Abstract
In this work, we study the multi-product dynamic lot-sizing problem with capacity constraints and batch ordering. This problem arises in short to medium range production scheduling for several products over a finite number of periods to meet known demand. Each period has a capacity for placing orders, and every order for each product must have a fixed quantity, or batch size, though multiple orders can be placed for each product. We define three mixed-integer linear programming (MILP) models and apply Lagrangian relaxation to formulate the corresponding dual problems by relaxing the capacity constraints. The aim is to identify the dual problem that is the easiest to solve and provides the solution with the smallest duality gap. Subgradient optimisation is applied to solve the preferred Lagrangian dual model, which uses one of two heuristics to find good feasible solutions. We also show that the special case, where the batch sizes for all products are the same, can be modeled as a transportation problem. A set of numerical experiments is designed to compare the performance of the Lagrangian relaxation approach with a commercial MILP solver to identify the version of the subgradient algorithm and the MILP model that provide the best solutions.
Acknowledgements
This work was supported by the College of Engineering ROCKET Seed Grant Program of Penn State University.
Disclosure statement
No potential conflict of interest was reported by the author(s).
Data availability statement
The data that support the findings of this study are available from the first author, KAB, upon reasonable request.
Additional information
Funding
Notes on contributors
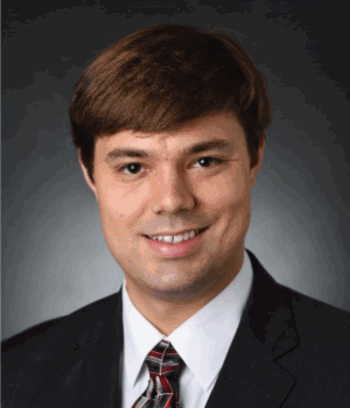
Kevin A. Bunn
Kevin A. Bunn received his dual Ph.D. degree in Industrial Engineering and Operations Research from the Pennsylvania State University in 2021. His research is mainly focused on supply chain optimisation. Currently, he works as a Research Scientist at Amazon in Seattle, WA, where he focuses on optimising Amazon’s warehouse operations.
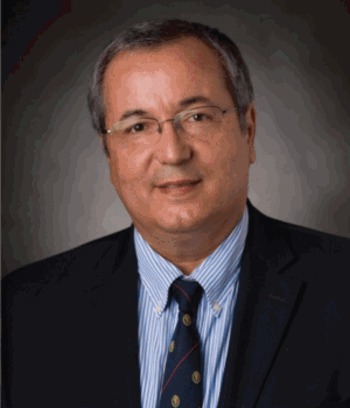
José A. Ventura
José A. Ventura received his Ph.D. degree in Industrial and Systems Engineering from the University of Florida in 1986. He is currently a Distinguished Professor of Industrial Engineering and Chair of the Interdisciplinary Operations Research Graduate Program at the Pennsylvania State University. He is a Fellow of IISE, the recipient of the 2018 David F. Baker Distinguished Research Award from IISE, and an Associate Editor of AIMS Environmental Science and Energies. His research interests include supply chain engineering, production management, transportation networks, and energy.