Abstract
Epilepsy is a chronic brain condition affecting one in 100 patients. EEG (Electroencephalogram) is a signal representing the influence of combining various processes in the brain. This paper aims to identify the likelihood levels of epilepsy from the features extracted from the EEG signals and to compare them with the output of the Singular Value Decomposition and Extreme Learning Machine (ELM). The SVD approach extracts functionality from EEG signals (that is, to minimize dimensionality) while the ELM is used as a classification system. A study of twenty patients is documented in this paper. The criteria such as the Performance Index (PI), Sensitivity (Se), Specificity (Sp), Average Detection (AD), and Good Detection Ratio (GDR) are evaluated using ELM to identify epilepsy. Results show that when SVD is classified with ELM at sigmoid activation function, an average detection of 98.94% and a GDR of 97.83% are obtained.
DISCLOSURE STATEMENT
No potential conflict of interest was reported by the author(s).
Additional information
Notes on contributors
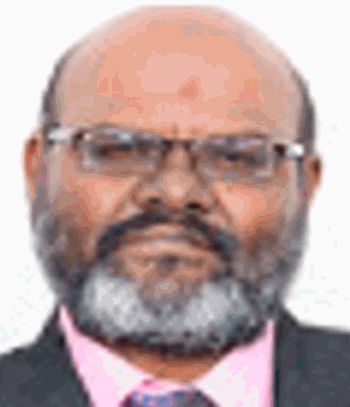
R. Harikumar
R Harikumar received his BE degree in electronics and communication engineering from Regional Engineering College Trichy, India, in 1988; ME degree in applied electronics from College of Engineering, Guindy, Anna University Chennai, India, in 1990, and PhD degree in information and communication engineering from Anna University, India. Presently, he is working as a professor in the Department of Electronics and Communication Engineering at Bannari Amman Institute of Technology, India. His research interests include bio-medical signals processing, soft computing.
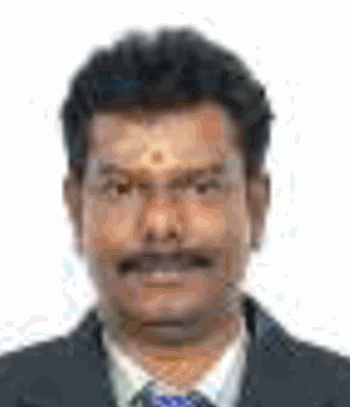
C. Ganesh Babu
C Ganesh Babu received his BE (ECE) degree from PSG College of Technology Coimbatore in 1994. He obtained his ME (Microwave and Optical Engineering) degree from Allagapa Chettiar College of Engineering and Technology, Karaikudi in 1997. He was awarded PhD in I&C Engg from Anna University, Chennai in 2010. He has 22 years of teaching experience. He was assistant professor in electronics and communication engineering at Sri Krishna College of Engineering and Technology, Coimbatore. Currently, he is working as a professor of electronics and communication engineering at Bannari Amman Institute of Technology, Sathyamangalam. Three scholars have completed their doctoral degrees under his guidance and seven scholars are continuing. He has published eighty papers in international and national journals and also published around fifty-seven papers in international and national conferences. His area of interest include signal processing, virtual instrumentation. He is a life member of IEEE, IETE, IET, and ISTE. Email: [email protected]
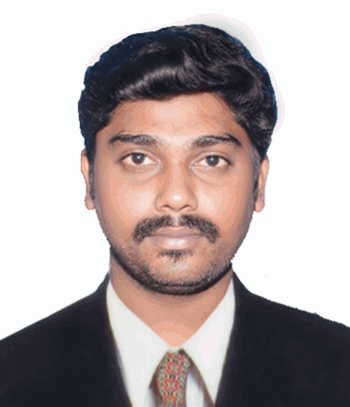
M. Gowri Shankar
M Gowri Shankar received his BE (EEE) degree from Mahendra Engineering College, Tiruchengode in 2012. He obtained his ME (VLSI Design) degree from MIET Engineering College, Trichy in 2014. He has five years of teaching experience. He is pursuing his doctoral research program as a QIP- research scholar (Full Time) in biosignal processing under the guidance of Dr C Ganesh Babu at the Department of Electronics and Communication Engineering, Bannari Amman Institute of Technology Sathyamangalam. His area of interest is bio-signal processing and VLSI design. He is a member of IAENG. Email: [email protected]