Abstract
Manufacturing in the age of Industry 4.0 can be characterised by a high product variety and complex material flows. The increasing individualisation of products requires adaptive production planning and control systems. Research in the area of Machine Learning demonstrates the applicability and potential of Reinforcement Learning (RL) systems for the control of complex manufacturing. However, a major disadvantage of RL-methods is that they are usually considered as ‘black box’ models. For this reason, this paper investigates methods of explainable reinforcement learning in production control. Based on a comprehensive literature review an approach to increase the plausibility of RL-based control strategies is presented. The approach combines the advantages of high prediction accuracy (e.g. neural networks) and high explainability (e.g. decision trees). In doing so, understandable control strategies such as heuristics can be generated, and an advanced RL-system can be designed including specific domain expertise. The results are demonstrated based on a real-world system, taken from semiconductor manufacturing, which is investigated in a simulated approach.
Acknowledgments
In particular, we thank Stephen C. Graves Professor of Operations Management at the MIT Sloan School of Management for his support and fruitful discussions.
Disclosure statement
No potential conflict of interest was reported by the author(s).
Additional information
Funding
Notes on contributors
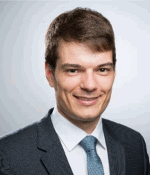
Andreas Kuhnle
Andreas Kuhnle is a researcher at the Institute for Production Science (wbk) at the Karlsruhe Institute of Technology (KIT). His research deals with the optimisation of production systems in planning and operation, primarily in discrete manufacturing. In close cooperation with industry from, for example, the semiconductor industries, innovative and application-oriented approaches are researched and implemented. This paper was part of a research stay at the MIT Sloan School of Management supervised by Stephen C. Graves.
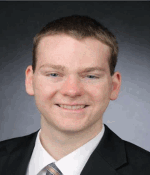
Marvin Carl May
Marvin Carl May is a researcher at the Institute for Production Science (wbk) at the Karlsruhe Institute of Technology (KIT). His research concerns production system optimisation on strategical, tactical and operational levels by means of intelligent algorithms. The focus lies on discrete manufacturing, in close cooperation with industry, e.g. semiconductor manufacturing, and aims at researching and implementing innovative and application-oriented solutions to technological problems.
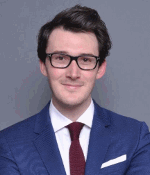
Louis Schäfer
Louis Schäfer is a researcher at the Institute for Production Science (wbk) at the Karlsruhe Institute of Technology (KIT). His research focuses on automated production system planning with the goal of realising an integrated product-production-codesign. In close cooperation with industry partners from sectors such as the automotive supply industry, he conducts research at the interface between product development and production planning by, for example, automatically generating production sequences directly from CAD product data.
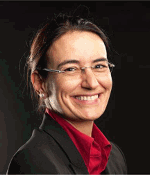
Gisela Lanza
Gisela Lanza is member of the management board at the Institute of Production Science (wbk) of the Karlsruhe Institute of Technology (KIT). She heads the Production Systems division dealing with the topics of global production strategies, production system planning, and quality assurance in research and industrial practice. Her research focus is on the holistic design and evaluation of production systems. The methodological approach includes the use of quantitative methods to increase efficiency. In addition, a special focus is placed on data-driven planning and control of production networks in order to translate corporate strategy into tactical and operative network design.