ABSTRACT
Product configuration design combines the existing configurable components of enterprises to quickly form a new product that meets customised requirements. However, the representation, maintenance, and update of the configuration knowledge, and the mismatch problem restrict the rationality and efficiency of existing configuration design methods. In this paper, a recommendation model is developed for customised product configuration design, which takes personalised customer requirements and product component information as input and outputs a ranked list of component instances. It consists of two sub-models: a retrieval sub-model and a ranking sub-model. The retrieval sub-model selects a set of component instance candidates from all possible candidates, and then the ranking sub-model ranks them and selects the best possible candidate. To boost the ranking sub-model performance, we propose a novel interacting network, DualAdap, to extract meaningful low-order, high-order, and adaptive-order cross features. Based on learned cross features, the ranking sub-model computes the adoption scores of all candidates and then selects the best possible candidate according to adoption scores. The configuration design of the elevator traction machine is taken as a case study. Results verify that our recommendation model can identify similar component instances and then accurately pick out the best possible candidate that meets customer requirements.
Disclosure statement
No potential conflict of interest was reported by the author(s).
Data availability statement
The data that support the findings of this study are openly available at: https://github.com/WennyZhou/Recommend4configuration.
Notes
Additional information
Funding
Notes on contributors
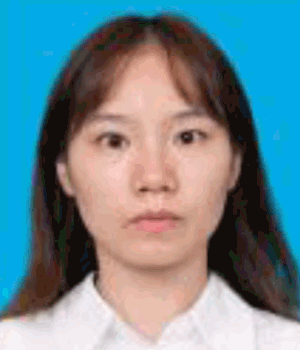
Huifang Zhou
Huifang Zhou received the B.S. degree in School of mechanical engineering from Shandong University, China, in 2017. She is currently a Ph.D. candidate in the School of Mechanical Engineering, Zhejiang University, China. Her research interests include engineering optimisation, product configuration design and machine learning algorithms.
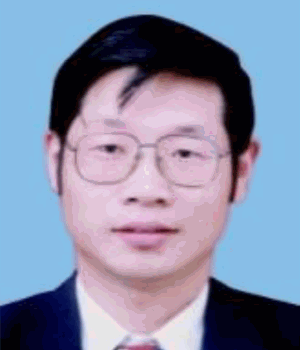
Shuyou Zhang
Shuyou Zhang was born in Zhejiang, China in 1963. He received his M.S. degree in Department of Mechanical Engineering from Zhejiang University in 1991, the Ph.D. degree in State Key Lab. of CAD&CG from Zhejiang University in 1999. He is currently a Professor in Zhejiang University, assistant director of Computer Graphics Professional Committee for China Engineering Graphic Society. His research search areas and descriptors include (1) Technology of Product Digital Design; (2) Technology of Design and Stimulation for Complex Equipments; (3) Engineering and Computer Graphics; (4) The Key Technology for Enterprise Informatisation.
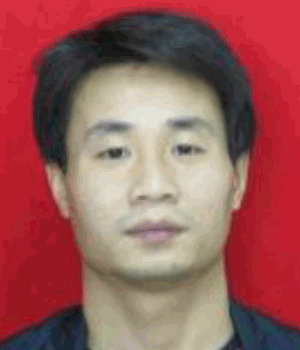
Lemiao Qiu
Lemiao Qiu received his Ph.D. degree in Mechanical Engineering in State Key Lab of CAD&CG from Zhejiang University, China, in 2008. He is currently an associate professor in the Department of Mechanical Engineering, Zhejiang University, China. His research interests include product digital design, manufacturing informatisation, design and stimulation for complex equipment.
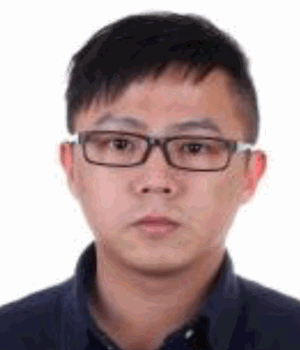
Zili Wang
Zili Wang is currently a Research Associate at the Department of Mechanical Engineering, Zhejiang University, Hangzhou, China. He received the Ph.D. degree from the Department of Mechanical Engineering, Zhejiang University, Hangzhou, China, in 2018. His research interests include computer-aided design, intelligent manufacturing, digital-twin-based design, and designing optimisation.
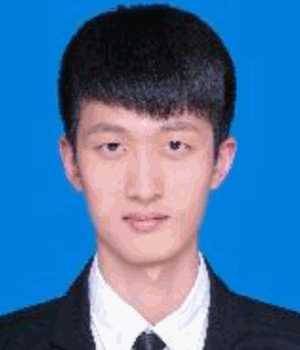
Kerui Hu
Kerui Hu received the B.Eng. degree in the School of China University of Mining and Technology, Xuzhou, China, in 2018. He is currently a Ph.D. candidate in the School of Mechanical Engineering, Zhejiang University, China. His research interests include data mining and collaborative filtering.