberger-Castori S, Vuaroqueaux V, Lerebours F, Welzel K, et al. DNA methylation markers predict outcome in node-positive, estrogen receptor-positive breast cancer with adjuvant anthracycline-based chemotherapy. Clin Cancer Res 2009; 15:315 - 23; http://dx.doi.org/10.1158/1078-0432.CCR-08-0166; PMID: 19118060
- Cancer Genome Atlas N, Cancer Genome Atlas Network. Comprehensive molecular portraits of human breast tumours. Nature 2012; 490:61 - 70; http://dx.doi.org/10.1038/nature11412; PMID: 23000897
- Hill VK, Ricketts C, Bieche I, Vacher S, Gentle D, Lewis C, Maher ER, Latif F. Genome-wide DNA methylation profiling of CpG islands in breast cancer identifies novel genes associated with tumorigenicity. Cancer Res 2011; 71:2988 - 99; http://dx.doi.org/10.1158/0008-5472.CAN-10-4026; PMID: 21363912
- Rozowsky J, Euskirchen G, Auerbach RK, Zhang ZD, Gibson T, Bjornson R, Carriero N, Snyder M, Gerstein MB. PeakSeq enables systematic scoring of ChIP-seq experiments relative to controls. Nat Biotechnol 2009; 27:66 - 75; http://dx.doi.org/10.1038/nbt.1518; PMID: 19122651
- Fackler MJ, Umbricht CB, Williams D, Argani P, Cruz LA, Merino VF, Teo WW, Zhang Z, Huang P, Visvananthan K, et al. Genome-wide methylation analysis identifies genes specific to breast cancer hormone receptor status and risk of recurrence. Cancer Res 2011; 71:6195 - 207; http://dx.doi.org/10.1158/0008-5472.CAN-11-1630; PMID: 21825015
- Fang F, Turcan S, Rimner A, Kaufman A, Giri D, Morris LG, Shen R, Seshan V, Mo Q, Heguy A, et al. Breast cancer methylomes establish an epigenomic foundation for metastasis. Sci Transl Med 2011; 3:75ra25; http://dx.doi.org/10.1126/scitranslmed.3001875; PMID: 21430268
- Dedeurwaerder S, Desmedt C, Calonne E, Singhal SK, Haibe-Kains B, Defrance M, Michiels S, Volkmar M, Deplus R, Luciani J, et al. DNA methylation profiling reveals a predominant immune component in breast cancers. EMBO Mol Med 2011; 3:726 - 41; http://dx.doi.org/10.1002/emmm.201100801; PMID: 21910250
- Bediaga N, Acha-Sagredo A, Guerra I, Viguri A, Albaina C, Ruiz Diaz I, et al. DNA methylation epigenotypes in breast cancer molecular subtypes. BCR 2010; 12.
- Holm K, Hegardt C, Staaf J, Vallon-Christersson J, Jonsson G, Olsson H, et al. Molecular subtypes of breast cancer are associated with characteristic DNA methylation patterns. BCR 2010; 12.
- Martens JW, Margossian AL, Schmitt M, Foekens J, Harbeck N. DNA methylation as a biomarker in breast cancer. Future Oncol 2009; 5:1245 - 56; http://dx.doi.org/10.2217/fon.09.89; PMID: 19852739
- Ulirsch J, Fan C, Knafl G, Wu MJ, Coleman B, Perou CM, Swift-Scanlan T. Vimentin DNA methylation predicts survival in breast cancer. Breast Cancer Res Treat 2013; 137:383 - 96; http://dx.doi.org/10.1007/s10549-012-2353-5; PMID: 23239149
- Choy M-K, Movassagh M, Goh H-G, Bennett MR, Down TA, Foo RS. Genome-wide conserved consensus transcription factor binding motifs are hyper-methylated. BMC Genomics 2010; 11:519; http://dx.doi.org/10.1186/1471-2164-11-519; PMID: 20875111
- Comb M, Goodman HM. CpG methylation inhibits proenkephalin gene expression and binding of the transcription factor AP-2. Nucleic Acids Res 1990; 18:3975 - 82; http://dx.doi.org/10.1093/nar/18.13.3975; PMID: 1695733
- Miranda TB, Jones PA. DNA methylation: the nuts and bolts of repression. J Cell Physiol 2007; 213:384 - 90; http://dx.doi.org/10.1002/jcp.21224; PMID: 17708532
- Prendergast GC, Lawe D, Ziff EB. Association of Myn, the murine homolog of max, with c-Myc stimulates methylation-sensitive DNA binding and ras cotransformation. Cell 1991; 65:395 - 407; http://dx.doi.org/10.1016/0092-8674(91)90457-A; PMID: 1840505
- Höller M, Westin G, Jiricny J, Schaffner W. Sp1 transcription factor binds DNA and activates transcription even when the binding site is CpG methylated. Genes Dev 1988; 2:1127 - 35; http://dx.doi.org/10.1101/gad.2.9.1127; PMID: 3056778
- Filion GJ, Zhenilo S, Salozhin S, Yamada D, Prokhortchouk E, Defossez P-A. A family of human zinc finger proteins that bind methylated DNA and repress transcription. Mol Cell Biol 2006; 26:169 - 81; http://dx.doi.org/10.1128/MCB.26.1.169-181.2006; PMID: 16354688
- Hashimshony T, Zhang J, Keshet I, Bustin M, Cedar H. The role of DNA methylation in setting up chromatin structure during development. Nat Genet 2003; 34:187 - 92; http://dx.doi.org/10.1038/ng1158; PMID: 12740577
- Hendrich B, Bird A. Identification and characterization of a family of mammalian methyl-CpG binding proteins. Mol Cell Biol 1998; 18:6538 - 47; PMID: 9774669
- Johnson LM, Bostick M, Zhang X, Kraft E, Henderson I, Callis J, Jacobsen SE. The SRA methyl-cytosine-binding domain links DNA and histone methylation. Curr Biol 2007; 17:379 - 84; http://dx.doi.org/10.1016/j.cub.2007.01.009; PMID: 17239600
- Rottach A, Frauer C, Pichler G, Bonapace IM, Spada F, Leonhardt H. The multi-domain protein Np95 connects DNA methylation and histone modification. Nucleic Acids Res 2010; 38:1796 - 804; http://dx.doi.org/10.1093/nar/gkp1152; PMID: 20026581
- Prokhortchouk A, Hendrich B, Jørgensen H, Ruzov A, Wilm M, Georgiev G, Bird A, Prokhortchouk E. The p120 catenin partner Kaiso is a DNA methylation-dependent transcriptional repressor. Genes Dev 2001; 15:1613 - 8; http://dx.doi.org/10.1101/gad.198501; PMID: 11445535
- Jones PA. Functions of DNA methylation: islands, start sites, gene bodies and beyond. Nat Rev Genet 2012; 13:484 - 92; http://dx.doi.org/10.1038/nrg3230; PMID: 22641018
- Thurman RE, Rynes E, Humbert R, Vierstra J, Maurano MT, Haugen E, Sheffield NC, Stergachis AB, Wang H, Vernot B, et al. The accessible chromatin landscape of the human genome. Nature 2012; 489:75 - 82; http://dx.doi.org/10.1038/nature11232; PMID: 22955617
- Szyf M. DNA methylation signatures for breast cancer classification and prognosis. Genome Med 2012; 4:26; http://dx.doi.org/10.1186/gm325; PMID: 22494847
- Piva R, Rimondi AP, Hanau S, Maestri I, Alvisi A, Kumar VL, del Senno L. Different methylation of oestrogen receptor DNA in human breast carcinomas with and without oestrogen receptor. Br J Cancer 1990; 61:270 - 5; http://dx.doi.org/10.1038/bjc.1990.50; PMID: 2155643
- Li L, Lee KM, Han W, Choi JY, Lee JY, Kang GH, Park SK, Noh DY, Yoo KY, Kang D. Estrogen and progesterone receptor status affect genome-wide DNA methylation profile in breast cancer. Hum Mol Genet 2010; 19:4273 - 7; http://dx.doi.org/10.1093/hmg/ddq351; PMID: 20724461
- Widschwendter M, Siegmund KD, Müller HM, Fiegl H, Marth C, Müller-Holzner E, Jones PA, Laird PW. Association of breast cancer DNA methylation profiles with hormone receptor status and response to tamoxifen. Cancer Res 2004; 64:3807 - 13; http://dx.doi.org/10.1158/0008-5472.CAN-03-3852; PMID: 15172987
- Ruike Y, Imanaka Y, Sato F, Shimizu K, Tsujimoto G. Genome-wide analysis of aberrant methylation in human breast cancer cells using methyl-DNA immunoprecipitation combined with high-throughput sequencing. BMC Genomics 2010; 11:137; http://dx.doi.org/10.1186/1471-2164-11-137; PMID: 20181289
- Bernstein BE, Birney E, Dunham I, Green ED, Gunter C, Snyder M, ENCODE Project Consortium. An integrated encyclopedia of DNA elements in the human genome. Nature 2012; 489:57 - 74; http://dx.doi.org/10.1038/nature11247; PMID: 22955616
- Theodorou V, Stark R, Menon S, Carroll JS. GATA3 acts upstream of FOXA1 in mediating ESR1 binding by shaping enhancer accessibility. Genome Res 2013; 23:12 - 22; http://dx.doi.org/10.1101/gr.139469.112; PMID: 23172872
- Watters RJ, Benos PV, Oesterreich S. To bind or not to bind--FoxA1 determines estrogen receptor action in breast cancer progression. Breast Cancer Res 2012; 14:312; http://dx.doi.org/10.1186/bcr3146; PMID: 22713214
- Margueron R, Reinberg D. The Polycomb complex PRC2 and its mark in life. Nature 2011; 469:343 - 9; http://dx.doi.org/10.1038/nature09784; PMID: 21248841
- Kennison JA. The Polycomb and trithorax group proteins of Drosophila: trans-regulators of homeotic gene function. Annu Rev Genet 1995; 29:289 - 303; http://dx.doi.org/10.1146/annurev.ge.29.120195.001445; PMID: 8825476
- Chinnadurai G. CtBP, an unconventional transcriptional corepressor in development and oncogenesis. Mol Cell 2002; 9:213 - 24; http://dx.doi.org/10.1016/S1097-2765(02)00443-4; PMID: 11864595
- Aran D, Sabato S, Hellman A. DNA methylation of distal regulatory sites characterizes dysregulation of cancer genes. Genome Biol 2013; 14:R21; http://dx.doi.org/10.1186/gb-2013-14-3-r21; PMID: 23497655