Abstract
This paper presents a new robust convergence condition of iterative learning control with initial state learning (ILC-ISL) for linear multivariable discrete-time systems in the presence of iteration-varying uncertainty. This method is based on linear matrix inequality (LMI) and provides fixed learning gains during time and iteration. Since the convergence of the ILC algorithm may change due to uncertainty in the parameters of a system, and the ILC algorithm is incapable of dealing with iteration-related challenges, it is a major challenge to reject the effect of iteration varying uncertainty. Besides, in the basic ILC algorithm, the initial state is constant in each iteration and consequently always leads to a tracking error. In this paper, first, a convergence condition of the ILC-ISL algorithm is designed based on closed-loop system stability in the iteration domain, and second, a new robust convergence condition is achieved by the LMI approach. Finally, the effectiveness of the proposed robust convergence scheme is evaluated through a numerical example and a mechanical system, respectively.
Acknowledgments
The authors would like to thank the editors and the referees for carefully reading the paper and for their comments which have helped to greatly improve the paper.
Data availability statement (DAS)
Data sharing is not applicable to this article as no new data were created or analysed in this study.
Disclosure statement
No potential conflict of interest was reported by the author(s).
Notes
1 Unscented Kalman Filter
2 Sum of Squares Error
3 Sum of Absolute Error
Additional information
Notes on contributors

Mojtaba Ayatinia
Mojtaba Ayatinia was born in Khuzestan, Iran, in 1986. He received the B.E. degree in electrical engineering from the Islamic Azad University (Dezful-Br.), in, Dezful, Iran, in 2009, and the M.sc in Control systems for the Islamic Azad University (Borujerd-Br.), in, Borujerd, Iran, in 2015. Since 2015, He is a student of control and systems engineering Ph.D. of Electrical Engineering Department, Islamic Azad University (Ahvaz-Br.), Iran. His main areas of research interest are Iterative Learning Control, Artificial Intelligence, Model-Based Predictive Control Systems and Chaotic systems theory and applications.
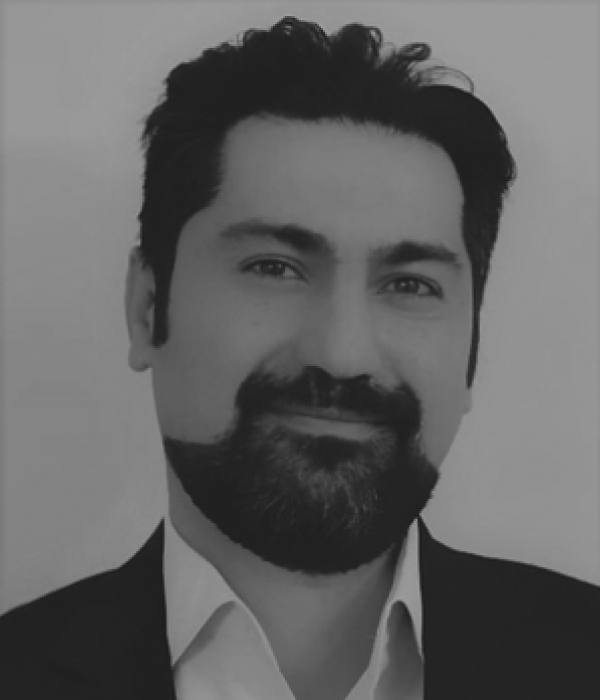
Mehdi Forouzanfar
Mehdi Forouzanfar received the M.Sc. and Ph.D. degree in control-electrical engineering from the Amirkabir Industrial University, Tehran, Iran, in 2009, and from the Sahand Industrial University, Tabriz, Iran, in 2017, respectively. Since 2017, He is a faculty member of Electrical Engineering Department, Islamic Azad University (Ahvaz-Br.), Iran. His main areas of research interest are Active Fault Detection and FTC, Optimal Control, Identification, Artificial Intelligence, and Cyber Physical System.
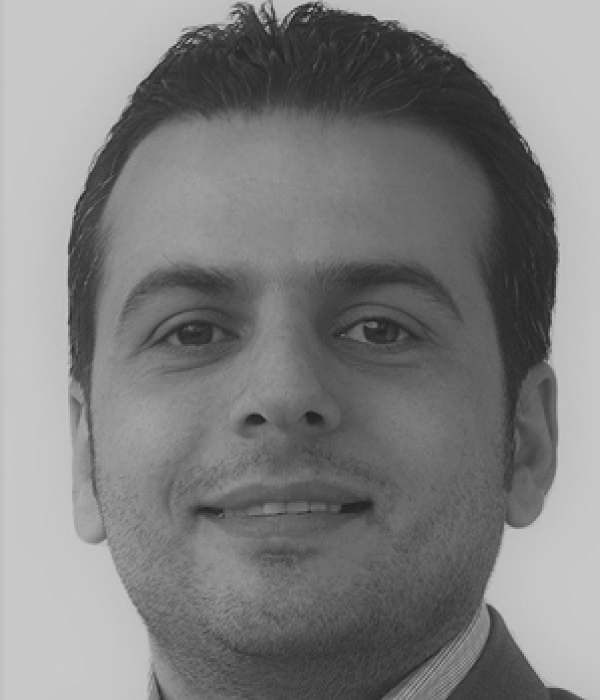
Amin Ramezani
Amin Ramezani (IEEE Member 2005) was born in Khuzestan, Iran, in 1979. He received the B.E. degree in electrical engineering from the Shahid Beheshti University of Tehran, Tehran, Iran, in 2001, and the M.sc in Control systems for Sharif University of Technology, Tehran, Iran, in 2004 and Ph.D. degrees in electrical engineering from the University of Tehran, Tehran, Iran, in 2011. In 2012, he joined the Department of Electrical Engineering, Tarbiat Modares University, Tehran, Iran, as a Lecturer. He is the chair of Advanced Control Lab and cooperates as the PC members of some conferences and special editor and reviewer of international journals in the field of control systems. His current research interests include Fault Tolerant Control Systems, Model-Based Predictive Control Systems, Stochastic Control Systems, Hybrid Systems. Dr. Ramezani is a member of IEEE since 2005 and a member of Iranian Community Instrument and Control Engineers since 2012. He has contributed in more than 100 journal papers and 200 conference papers.