Abstract
Mining frequent patterns with single minimum support is quite unpredictable as it may miss rare itemsets depending upon the high value of minimum threshold. The phenomenon is commonly known as “rare item problem” dilemma. In this connection, frequent pattern mining under multiple minimum supports (MMS) is considered as an adequate solution of the problem. Existing approaches in this domain require user-defined minimum item support (MIS), and consider only the support in recognizing the importance of the items and ignore other factors including the inter-item relationships. The generation of huge number of itemsets including the itemsets with low associability or high dissociation is another problem in the existing MMS-based approaches. Keeping view of the above issues, this paper introduces AutoMMS-FPM, an automated MMS specification approach for frequent pattern mining with partial devoid of dissociation. The proposed approach not only takes account of support but also some other factors such as influence (inf), dissociation (d) and length importance factor (ρ) to boost up the mining process. Influence refers to the inter-item relationship and it is formulated based on the concept of degree centrality of the corresponding item network. MIS for the items are calculated automatically using MBS and RIF factors where the first one relates to the item support and the second one relates to the item influence. Experimental results on both of the synthetic and real datasets show that the proposed algorithm outperforms than existing approaches in terms of number of generated itemsets, run time, memory usages, dissociation and scalability.
ACKNOWLEDGEMENT
This research is partially supported by the DSTPURSE II program, University of Kalyani, West Bengal, India.
Additional information
Notes on contributors
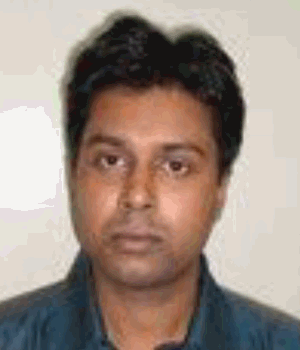
S. Datta
S Datta received the BE degree in computer science & engineering from University of North Bengal, Siliguri, India in 2004 and the MTech degree from Jadavpur University, Kolkata, India in 2011. He is currently a PhD research scholar in the Department of Computer Science & Engineering, University of Kalyani, Nadia, India. He is also serving as an assistant professor in the Department of Computer Science & Engineering, Neotia Institute of Technology, Management & Science, Diamond Harbour Road, West Bengal, India. His research interests include data mining, machine learning, and business intelligence.
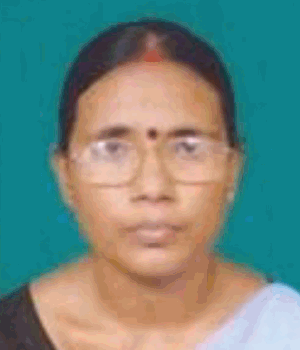
K. Mali
K Mali received both BTech and MTech degrees from Calcutta University, Kolkata, India in 1987 and 1989, respectively. She received PhD degree in computer science & engineering from Jadavpur University, Kolkata, India, in 2005. She is currently a professor in the Department of Computer Science & Engineering, University of Kalyani, Nadia, India. Her research interests include data mining, pattern recognition, image processing and soft computing. E-mail: [email protected].
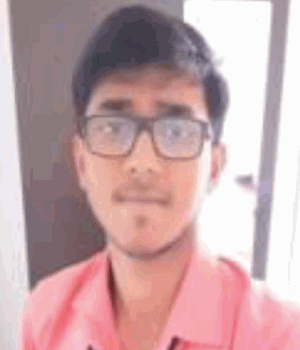
S. Ghosh
S Ghosh received BTech degree from Neotia Institute of Technology, Management and Science, Diamond Harbour, West Bengal affiliated to Maulana Abul Kalam Azad University of Technology (MAKAUT), Kolkata, West Bengal, India in the year 2019. He is currently associated with Tata Consultancy Services, Bangalore, Karnataka, India as an assistant system engineer. His research of interest includes pattern mining, association rule mining, and soft computing. E-mail: [email protected].