ABSTRACT
Augmented reality (AR) has proven to be an invaluable interactive medium to reduce cognitive load by bridging the gap between the task-at-hand and relevant information by displaying information without disturbing the user's focus. AR is particularly useful in the manufacturing environment where a diverse set of tasks such as assembly and maintenance must be performed in the most cost-effective and efficient manner possible. While AR systems have seen immense research innovation in recent years, the current strategies utilised in AR for camera calibration, detection, tracking, camera position and orientation (pose) estimation, inverse rendering, procedure storage, virtual object creation, registration, and rendering are still mostly dominated by traditional non-AI approaches. This restricts their practicability to controlled environments with limited variations in the scene. Classical AR methods can be greatly improved through the incorporation of various AI strategies like deep learning, ontology, and expert systems for adapting to broader scene variations and user preferences. This research work provides a review of current AR strategies, critical appraisal for these strategies, and potential AI solutions for every component of the computational pipeline of AR systems. Given the review of current work in both fields, future research work directions are also outlined.
Disclosure statement
No potential conflict of interest was reported by the author(s).
Notes
1 Cognitive load is the mental effort needed for processing information (Porter and Heppelmann Citation2017).
2 Short-term trackers were supplied with the position of the target in each frame and did not include a target re-detection module. So, they failed at the first instance of occlusion (Kristan et al. Citation2019).
3 In addition to tracking, long-term trackers had to identify tracking failure and trigger the included target re-detection module. So, long term trackers were evaluated for their performance under occlusion (Kristan et al. Citation2019).
4 Short-term trackers evaluated for real-time performance (Kristan et al. Citation2019).
Additional information
Funding
Notes on contributors
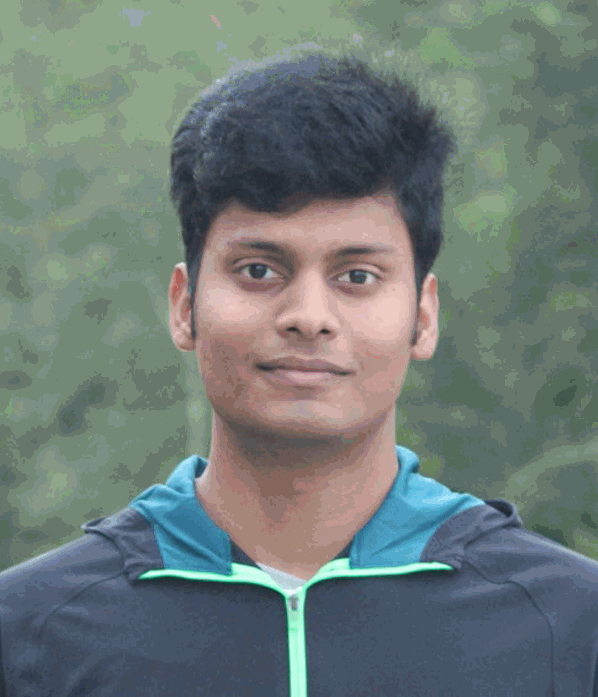
Chandan K. Sahu
Chandan K. Sahu received his B.Tech. degree from National Institute of Technology Rourkela, India in 2014, and his M.S. degree from the State University of New York at Buffalo, USA, in 2020, both in mechanical engineering. He worked at Honda Motorcycle and Scooter India, from 2014 to 2017. He is currently a research assistant at the Geometric Reasoning and Artificial Intelligence Laboratory (GRAIL) at Clemson University's International Center for Automotive Research (CU-ICAR). He is interested in machine learning in general, with a focus on their geometric attributes and mechanical applications. His research is focussed on augmented reality, additive manufacturing, cyber-physical systems and autonomous vehicle applications. His current research work emphasises on supplementing neural networks with physics models for modelling complex systems where only limited data and limited physics are available.
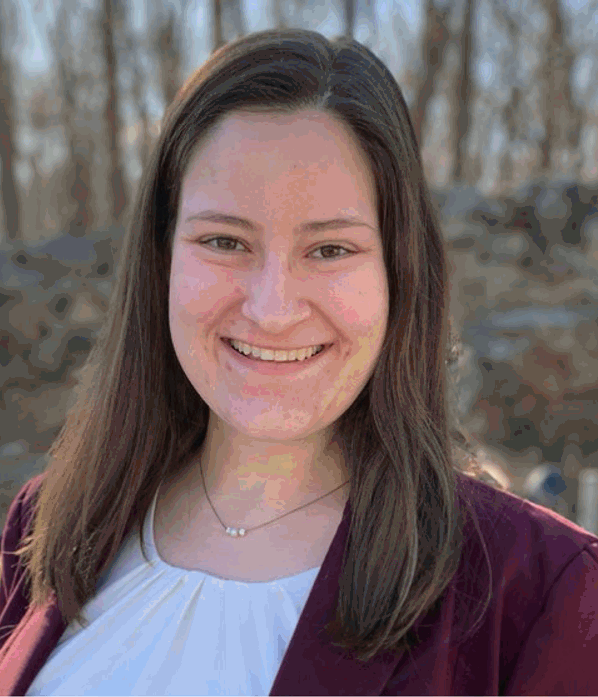
Crystal Young
Crystal Young received her B.S. degree in physics from Stony Brook University, Stony Brook, NY, in 2018, and her M.S. degree in mechanical engineering from the University at Buffalo, Buffalo, NY, in 2020. She also holds an Advanced Certificate in Advanced Manufacturing from the University at Buffalo, 2020. She recently completed an internship with the Naval Research Enterprise Internship Program in September 2020. Her current research interests include smart and sustainable manufacturing, optimal design, augmented reality, artificial intelligence, and assistive technologies. She is a member of Sigma Pi Sigma, The National Physics Honor Society.
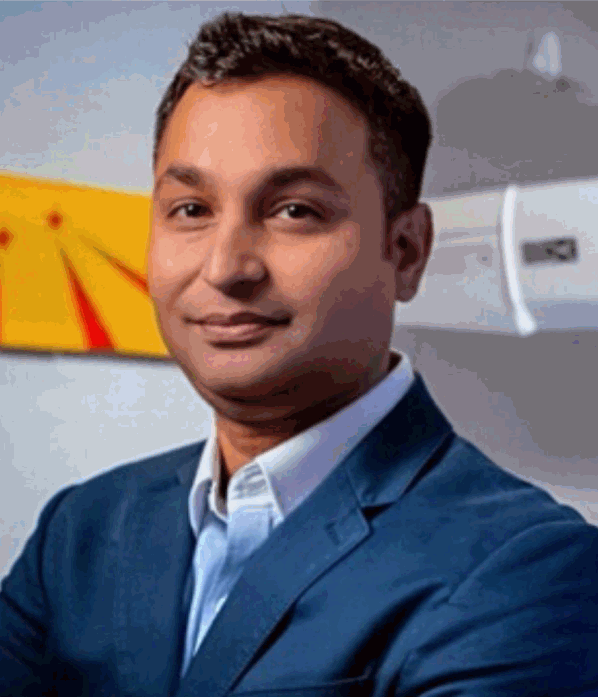
Rahul Rai
Rahul Rai received the B.Tech. degree from the National Institute of Foundry and Forge Technology (NIFFT), Ranchi, India, in 2000, the M.S. degree in manufacturing engineering from the Missouri University of Science and Technology (Missouri S&T), USA, in 2002, and the Ph.D. degree in mechanical engineering from The University of Texas at Austin, USA, in 2006. He is currently a Dean's Distinguished Professor in the Department of Automotive Engineering at the International Center for Automotive Engineering (ICAR) at Clemson University. Dr Rai's research is focussed on developing computational tools for Manufacturing, Cyber-Physical System (CPS) Design, Autonomy, Collaborative Human-Technology Systems, Diagnostics and Prognostics, and Extended Reality (XR) domains. By combining engineering innovations with methods from machine learning, AI, statistics and optimisation, and geometric reasoning, his research strives to solve important problems in the above-mentioned domains. His research has been supported by NSF, DARPA, ONR, NSWC, DMDII, HP, NYSERDA, and NYSPII. He has authored over 100 articles to date in peer-reviewed conferences and journals covering a wide array of problems. He was a recipient of the 2009 HP Design Innovation and the 2017 ASME IDETC/CIE Young Engineer Award. He also received the 2019 PHM Society Conference Best Paper Award.