Abstract
Concentrations of ambient particulate matter (PM) depend on various factors including emissions of primary pollutants, meteorology and chemical transformations. New Delhi, India is the most polluted megacity in the world and routinely experiences extreme pollution episodes. As part of the Delhi Aerosol Supersite study, we measured online continuous PM1 (particulate matter of size less than 1 μm) concentrations and composition for over five years starting January 2017, using an Aerosol Chemical Speciation Monitor (ACSM). Here, we describe the development and application of machine learning models using random forest regression to estimate the concentrations, composition, sources and dynamics of PM in Delhi. These models estimate PM1 species concentrations based on meteorological parameters including ambient temperature, relative humidity, planetary boundary layer height, wind speed, wind direction, precipitation, agricultural burning fire counts, solar radiation and cloud cover. We used hour of day, day of week and month of year as proxies for time-dependent emissions (e.g., emissions from traffic during rush hours). We demonstrate the applicability of these models to capture temporal variability of the PM1 species, to understand the influence of individual factors via sensitivity analyses, and to separate impacts of the COVID-19 lockdowns and associated activity restrictions from impacts of other factors. Our models provide new insights into the factors influencing ambient PM1 in New Delhi, India, demonstrating the power of machine learning models in atmospheric science applications.
Copyright © 2023 American Association for Aerosol Research
Graphical Abstract
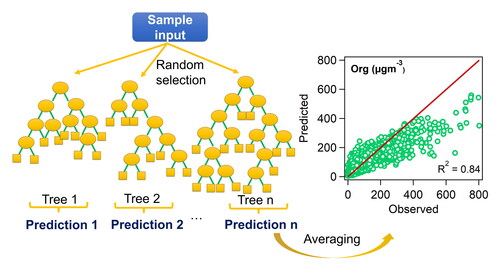
Editor:
Acknowledgements
We thank Dr. Ann Marie Carlton for fruitful discussions about MODIS cloud data and Dr. Barron Henderson for providing the scripts to extract MODIS cloud data.
Disclosure statement
No potential conflict of interest was reported by the authors.