Abstract
Heating, ventilation, and air conditioning (HVAC) systems play a crucial role in production environments. Optimising the control strategy of an HVAC system is an effective approach to achieving energy savings and reducing emissions in a production environment. In existing optimal control models for HVAC systems, occupant behaviour has been incorporated as a key influencing factor. However, model predictive control algorithms have not introduced to examine its performance in optimising HVAC system operation. An optimisation strategy considering occupant (leaders and users of HVAC systems) behaviour (OSOB) is proposed based on model predictive control algorithm. The concept of environmentally specific transformational leadership is introduced to characterise the behaviours of leaders. Then the distinct decisions driven by double objectives (carbon emissions and electricity costs) can be explained. The OSOB was applied to a representative office building in Beijing. The findings indicate that incorporating occupant behaviour into algorithm can lead to a significant reduction in carbon emissions, up to 45%, as well as a decrease in electricity bills, up to 17%. This study not only incorporates occupant behaviour as a significant influencing factor, but also offers valuable insights for product manufacturers seeking to reduce the carbon emissions produced during building operations.
Disclosure statement
No potential conflict of interest was reported by the author(s).
Data availability statement
The data that support the findings of this study are available from the corresponding author upon reasonable request.
Additional information
Funding
Notes on contributors
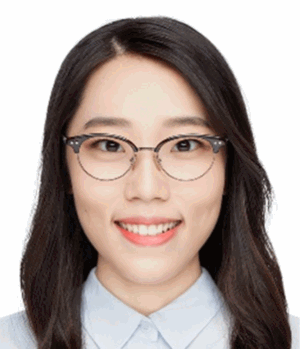
Meng Shen
Meng Shen is an associate professor of School of Management and Economics, Beijing Institute of Technology, China. She worked as a research fellow in National University of Singapore after she got her Ph.D. degree in Public Administration from Tianjin University, China. She was formerly a joint Ph.D. at University of Maryland, College Park. Her research focuses on green buildings, smart facility management and energy conservation behaviour in buildings.
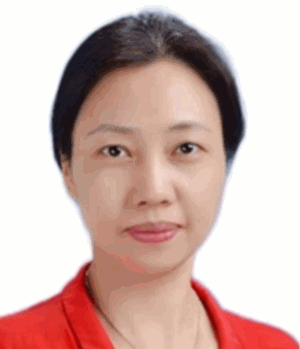
Baojun Tang
Baojun Tang is a Distinguished Professor of School of Management and Economics, Beijing Institute of Technology, China. She is Deputy Director of the Centre for Energy and Environmental Policy Research. She has presided over more than 30 scientific research projects, and has published more than 100 papers and 9 monographs. She has won a total of 8 provincial and ministerial awards including the first prize, 17 authorised patents and 28 software copyrights.
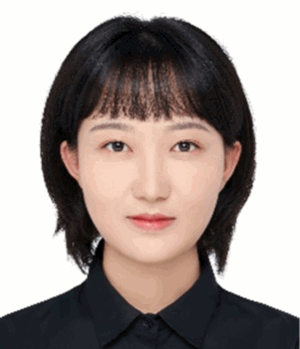
Keai Zhang
Keai Zhang is a master student in School of Management and Economics, Beijing Institute of Technology, China. Her main research interests include Building energy efficiency and intelligent energy management.