Abstract
Air pollution has become an environmental threat globally. Therefore, air pollution estimation and prediction are crucial for effecting timely control measures. This paper proposes an ensemble deep particulate forecaster (EDPF) to predict hourly PM2.5 concentrations for a day ahead. Air quality data from Thiruvananthapuram, Kerala (an Indian state), is used as a case study to demonstrate the model. The effect of other pollutant concentrations and various meteorological parameters on PM2.5 prediction is first studied using Pearson correlation analysis and recursive feature elimination method. Thus, the most relevant features for the forecast of PM2.5 concentrations are identified. Then preliminary PM2.5 predictions are made using two base predictors: an attention-based convolutional neural network - bidirectional long short-term memory (CNN-BiLSTM) model and a bidirectional long short-term memory model (BiLSTM). Finally, a feed-forward deep neural network (DNN) model combines the preliminary predictions yielding the final PM2.5 predictions. The proposed model is validated for long-term forecasting of PM2.5 at Thiruvananthapuram, and the model attains root mean squared error of 12.96 µg/m3 and mean absolute error of 9.28 µg/m3 for forecasting 24 h ahead PM2.5 concentrations. The experimental results demonstrate that the model performs better compared to various deep learning models in the literature. The effectiveness of the model in all four seasons is also evaluated, which reveals winter is the most challenging season to forecast.
Acknowledgement
The authors are grateful to the Central Pollution Control Board, India for the data support. The authors would also like to acknowledge the LBS Institute of Technology for Women, Kerala for providing lab facilities, library and infrastructure.
Disclosure statement
No potential conflict of interest was reported by the author(s).
Additional information
Notes on contributors

Anju S. Mohan
Anju S Mohan received BTech (2009) and MTech (2012) degrees in electronics engineering from Cochin University of Science and Technology (CUSAT), Kerala, India. She is a PhD student at the Department of Electronics and Communication engineering, LBS Institute of Technology for Women, Kerala. Her research interest includes deep learning and time-series forecasting.
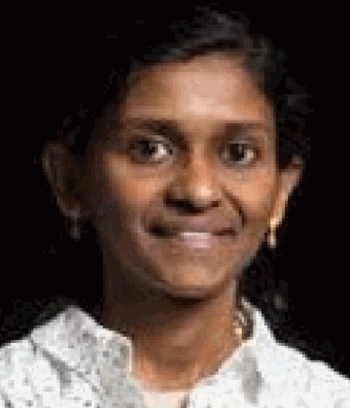
Lizy Abraham
Lizy Abraham is an assistant professor at the LBS Institute of Technology at Kerala Technological University. She received her PhD in electronics & communication engineering with a specialization in signal processing from University of Kerala, India. She did a post-doctoral fellowship in wireless sensor networks at Tyndall National Institute, University College Cork, Ireland. Her research interests include machine learning, wireless sensor networks, and computer vision. E-mail: [email protected]