ABSTRACT
To maintain high-quality semiconductor wafer production processes, it is necessary to build high-quality virtual metrology (VM) model. Based on the result of the VM, the engineer can monitor and control the specific process. In the manufacturing process, various sources are obtained from sensor equipment. It is important to consider that these source data exhibit varying characteristics during the model construction. However, when all data sources are simply aggregated into a single model, the performance of the overall model may degrade, especially if any of the individual sources contain outliers or noise. To address this issue, we develop a data-fusion model designed to incorporate diverse data sources into a unified multi-source model. In particular, we improve an Adaboost regression algorithm to make it suitable for multi-source data in the field of VM. The algorithm combines the residuals of the models derived from each individual data source and all sources to adaptively adjust the thresholding value, which, in turn, determines whether the predicted values are accurate or not for each weak learner. Extensive practical validations on real-world processing data from semiconductor manufacturers have demonstrated that the proposed method outperforms single learning algorithms and is more robust than all benchmarks.
Disclosure statement
No potential conflict of interest was reported by the author(s).
Data availability statement
The data that support the findings of this study are available from the corresponding author upon reasonable request.
Additional information
Funding
Notes on contributors
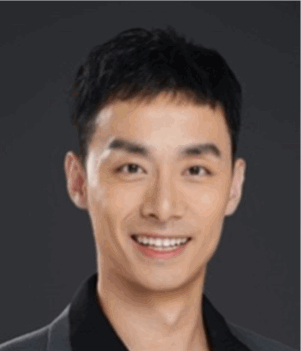
Yifan Xie
Yifan Xie received the B.S. degrees in statistics from Zhongnan University of Economics and Law, Wuhan, China and University of Glasgow, Glasgow, United Kingdom in 2015 and M.S. degrees in applied urban science and informatics from New York University, New York, NY, in 2016, economics and finance from Pompeu Fabra University, Barcelona, Spain, in 2018, and management research from Rutgers University, Newark, NJ, in 2020. He is currently a doctoral candidate in industrial and systems engineering at Rutgers University, New Brunswick, NJ. In addition to research, his is interested in the areas of machine learning algorithms, kernel method and data mining.
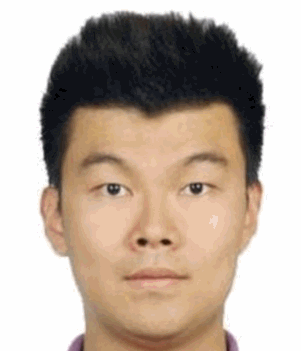
Tianhui Wang
Tianhui Wang received the B.S. degree in transportation engineering from Tongji University, Shanghai, China in 2014. He is currently a doctoral candidate in industrial and systems engineering at Rutgers University, New Brunswick, NJ. His research interests include data mining, optimisation methods in machine learning.
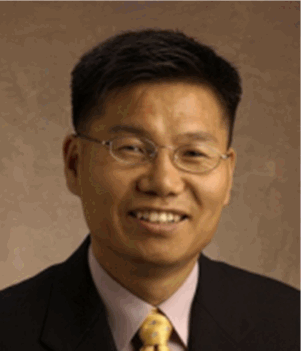
Myong K. Jeong
Myong K. Jeong Dr. is a Professor with the Department of Industrial and Systems Engineering and the Rutgers Center for Operations Research, Rutgers University. He has focussed on developing data mining techniques, process monitoring and control procedures, and optimisation techniques for machine learning.
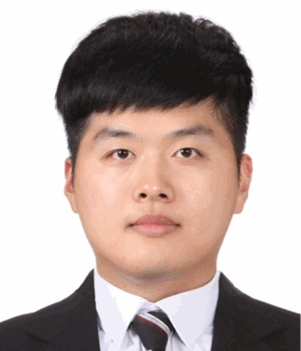
Gyeong Taek Lee
Gyong Taek Dr. Lee is an Assistant Professor with the Department of Mechanical, Smart, and Industrial Engineering from Gachon University, Seongnam, South Korea. He received the Ph.D. degree in industrial engineering from Yonsei University, South Korea, in 2022. He was a Visiting Researcher with the Department of Industrial and Systems Engineering, Rutgers University, The State University of New Jersey, Piscataway, NJ, USA. His current research interests include reinforcement learning and manufacturing data science.