ABSTRACT
Time series forecasting plays an increasingly important role in modern business decisions. In today's data-rich environment, people often aim to choose the optimal forecasting model for their data. However, identifying the optimal model requires professional knowledge and experience, making accurate forecasting a challenging task. To mitigate the importance of model selection, we propose a simple and reliable algorithm to improve the forecasting performance. Specifically, we construct multiple time series with different sub-seasons from the original time series. These derived series highlight different sub-seasonal patterns of the original series, making it possible for the forecasting methods to capture diverse patterns and components of the data. Subsequently, we produce forecasts for these multiple series separately with classical statistical models (ETS or ARIMA). Finally, the forecasts are combined. We evaluate our approach on widely used forecasting competition data sets (M1, M3, and M4) in terms of both point forecasts and prediction intervals. We observe performance improvements compared with the benchmarks. Our approach is particularly suitable and robust for the data with higher frequency. To demonstrate the practical value of our proposition, we showcase the performance improvements from our approach on hourly load data that exhibit multiple seasonal patterns.
Acknowledgments
Fotios Petropoulos thanks Ivan Svetunkov for his feedback in the early stages of the development of the algorithm proposed in this paper.
Data availability statement
The M1 and M3 data sets that support the findings of this study are publicly available in the Mcomp R package (Hyndman Citation2018), and the M4 data set in the M4comp2018 R package (Montero-Manso, Netto, and Talagala Citation2018). Both the two data sets of electricity load for the application studies in Section 5 are publicly available at National Grid .Footnote1
Disclosure statement
No potential conflict of interest was reported by the author(s).
Notes
Additional information
Funding
Notes on contributors
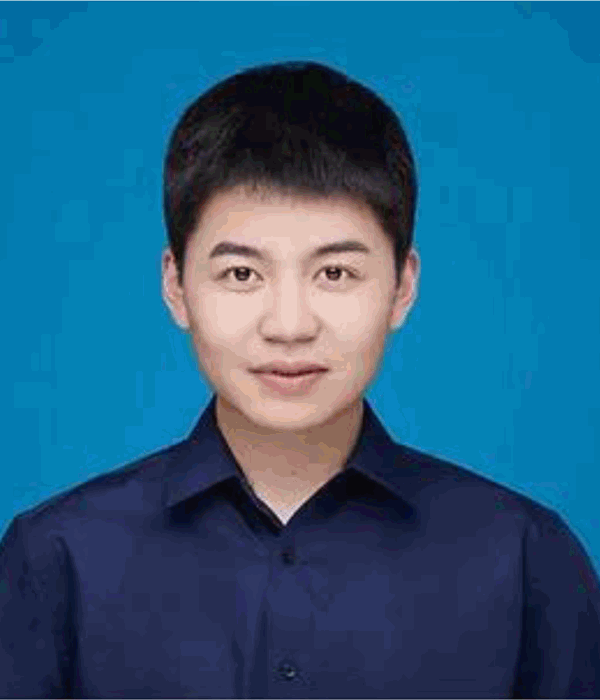
Xixi Li
Xixi Li is a Ph.D. student in Statistics at The University of Manchester. Prior to that, he obtained his Master's and Bachelor's degree from Beihang University. His current research aims to (i) design statistical time series models that incorporate the components of deep learning; and (ii) develop computationally efficient statistical models for integer-valued time series analysis.
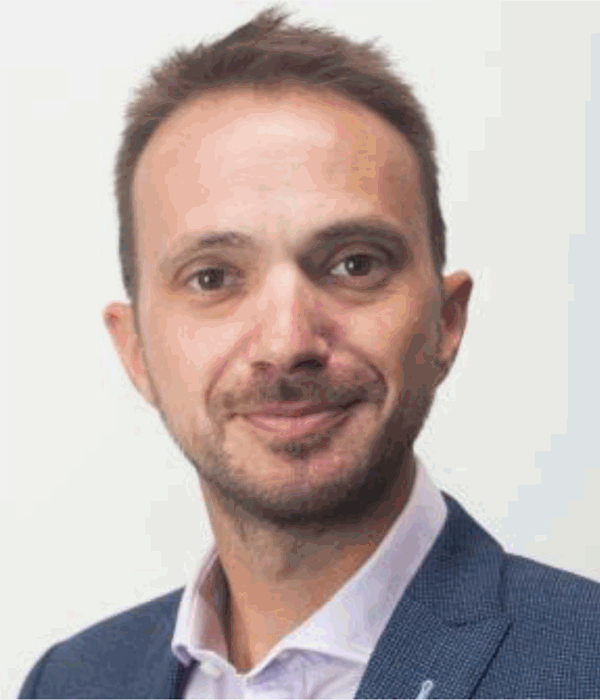
Fotios Petropoulos
Fotios Petropoulos is Professor at the School of Management of the University of Bath, Editor of the International Journal of Forecasting and the Forecasting Support Systems Editor of Foresight. His research expertise lies in behavioural aspects of forecasting and improving the forecasting process, applied in the context of business and supply chain. He is the co-founder of the Forecasting Society (http://www.forsoc.net/).
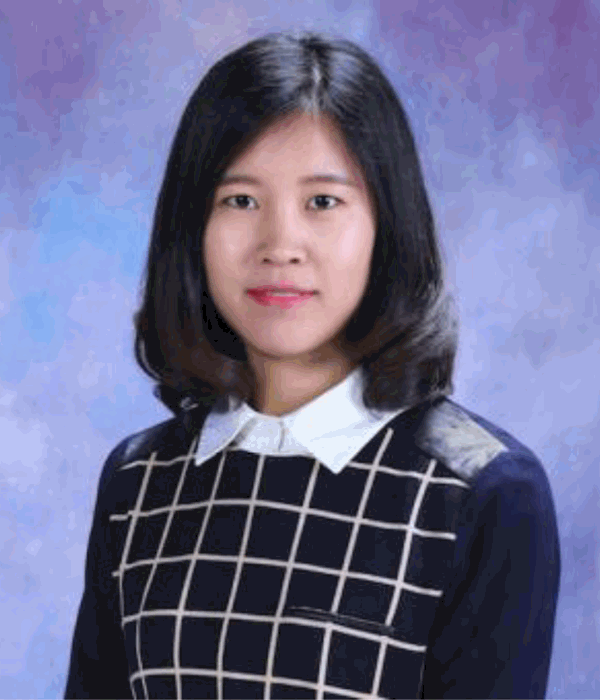
Yanfei Kang
Yanfei Kang is Associate Professor of Statistics in the School of Economics and Management at Beihang University in China. Prior to that, she was Senior R&D Engineer in Big Data Group of Baidu Inc. Yanfei obtained her Ph.D. degree of Applied and Computational Mathematics at Monash University in 2014. She worked as a postdoctoral research fellow in feature-based time series forecasting during 2014 and 2015 at Monash University. Her research interests include time series forecasting, time series visualisation, statistical computing and machine learning.