Abstract
A framework for Computer-Aided Diagnosis (CAD) to diagnose Cavitary TB and Miliary TB from chest Computed Tomography (CT) slices has been designed and implemented. The lung tissues from the CT slices are segmented using region-based Active Contour Model (ACM) and the Region of Interests (ROIs) labelled by an expert radiologist are extracted. Features based on shape and texture are extracted from each ROI. A wrapper-based Improved Artificial Bee Colony Optimization (I-ABCO) algorithm with the accuracy of the Support Vector Machine (SVM) classifier as the fitness function is used to select the optimal subset of features. The search process of I-ABCO is improved using two evaluation functions, namely, rough dependency measure (RDM) and mutual information (MI), to promote better exploitation of the search space. The selected features are used to train the Radial Basis Function Neural Network (RBFNN) classifier, using ten-fold cross validation and the performance is evaluated. Experimentation has been performed on CT slices using two datasets: Tuberculosis dataset and Lung Image Database Consortium-Image Database Resource Initiative (LIDC-IDRI) dataset. The accuracy of the CAD systems using I-ABCO with MI and I-ABCO with RDM for both datasets are (88.34%, 92.63%) and (87.32%, 90.17%), respectively. The CAD system using the basic Artificial Bee Colony Optimization (ABCO) algorithm for feature selection is also experimented with the same datasets and an accuracy of (85.68%, 88%) is achieved. The performance of the proposed algorithms outperforms the ABCO algorithm in diagnostic accuracy, which ensures that selecting the optimal feature subset efficiently has an impact on the classification accuracy.
Additional information
Notes on contributors
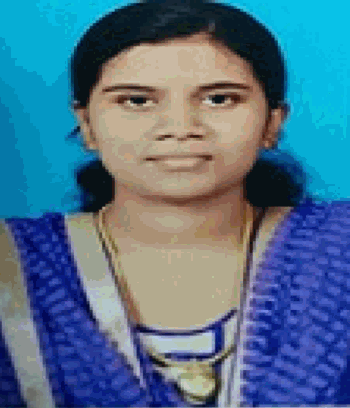
Anisha Isaac
Anisha Isaac completed BE (Computer Science and Engineering) (2012), ME (Computer Science and Engineering) (2014) from Anna University, Chennai, Tamil Nadu, India. She is currently pursuing PhD in medical image processing at Ramanujan Computing Centre, Anna University, Chennai, Tamil Nadu, India. Her areas of interest include medical image processing, bio-inspired computing and data mining. Email: [email protected]

H. Khanna Nehemiah
H Khanna Nehemiah completed BE (Computer Science and Engineering) (1997), ME (Computer Science and Engineering) (1998) from University of Madras, Chennai, Tamil Nadu, India and PhD (Computer Science and Engineering) (2007) from Anna University, Chennai, Tamil Nadu, India. He is currently working as a professor in Ramanujan Computing Centre, Anna University, Chennai, Tamil Nadu, India. His areas of interest include Software Engineering, database systems, data mining, medical image processing, artificial intelligence, soft computing and bio-inspired computing.
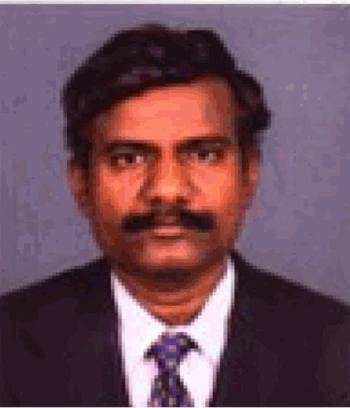
A. Kannan
A Kannan completed ME (Computer Science and Engineering) (1991) and PhD (Computer Science and Engineering) (2000) from Anna University, Chennai, Tamil Nadu, India. He retired as a professor from the Department of Information Science and Technology, Anna University, Chennai, Tamil Nadu, India. He is currently working as a senior professor in Vellore Institute of Technology, Vellore. His areas of interest include database management systems, artificial intelligence, bio-inspired computing and information security. Email: [email protected]