Figures & data
Table 1. FCM model factors for prediction of ischemic stroke.
Figure 3. Sum of the three linguistic variables suggested by neurologists for the concept of blood cholesterol (C2) and obtaining numerical weights by defuzzification using the centre of area method.
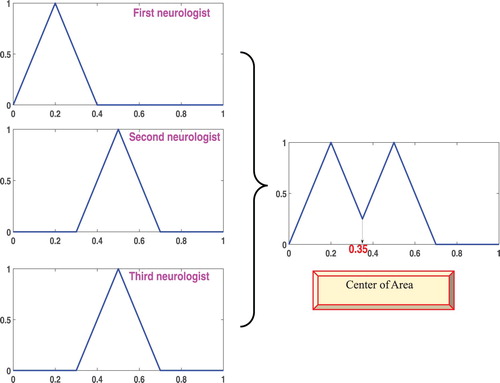
Figure 4. FCM model for predicting the risk of ischemic stroke with numerical values of the initial weights.
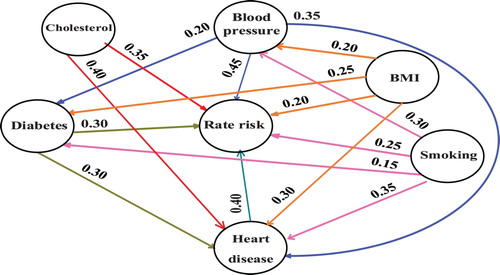