Abstract
Assemble-to-order approaches deal with randomness in demand for end items by producing components under uncertainty, but assembling them only after demand is observed. Such planning problems can be tackled by stochastic programming, but true multistage models are computationally challenging and only a few studies apply them to production planning. Solutions based on two-stage models are often short-sighted and unable to effectively deal with non-stationary demand. A further complication may be the scarcity of available data, especially in the case of correlated and seasonal demand. In this paper, we compare different scenario tree structures. In particular, we enrich a two-stage formulation by introducing a piecewise linear approximation of the value of the terminal inventory, to mitigate the two-stage myopic behaviour. We compare the out-of-sample performance of the resulting models by rolling horizon simulations, within a data-driven setting, characterised by seasonality, bimodality, and correlations in the distribution of end item demand. Computational experiments suggest the potential benefit of adding a terminal value function and illustrate interesting patterns arising from demand correlations and the level of available capacity. The proposed approach can provide support to typical MRP/ERP systems, when a two-level approach is pursued, based on master production and final assembly scheduling.
Disclosure statement
No potential conflict of interest was reported by the author(s).
Data availability statement
The open-source code is available at https://github.com/DanieleGioia/ATO, to properly guarantee reproducibility and to enable interested readers to carry out further experiments.
Notes
1 We denote random variables with capital letters and their realisations with lowercase letters.
Additional information
Notes on contributors
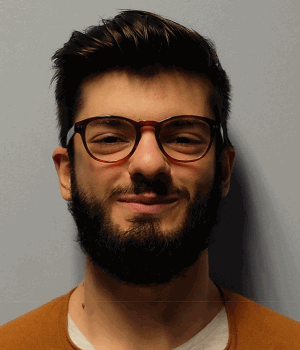
Daniele Giovanni Gioia
Daniele G. Gioia is a Mathematical Engineer pursuing a Ph.D. in dynamic optimisation under uncertainty at Politecnico di Torino. His application context deals with decision-making under uncertainty in engineering/management applications. He previously earned his master's and bachelor's degrees in mathematical engineering at Politecnico di Torino.
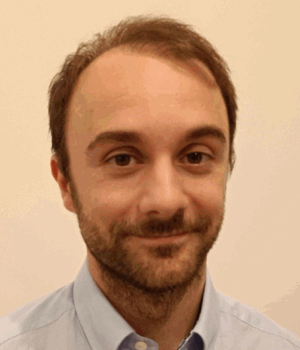
Edoardo Fadda
Edoardo Fadda received a master's degree in mathematical engineering and a Ph.D. in information technology and system engineering from the Politecnico di Torino, Torino, Italy, in 2014 and 2018, respectively. He is an Assistant Professor with the Dipartimento di Scienze Matematiche, Politecnico di Torino. Since 2018 he has been a member of the Isires's Scientific and Technical Committee (Istituto Italiano di Ricerca e Sviluppo). His main interests are optimisation under uncertainty, statistics, and their applications.
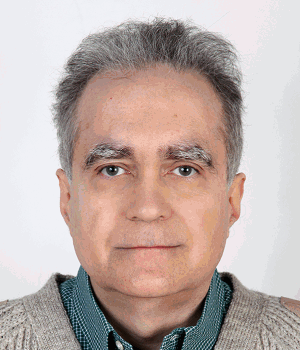
Paolo Brandimarte
Paolo Brandimarte graduated cum laude in electronic engineering in 1987, at Politecnico di Torino, where he spent all of his academic career. He held positions as assistant professor of Control Systems, starting in 1989, and associate professor of Manufacturing Systems. Currently, he is a full professor of Operations Research at the Department of Mathematical Sciences. His main research fields have been non-standard scheduling problems, production planning models, and supply chain and inventory management. In more recent years, he also worked on telecommunications, quantitative finance, marketing analytics, and data-driven control, both in academic research and consulting. From a methodological perspective, his main expertise is in metaheuristics for combinatorial optimisation, matheuristics for integer programming, stochastic programming, and simulation-based optimisation. Besides papers on refereed journals, he has authored or edited fourteen books, including a book on Numerical Methods in Finance that has been translated to Chinese. His most recent book is a booklet on Dynamic Programming and Reinforcement Learning, published within the book series EURO Advanced Tutorials on Operations Research. The URL containing information and material related to his books is https://staff.polito.it/paolo.brandimarte.