Abstract
In this manuscript, a deep learning method is implemented for automatic detection of abnormal and normal Electroencephalogram (EEG) signals, especially for early diagnosis of Epileptic Seizure (ES) disease. Firstly, 8th order Butter Worth Filter (BWF) is applied for removing artifacts from Bern Barcelona (BB), Bonn University (BU), and Temple University Hospital (TUH) datasets. Next, the swarm decomposition method is employed for signal decomposition where it provides a significant frequency localization by separating the essential signal components from the denoised EEG signals. Then, the semantic feature extraction is performed for extracting feature values from the decomposed signals. The feature extraction includes the benefits like reduction in overfitting risk, accuracy improvement, and speed up the training process. Further, the extracted multidimensional feature values are reduced by proposing enhanced Salp Swarm Algorithm (SSA), where it selects optimum feature values for classification. The enhanced SSA uses Whale Optimization Algorithm (WOA) for identifying the potential feature values from the multi-dimensional feature space. Lastly, the selected features are fed to the Long Short Term Memory (LSTM) network for classifying abnormality and normality of seizures. The experimental results show that the enhanced SSA based LSTM network classifies abnormality and normality of seizure with classification accuracy of 97.84%, 99.32%, and 99.20% on the BU, BB, and TUH datasets.
Acknowledgements
Author contributions: T. Jhansi Rani: Visualization, Methodology, Implementation, Data Acquisition, Writing – Original Draft, Report Writing. D. Kavitha: Project Administration, Conceptualization, Validation, Formal Report Analysis, Writing – Review & Edit. All authors approved the final manuscript.
Disclosure statement
No potential conflict of interest was reported by the author(s).
DATA AVAILABILITY STATEMENT
The datasets generated during and/or analyzed during the current study are available in the [Bonn, TUH and Bern] repository: Bonn: https://www.ukbonn.de/epileptologie/?idcat=193%26lang=3; TUH: https://isip.piconepress.com/projects/tuh_eeg/ and Bern: https://www.upf.edu/web/mdm-dtic/-/1st-test-dataset?inheritRedirect=true#.YkcLvShBzIU.
Additional information
Notes on contributors
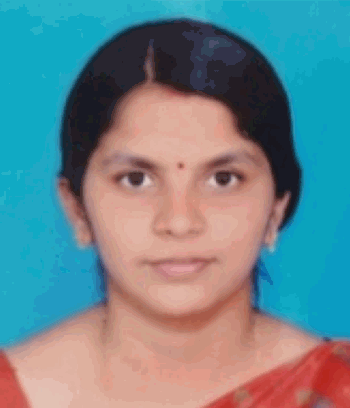
T. Jhansi Rani
T Jhansi Rani completed BTech in CSE Jawaharlal Nehru Technological University Hyderabad, India, MTech in CSE under JNTU-Anantapur, pursing PhD at JNTU-Anantapur in the area healthcare analytics using machine learning. Possesses 12 years of teaching experience.
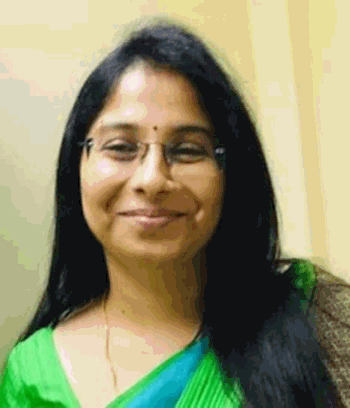
D. Kavitha
D Kavitha obtained PhD in 2012 from Sri Krishna Devaraya University, Anantapuramu, AP, India. Guided 7 PhD scholars. Possesses 20 years of teaching experience. Published over 41 papers in national/international journals and conferences. Executing an AICTE – sponsored project. Email: [email protected].