Abstract
The majority of warehouse expenses is driven by labour cost. Therefore, efficient management of labour resources is required. To do so, workforce planning is used to match the workforce capacity with the incoming workload. While doing so, it is often wrongly assumed that each worker has the same and constant capacity or performance. Addressing this, we build a model to predict the employee-based productivity of newly hired warehouse personnel that will support workforce planning by incorporating multiple data sources. To this end, we develop a framework to identify relevant variables in four categories: warehouse, operator, shift and product. We demonstrate that Extreme Gradient Boosting, using these variables may reduce the root mean squared error of the prediction by more than 50%. A comprehensive scenario analysis shows that improving productivity predictions translates into substantial cost savings. Furthermore, a sensitivity analysis identifies which variable categories should be favoured in the data collection process to achieve the best prediction results.
Abbreviations: CMA: Cumulative Moving Averages; EGB: Extreme Gradient Boosting; GB: Gradient Boosting; LSP: Logistics Service Provider; MAE: Mean Absolute Error; RF: Random Forest; RMSE: Root Mean Squared Error; SMA: Simple Moving Averages
Data availability statement
The collected data are proprietary to the Logistics Service Provider and contain records of employees' personal information, which is why the data cannot be made publicly available.
Disclosure statement
No potential conflict of interest was reported by the author(s).
Notes
1 Outliers are observations beyond the whiskers that extend the inner quartiles by 1.5 times the interquartile range.
2 Significance of p<0.01 for cross-validated RMSE versus all other models
Additional information
Notes on contributors
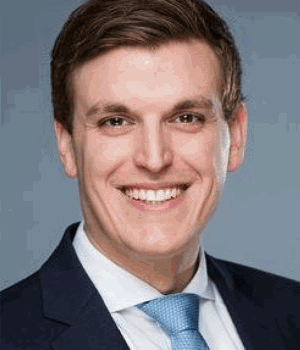
Sven F. Falkenberg
Sven Falkenberg is a doctoral student and research assistant at the chair of Logistics Management at WHU – Otto Beisheim School of Management. His research involves the use of novel data, machine learning and other algorithms in the warehouse context. He holds a B.Sc. and M.Sc. in Supply Chain Management from the University of Cologne with stays at the University of Strathclyde in Glasgow, Scotland, and the Indian Institute of Management in Ahmedabad, India. His studies focused on supply chain strategies, location planning, logistics and inventory management. The focus of his thesis was on safety stock considerations in cyclic production environments.
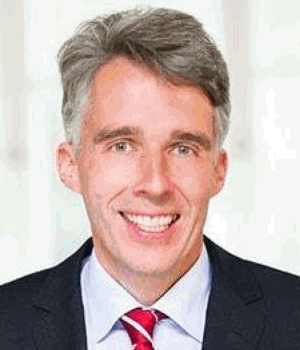
Stefan Spinler
Stefan Spinler holds the Chair of Logistics Management at WHU – Otto Beisheim School of Management. He received a master's degree in electrical engineering from Friedrich–Alexander University in Erlangen, with research stays at University College London and Bell Labs (U.S.A.). His research on options on capacity has been presented at international conferences and leading US business schools. Moreover, his research was awarded a number of prizes, most notably the Management Science Strategic Innovation Award (from EURO) as well as the GOR dissertation award. Upon the completion of his doctoral studies, Prof. Spinler spent a year as a lecturer at the Wharton School, where he taught classes in the M.B.A. and Ph.D. programs and developed a real options class for executives. He has been invited to teach the real options class at MIT as a guest professor in their Leaders for Manufacturing Program. He was awarded the best guest lecturer award twice at HHL. His postdoctoral degree (Habilitation) covered aspects of market-based supply chain coordination and was completed in September 2008. In January 2009, Prof. Spinler was appointed to the Chair of Logistics Management at WHU.