Abstract
Behavioral cloning from observation (BCO) allows the robot to learn the policy without the expert's action information. However, it requires a few interactions with the environment to infer expert's action with risk of robot failures. In addition, BCO assumes that the inferred action is of accurate, causing wrong and inefficient updates of the policy. Both problems can be resolved by outlier detection whether the faced state is experienced or not. This paper addresses such outlier detection mechanisms using variational autoencoder (VAE) to improve safety and efficiency of the standard BCO. For the first safety problem, we suppose that the expert's demonstrations only visited the safe states, and then, VAE is learned by the expert's state data to detect inexperienced and dangerous scenes. For the second efficiency problem, another VAE is trained with the state data safely collected by the imitator's policy to detect the scenes where the inferred actions are not accurate. In handwriting robot experiments, the proposed mechanisms succeeded in improving the standard BCO in terms of both the safety (roughly 64%) and the efficiency (roughly 44%). The high versatility of the proposed mechanisms is verified from learning various alphabets.
GRAPHICAL ABSTRACT
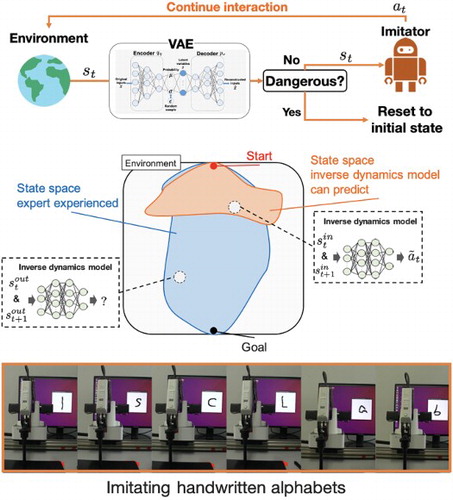
Disclosure statement
No potential conflict of interest was reported by the authors.
Additional information
Notes on contributors
Hidehito Fujiishi
Hidehito Fujiishi received his B.E. in Hiroshima City University, Hiroshima, Japan, in 2019 and his M.E. in Nara Institute of Science and Technology, Nara, Japan, in 2021. His research interests are imitation learning with deep neural networks.
Taisuke Kobayashi
Taisuke Kobayashi received his B.E., M.E., and Ph.D. degrees from Nagoya University, Aichi, Japan, in 2012, 2014, and 2016, respectively. From 2018 to 2019, he was a visiting scholar of the Technical University of Munich, Munich, Germany. Since 2016, He is an assistant professor of the Nara Institute of Science and Technology, Nara, Japan. Since 2020, he is also a JST PRESTO researcher. His research interests include the locomotion control by intelligent systems and autonomous robotics with reinforcement learning.
Kenji Sugimoto
Kenji Sugimoto received the M.S. and Ph.D. degrees from Kyoto University in 1982 and 1989, respectively. After working with Mitsubishi Electric Corporation, he became an assistant professor with Kyoto University in 1985. Then he became an associate professor with Okayama University and Nagoya University. Since 1999, he has been a professor with the Nara Institute of Science and Technology. His current research interests include control theory and system science. Prof. Sugimoto is a member of IEEE, SICE, and ISCIE.