ABSTRACT
Co-operative co-evolution approach solves problems by breaking them into subproblems. The proposed framework for Clinical Decision Support System (CDSS) uses a co-operative coevolution approach which treats Feature Selection (FS) and Instance Selection (IS) as independent subproblems. FS and IS remove less relevant features and instances, respectively, thereby improving the overall performance of the system. In this work, both Feature and Instance selection are done using the wrapper approach, which uses co-operative co-evolution and random forest classifier. The reduced dataset is used to train a random forest classifier and this trained model helped in making clinical decisions. These decisions assist physicians as the second opinion for diagnosis and treatment. Wisconsin Diagnostic Breast Cancer (WDBC), Hepatitis, Pima Indian Diabetes (PID), Cleveland Heart Disease (CHD), Statlog Heart Disease (SHD), Vertebral Column, and Hepatocellular Carcinoma (HCC) datasets from the University of California Irvine (UCI) Machine Learning repository are used for experimentation. The proposed framework achieved an accuracy of 97.1%, 82.3%, 81.01%, 93.4%, 96.8%, 91.4%, and 72.2% for datasets WDBC, Hepatitis, PID, CHD, SHD, vertebral column, and HCC, respectively. The results prove that the CDSS-developed using co-operative coevolution can efficiently assist the physicians in decision-making.
ACKNOWLEDGEMENT
The authors thank Vishveshvaraya Ph.D. Scheme for Electronics and IT, Deity, Ministry of Communications and IT, Government of India's fellowship grant trough Anna University, Chennai for the financial support of the research work.
DISCLOSURE STATEMENT
No potential conflict of interest was reported by the authors.
Additional information
Notes on contributors
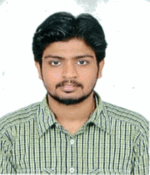
V. R. Elgin Christo
V R Elgin Christo completed BTech (Information Technology) (2013) and MTech (Information Technology) (2015) from Anna University, Chennai, Tamil Nadu, India. Currently he is pursuing PhD (Computer Science and Engineering), in Anna University, Chennai, Tamil Nadu, India. He is a research scholar under “Visvesvaraya PhD Scheme for Electronics and Information Technology”, Deity, Ministry of Communication and Information Technology, Government of India. His areas of interest include bio-inspired computing, medical image processing and data mining. E-mail: [email protected]
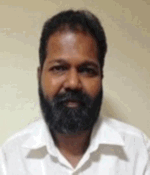
H. Khanna Nehemiah
Khanna Nehemiah H completed BE (Computer Science and Engineering) (1997), ME (Computer Science and Engineering) (1998) from University of Madras, Chennai, Tamil Nadu, India and PhD (Computer Science and Engineering) (2007) from Anna University, Chennai, Tamil Nadu, India. He is currently working as a professor in Ramanujan Computing Centre, Anna University, Chennai, Tamil Nadu, India. His areas of interest include software engineering, database systems, data mining, medical image processing, artificial intelligence, soft computing and bio-inspired computing.
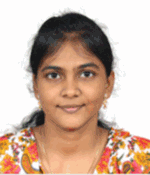
J. Brighty
Brighty J completed BE (Computer Science and Engineering) (2015) and ME (Computer Science and Engineering) (2017) from Anna University, Chennai, Tamil Nadu, India. Her areas of interest include bio-inspired computing and data mining. E-mail: [email protected]
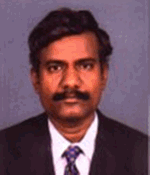
Arputharaj Kannan
Kannan Arputharaj completed ME (Computer Science and Engineering) (1991) and PhD (Computer Science and Engineering) (2000) from Anna University, Chennai, Tamil Nadu, India. He retired as a professor from the Department of Information Science and Technology, Anna University, Chennai, Tamil Nadu, India. He is currently working as a senior professor in Vellore Institute of Technology, Vellore. His areas of interest include database management systems, artificial intelligence, bio-inspired computing and information security. E-mail: [email protected]