ABSTRACT
This paper presents modification of standard Kalman filter (KF) based on augmented input estimation (AIE) and deadbeat dissipative FIR filtering (DDFF) for maneuvering target tracking. Although KF is a well-known tool in estimation and tracking but it has two weaknesses, disability in maneuvering motions and lack of robustness against temporary model uncertainty. For the first problem, the AIE is proposed to cover both the non-maneuvering and maneuvering parts of motion and so the maneuver detection procedure is eliminated. This model with an input estimation (IE) approach is a special augmentation in the state space model which considers both the state vector and the unknown input vector as a new augmented state vector. Also, KF is based on infinite impulse response (IIR) structure and cannot be robust against temporary model uncertainty. Unlike IIR, finite impulse response (FIR) filter is robust and stable against model uncertainty. So, for the second problem, an extra type of FIR filter named DDFF is introduced in this paper that not only has the intrinsic properties of FIR but also can improve these features by tuning some weighting parameters. In the last examples of the paper, the advantages of the proposed model against other models are shown.
Additional information
Notes on contributors
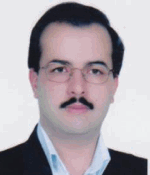
Mahmoud Reza Hadaegh
Mahmoud R Hadaegh received the BSc degree in electronics engineering from Shiraz University, Shiraz, Iran, in 1999, the MSc degree in control engineering from K N Toosi University of Technology, Tehran, in 2001 and PhD degree in control engineering from Science and Research Branch, Islamic Azad University, Tehran, in 2018. Now he is a lecturer in Islamic Azad University and his interest area is in target tracking for single and multiple targets and wireless sensor networks. Email: [email protected]
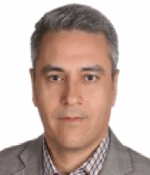
Hamid Khaloozadeh
Hamid Khaloozadeh received the BSc degree in control engineering from Sharif University of Technology, Tehran, Iran, in 1990, the MSc degree in control engineering from K N Toosi University of Technology, Tehran, in 1993, and the PhD degree in control engineering from Tarbiat Modarres University, Tehran, in 1998. He is currently a professor with the Department of Systems and Control, Faculty of Electrical Engineering at the K N Toosi University of Technology. He is director of the Industrial Control Center of Excellence (ICCE), at K N Toosi University of Technology. His interest area is stochastic estimation and control, system identification, optimal control, and time series analysis.
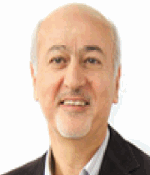
Mohammad Taghi Hamidi Beheshti
Mohammad T H Beheshti received his BS in electrical engineering from the University of Nebraska at Lincoln in 1984, his MS and PhD in control engineering from the Wichita State University, Wichita Kansas, in 1987 and 1992, respectively. Dr Beheshti is an associate professor of the ECE Department, Tarbiat Modares University, Tehran, Iran since 1995. Dr Beheshti’s research interest are in modeling and control of singular perturbation systems, modeling and control of communication and computer networks, NCS and microgrid systems. Email: [email protected]