Abstract
Lung cancer is the most common cancer causing the highest mortality rate as per the analysis of previous and recent year worldwide statistics. The main reason behind the high mortality is late detection of its fatal stage. Early detection of lung nodules offers challenging tasks due to nodules’ types, size, unfavorable locations, and attachment with the vessels. In this paper, we propose a method of early detection of lung nodules in computed tomography images, which can assist radiologists to locate the suspected nodules. The method consists of three major sections, i.e. the extraction and segmentation of lung parenchyma through clustering algorithm, extraction and highlighting the region of interests through optimal multilevel thresholding using particle swarm optimization, selection and application of 2D features to detect the initial nodule candidates and further iteratively false positive reduction by the analysis of 3D features. We used 84 CT scans with 301 nodules taken from publically available Lung Image Database Consortium – Image Database Resource Initiative (LIDC-IDRI) data set for experiment. In general for all types of nodules, the proposed method achieved an overall sensitivity of 84.05% with a reduced false positive rate of 1.93 per CT scan. Our results have been compared with the methods from literature which show improved false positive rate along with comparable sensitivity.
Additional information
Notes on contributors
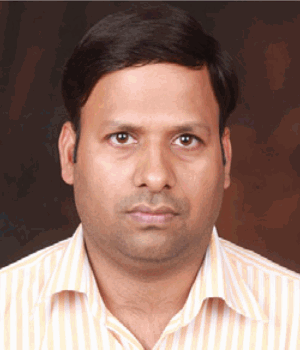
Satya Prakash Sahu
Satya Prakash Sahu received the BE and MTech degrees in computer science and engineering from the Rajiv Gandhi Technological University, Bhopal, India, and the PhD degree in information technology from the National Institute of Technology Raipur, India. He is an assistant professor in the Department of Information Technology, NIT Raipur. His research of interest includes artificial intelligence, machine learning, image processing, medical imaging, and soft computing. He has authored more than 13 research papers in national and international conferences and journals. Corresponding author. Email: [email protected]
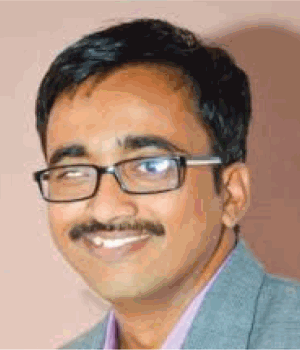
Narendra D Londhe
Narendra D Londhe received the BE degree from Amravati University, MTech and PhD degrees in electrical engineering, IIT Roorkee, He is an assistant professor with the Department of Electrical Engineering, National Institute of Technology Raipur. His research area includes medical signal and image processing, machine learning, pattern recognition, and medical ultrasound. He has authored over 50 research papers in the aforementioned areas. He has also served as a Reviewer of several journals. Email: [email protected].
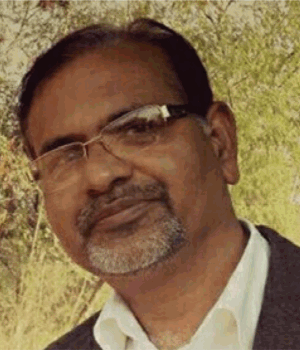
Shrish Verma
Shrish Verma received the master’s degree in electronics and electrical communication engineering from the IIT Kharagpur, with a focus on computer engineering, and the PhD degree in engineering from Pt. Ravi Shankar Shukla University, Raipur, India. He is currently a professor with the Department of Electronics and Telecom Engineering, NIT Raipur. His current research interests include digital system design, data mining and its applications, and image processing. He has authored over 50 research papers in various journals and conferences. Email: [email protected].