Figures & data
Figure 1. We illustrate the periodization process by displaying both a noisy data vector (left graph) representing a non-periodic function
defined on
. The cubic spline, defined on
and that matches the slopes of
at t = 0 and t = 1 is also illustrated (left graph). The combined vector represents a periodic function defined on
. We also show the exact derivative
(right graph) and the approximate derivative
for
(right graph). The approximation is reasonably good both for t = 0 and t = 1.
![Figure 1. We illustrate the periodization process by displaying both a noisy data vector fΔ (left graph) representing a non-periodic function f(t) defined on [0,1]. The cubic spline, defined on [1,2] and that matches the slopes of f(t) at t = 0 and t = 1 is also illustrated (left graph). The combined vector represents a periodic function defined on [0,2]. We also show the exact derivative f′(t) (right graph) and the approximate derivative DξcfΔ for ξc=35 (right graph). The approximation is reasonably good both for t = 0 and t = 1.](/cms/asset/a79b477f-b2b1-47d4-94a1-873b7d4a31a8/gapa_a_1876224_f0001_oc.jpg)
Figure 2. We display the functions and
in the top-left graph. In the top-right graph we show the error
, as a function of
, for the noise level
. Note that there is an optimal value for
. In addition we display the solution
, for
(middle left), which is close to the optimum, and for
(middle right). Also the exact solution
is displayed. In the bottom-left graph we show the optimal
as a function of the noise level ϵ and in the bottom-right graph we show the corresponding error, for the optimal
, as a function of ϵ. Note that for a larger noise level ϵ, we need a smaller value of
, and obtain a larger error in the computed surface temperature
.
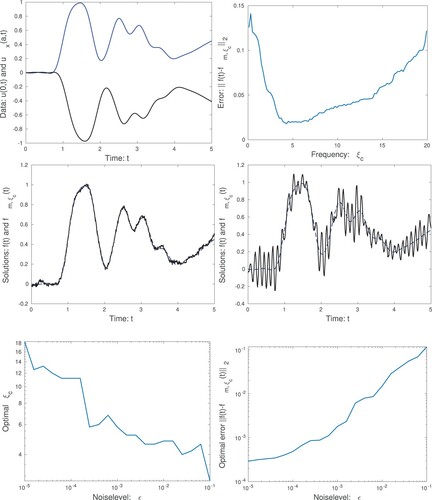
Figure 3. In the top-left graph we show the error , as a function of λ, for the noise level
. Note that there is an optimal value for λ. In the top-right graph we display the surface temperature for
which is close to the optimal value. In the bottom-left graph we show the optimal λ as a function of the noise level ϵ and in the bottom-right graph we show the corresponding error, for the optimal λ, as a function of ϵ. Note that for a larger noise level ϵ, we need a larger value of λ, and obtain a larger error in the computed surface temperature
.
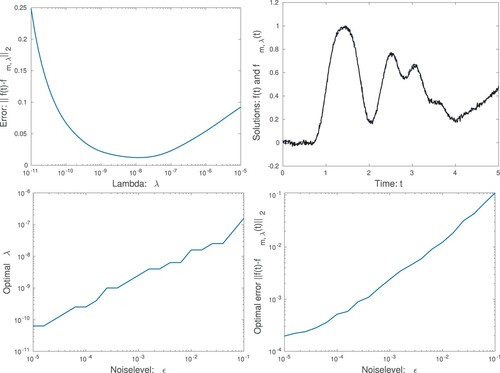
Figure 4. We present tests where the exact solution is a smoothed step function. The top graphs show the error
(left) for the spline method and the error
(right) for the Fourier method. The middle graphs display the numerical solutions
(left) obtained using the spline method and
and the solution
computed using the Fourier method and
. The lower graphs show the errors
x markers) and
o markers) for different random noise sequences
. In the left graph the variance of the noise is
,
and
. In the right graph instead
,
and
. In both cases 100 different sets of random noise were generated.
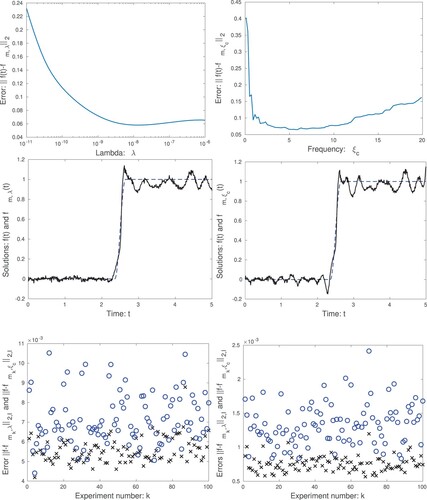