Abstract
Motivated by the practical research and development (R&D) process in high-end equipment manufacturing, this study investigates a bi-level scheduling problem in a complex R&D project network, where each project contains multiple modules with a complete task network. In the bi-level scheduling problem, the upper-level problem is that the R&D project leader makes the decision on allocating all R&D project modules to limited R&D researchers and the objective is to minimise the total penalty cost of all projects, and the lower-level problem is that the researchers schedule and sort the assigned tasks to minimise their minimum makespan. The different capacity of researchers is considered, and some structural properties are derived based on the capacity analytics. To tackle this complex scheduling problem, an effective Variable Neighborhood Search algorithm based on the ‘less is more' concept is proposed, where a Multi-Greedy Heuristic is incorporated. Interestingly, we observe that simpler algorithmic strategies may lead to better algorithmic performance. Computational experiments are carried out to demonstrate that the performance of the proposed algorithm is efficient and stable.
Data availability statement
The authors confirm that the data supporting the findings of this study are available within the article and its Appendix.
Additional information
Funding
Notes on contributors
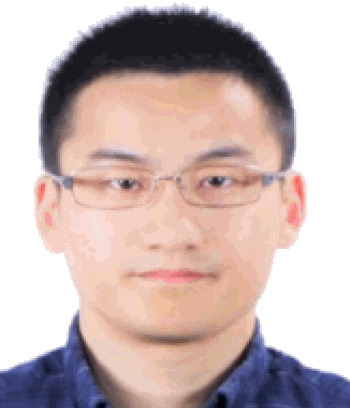
Jun Pei
Jun Pei serves as a Professor in the School of Management, Hefei University of Technology, China. His research interests cover production scheduling, business analytics, industrial internet, and optimisation in smart manufacturing. His research has appeared in premier academic journals, such as Production and Operations Management, INFORMS Journal on Computing, Omega and European Journal of Operational Research. He also serves as Co-Editor-in-Chief for Energy Systems, Associate Editor for Journal of Global Optimisation, Journal of Combinatorial Optimisation, Optimisation Letters, Computational Social Networks, and SN Operations Research Forum, and Lead Guest Editor for Annals of Operations Research.
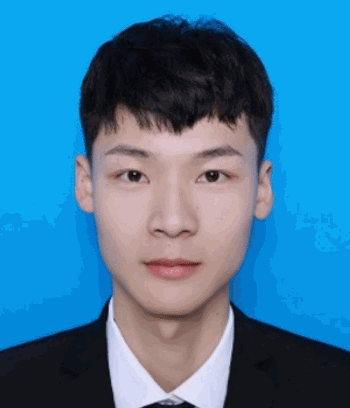
Haoxin Wang
Haoxin Wang received Master's Degree in School of Management, Hefei University of Technology, China. His research has appeared in Optimisation Letters. Currently, he is an Engineer in the NIO company, China.
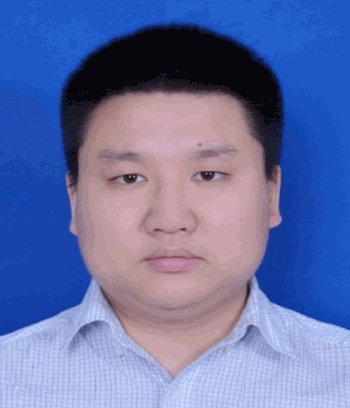
Min Kong
Min Kong received his Bachelor’s degree in Management from Hefei University of Technology, Hefei, China, in 2015. He received his Ph.D. degree in Management from the Hefei University of Technology in 2020. He serves as assistant professor in School of Management, Anhui Normal University, China. His research interests include supply chain scheduling and application of the Internet of Things. He has published papers in Journal of Global Optimisation, International Journal of Production Research, and Annals of Operations research, etc.
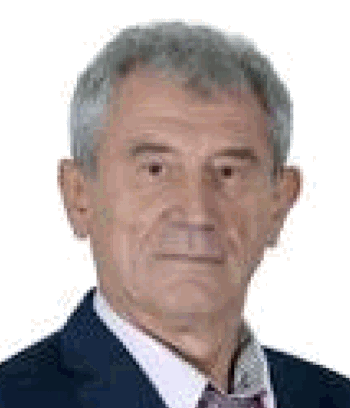
Nenad Mladenovic
Nenad Mldenovic is a Member of the European academy of sciences (Academia Europea). He leads or participates in several international research projects in the United Kingdom, France, China, Russia, Kazakhstan, and Serbia. His research has appeared in Operations Research, Mathematical Programming, INFORMS Journal on Computing, European Journal of Operational Research, etc, and he is a highly cited researcher and has more than 22,000 citations according to Google scholar. Prof. Nenad Mldenovic is the founder of Variable Neighborhood Search (VNS). His paper on the VNS metaheuristic with P. Hansen was selected among the 30 most influential published in EJOR in the 30-year history of EJOR and ranked as 8th most cited in 40 years of EJOR. Currently, he is a full professor at Khalifa University, Abu Dhabi, UAE.
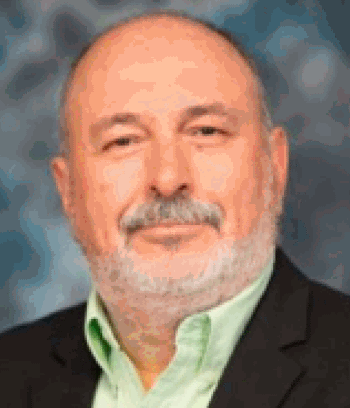
Panos M. Pardalos
Panos M. Pardalos is currently a Distinguished Professor and the Paul and Heidi Brown Preeminent Professor with the Departments of Industrial and Systems Engineering, University of Florida, USA, and a world-renowned leader in global optimisation, mathematical modeling, and data sciences. He has published more than 500 journal papers, and edited/authored more than 200 books. He is a Fellow of AAAS, AIMBE, and INFORMS and received the 2013 Constantin Caratheodory Prize of the International Society of Global Optimization. In addition, he received the 2013 EURO Gold Medal prize bestowed by the Association for European Operational Research Societies. He also received the prestigious Humboldt Research Award (2018–2019). He is also a Member of the Lithuanian Academy of Sciences, the Royal Academy of Spain, and the National Academy of Sciences of Ukraine. He is the Founding Editor of Optimisation Letters, Energy Systems, and Co-Founder of the International Journal of Global Optimisation, and Computational Management Science.