Abstract
The majority of conventional alcohol detection approaches are based on traditional machine learning approaches and are unable to retrieve the deeply hidden attributes of alcohol-based electroencephalography (EEG) signals. The intention of this research is to evolve a deep learning-based method for detecting alcohol-related EEG signals automatically. It also investigates whether a deep learning method for alcoholism classification is effective without using any explicit feature extraction and selection steps. To assess this, the paper implements and compares deep learning-based strategies to classify alcohol-related EEG signals. A framework that uses prerecorded raw EEG data as input to a deep learning algorithm called convolutional neural network (CNN), long short-term memory (LSTM), and a cascade model called CNN + LSTM is also implemented for detecting alcoholism on an openly accessible UCI Alcoholic EEG dataset. Some conventional techniques are also implemented on prerecorded raw EEG signals, and the classification results are compared with the proposed methods. The results of the experiments show that the proposed models are capable of achieving average classification accuracies of 99.60%, 98.12%, and 95.95% on the training dataset and 92.77%, 89%, and 91% on the testing dataset along with error rates of 7.5%, 11.90%, and 8.7% from CNN, LSTM, and CNN + LSTM, respectively.
ACKNOWLEDGEMENTS
The authors acknowledge Prof. Henri Begleiter of the Neuro Dynamics Laboratory at the State University of New York Health Center, Brooklyn, USA, who generously shared the data in the public domain. The authors also express their gratitude to the Birla Institute of Technology, Mesra, for providing the required infrastructural and institutional support for the research work.
DISCLOSURE STATEMENT
No potential conflict of interest was reported by the author(s).
Additional information
Notes on contributors
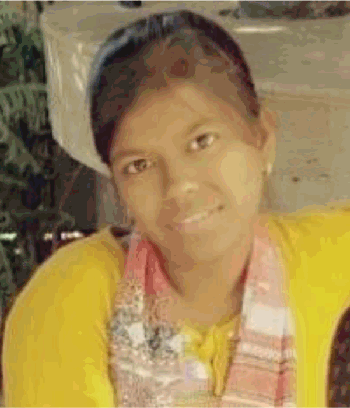
Nandini Kumari
Nandini Kumari attained the integrated MCA degree from Birla Institute Technology, Mesra, India, in 2017. She is currently a research scholar in the Department of Computer Science and Engineering, BIT, Mesra, India. Her current research interests include soft computing, artificial intelligence, and deep learning in signal processing.
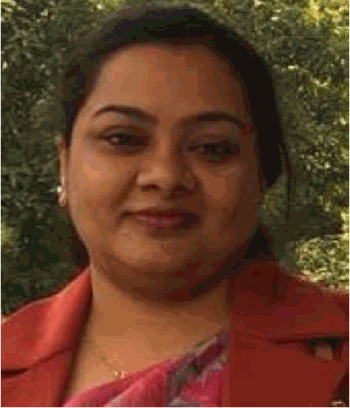
Shamama Anwar
Shamama Anwar is an assistant professor in the Department of Computer Science and Engineering, Birla Institute of Technology, Mesra, India. She acquired the MTech degree from Birla Institute of Technology, Mesra, in 2008, followed by the PhD degree in 2017.
Her research interests include artificial intelligence, soft computing, image and signal processing, cryptography, and information security. Email: [email protected]

Vandana Bhattacharjee
Vandana Bhattacherjee is a professor, Department of Computer Science and Engineering, Birla Institute of Technology, Mesra, Ranchi. She recieved the BE (CSE) degree from Birla Institute Technology, Mesra, India, in 1989 and MTech and PhD degrees in computer science from Jawaharlal Nehru University, New Delhi, India, in 1991 and 1995, respectively. Her research areas include software process models, software cost estimation, software metrics, data mining, and soft computing. E-mail: [email protected]