Abstract
Multimodal learning is a framework for building models that make predictions based on different types of modalities. Important challenges in multimodal learning are the inference of shared representations from arbitrary modalities and cross-modal generation via these representations; however, achieving this requires taking the heterogeneous nature of multimodal data into account. In recent years, deep generative models, i.e. generative models in which distributions are parameterized by deep neural networks, have attracted much attention, especially variational autoencoders, which are suitable for accomplishing the above challenges because they can consider heterogeneity and infer good representations of data. Therefore, various multimodal generative models based on variational autoencoders, called multimodal deep generative models, have been proposed in recent years. In this paper, we provide a categorized survey of studies on multimodal deep generative models.
GRAPHICAL ABSTRACT
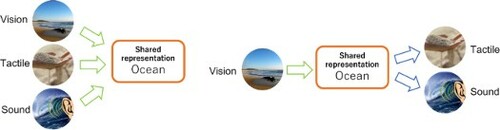
Disclosure statement
No potential conflict of interest was reported by the author(s).
Notes
1 means a subset of multimodal data different from
.
2 In the original text, a shared representation is called a joint representation; we have changed this terminology to match that in this paper. Strictly speaking, there is a subtle difference between shared representation and joint representation: the former refers to a space shared by different modalities while the latter refers to a shared representation of the joint model (see Section 5), i.e. a space in which different modalities are fused.
3 In this paper, we use the term ‘encoder’ to refer to any form of mapping from an input space to a latent space, whether deterministic or probabilistic, and ‘inference distribution’ to refer specifically to the conditional distribution .
4 Note that the inference distributions are the same when the outputs of the encoder networks (i.e. the parameters of the inference distributions) are the same, not necessarily when the values of the training parameters of these networks are all the same.
5 The 2-Wasserstein distance is the p-Wasserstein distance of order p = 2.
6 In the original paper [Citation66], JVAE and JMVAE are referred to as JMVAE and JMVAE-kl, respectively. However, because some papers refer to JMVAE-kl as JMVAE [Citation65,Citation67], we follow them to avoid confusion in terminology.
7 Recall that deep generative models are generative models whose distributions are parameterized by deep neural networks; therefore, if these models are large, their implementation can be much more complex than the implementation of regular generative models.
Additional information
Funding
Notes on contributors
Masahiro Suzuki
Masahiro Suzuki is a project assistant professor in the Graduate School of Engineering at the University of Tokyo. He was formerly a project researcher at the University of Tokyo from 2018 to 2020. He received his PhD from the University of Tokyo in 2018 and his MS degree from Hokkaido University in 2015. His research interests are in deep generative models, multimodal learning, and transfer learning.
Yutaka Matsuo
Yutaka Matsuo is a professor at the Graduate School of Engineering, the University of Tokyo. He received his BS, MS, and PhD degrees from the University of Tokyo in 1997, 1999, and 2002. After working at the National Institute of Advanced Industrial Science and Technology (AIST) and Stanford University, he joined the faculty of University of Tokyo in 2007. He served as Editor-in-chief from 2012 to 2014, and as the chair of the ELSI committee from 2014 to 2018 at Japan Society for Artificial Intelligence (JSAI). He is the president of the Japanese Deep Learning Association (JDLA), and a member of the board of directors at SoftBank Group Corp. He is working on artificial intelligence, especially on deep learning and web mining.