Abstract
An Artificial Neural Network (ANN) based Substrate Integrated Waveguide Band Pass Filter (SIW BPF) is proposed in this paper. A Feed Forward Back Propagation Neural Network (FF-BP NN) is utilized to optimize the filter dimensions. The Gradient Descent with Momentum and Adaptive Learning Rate algorithm is used to train the network at a learning rate of 0.01. The high pass characteristics of the SIW is converted into band pass by incorporating U slots on its top plane. Defected Ground Structure (DGS) is utilized in the bottom plane to improve the impedance matching. To validate, the prototype is fabricated using RT/Duroid 5880 and tested. The proposed filter has a center frequency at 14.68 GHz with a wide pass band from 12.92 to 16.43 GHz with a 3-dB Fractional Bandwidth (FBW) of 24%, return loss more than 20 dB and insertion loss of about 1.9 dB within the pass band. The filter has a small dimension of about 0.63 , where λg is the guided wavelength at the center frequency. This filter offers wide passband, smaller size, low insertion loss, good return loss and it is useful in Ku-band satellite communication applications.
Disclosure statement
No potential conflict of interest was reported by the author(s).
ACKNOWLEDGMENTS
We express our gratitude to the Council of Scientific and Industrial Research (CSIR), India for funding this work under SRF-Direct Category (File No:09/0895(11398)/2021-EMR-I).
Additional information
Funding
Notes on contributors
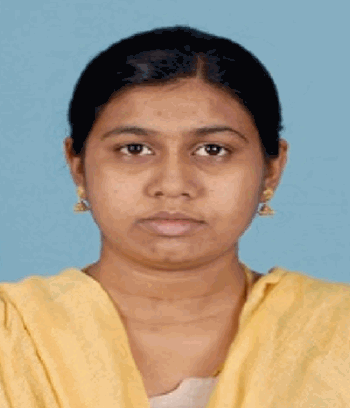
Gopalakrishnan Soundarya
Gopalakrishnan Soundarya received the BE degree in electronics and communication engineering from SNS College of Technology, Tamilnadu, India in 2009 and ME degree in communication systems from Sri Shakthi Institute of Engineering and Technology, Tamil Nadu, India in 2012. She is currently pursuing PhD at the National Institute of Technology, Tiruchirappalli, Tamilnadu, India. Her research interests include design and development of microstrip and substrate-integrated waveguide technology-based filters and antennas.
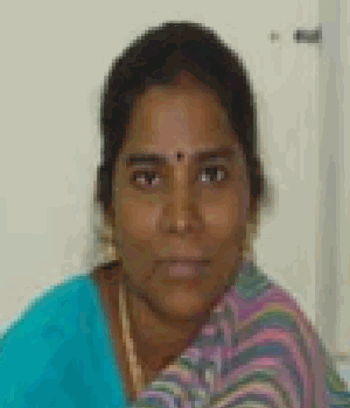
Nagarajan Gunavathi
Nagarajan Gunavathi studied at RVS College of Engineering and Technology, Dindigul, Tamilnadu, and received the BE degree in electronics and communication engineering from Madurai Kamaraj University, Tamilnadu, India. She completed ME degree in microwave and optical engineering from Alagappa Chettiar Government College of Engineering and Technology, Karaikudi, Tamilnadu, India. She received PhD degree in microwave engineering from the National Institute of Technology, Tiruchirappalli, India in 2016, where, she is an assistant professor in the Department of Electronics and Communication Engineering. She has 19 years of teaching experience. Published more than 17 papers in international journals and presented more than 23 papers at international and national conferences. Her research area of interest includes SAR-reduced mobile handset antennas, Metamaterial inspired planar antennas, SIW BPF filter for satellite communication, dual band/multiband antenna for 5G applications, and reconfigurable antennas. She is a Lifetime Member of IETE. Email: [email protected]