Abstract
Nonconformities are the major sources of waste in manufacturing process. Nonconformities cannot be fully eliminated but their occurrence rate can be predicted. This paper proposes a hybrid approach based on ontological modelling and machine learning for predicting the non-conformance rates of a manufacturing process and minimising its associated costs. Based on the proposed approach, the work orders, that are represented semantically using a formal ontology, are first clustered according to their semantic similarities and then, for each cluster, the appropriate models that predict the probability distribution of non-conformance rates are developed. When a new work order is created, the most similar work order is retrieved from historical records, and the probability distribution of its non-conformance rate is estimated by applying the predictive model of the cluster to which the work order belongs. The probability distribution is used to calculate the expected underproduction and overproduction cost and to determine the amount of production that minimises the expected costs. The proposed method was validated using a dataset obtained from a manufacturer of packaging for cosmetics. Compared to the expert’s opinions and other machine learning algorithms, the proposed method demonstrated better performance with respect to cost reduction.
Acknowledgements
An earlier version of this paper was published in the proceedings of IFIP International Conference on Advances in Production Management Systems, 2019, pp. 291–298.
Disclosure statement
No potential conflict of interest was reported by the author(s).
Additional information
Notes on contributors
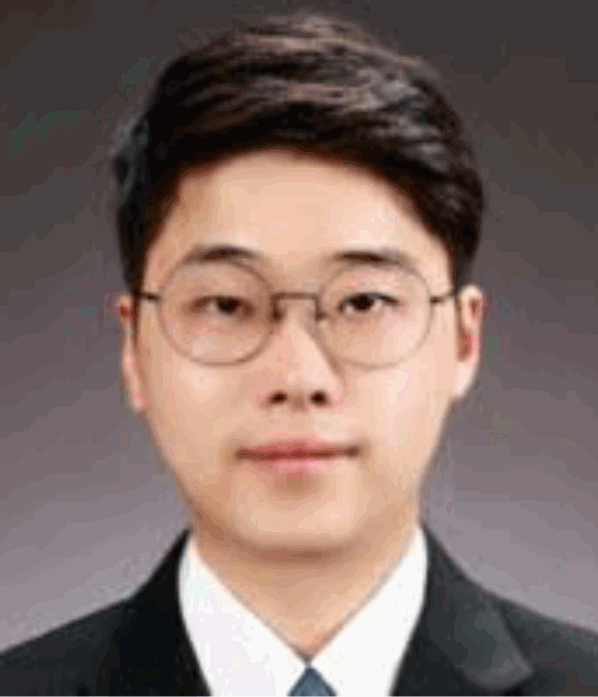
Bongjun Ji
Bongjun Ji is a PhD candidate in the Department of Industrial Engineering at the Pohang University of Science and Technology, visiting scholar at the Department of Engineering and Technology at Texas State University. His research interests include ontology engineering and machine learning application in the context of Smart Manufacturing System
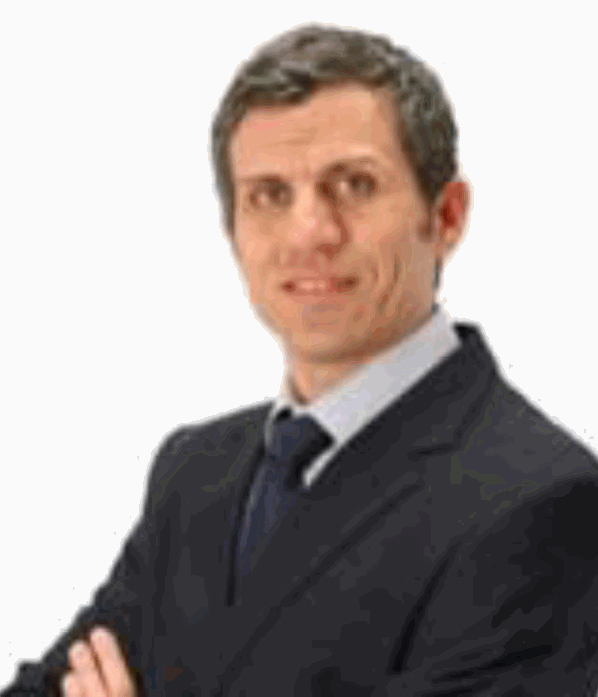
Farhad Ameri
Farhad Ameri is a professor in the Department of Engineering and Technology at Texas State University, San Marcos. He received his Doctoral in Manufacturing Engineering from the University of Michigan, Ann Arbor, in 2006. His research interests include engineering knowledge representation and artificial intelligence in design and manufacturing
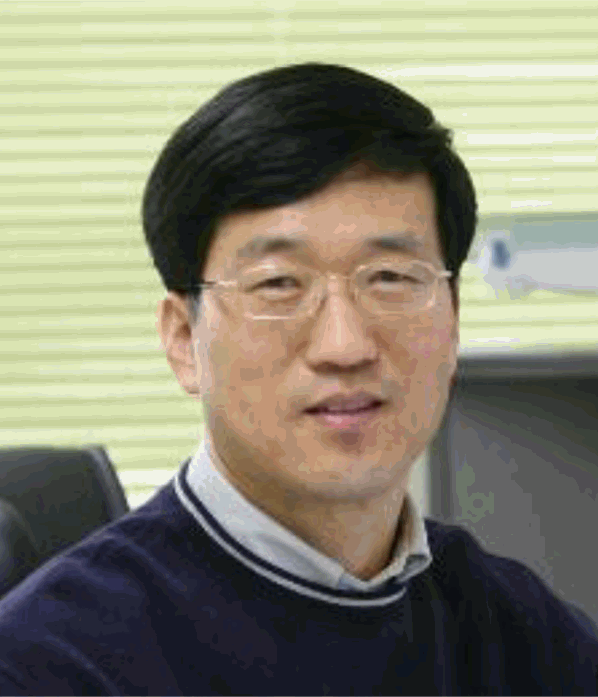
Hyunbo Cho
Hyunbo Cho is a professor of Department of Industrial and Management Engineering at POSTECH. He received his BS and MS degrees in Industrial Engineering from Seoul National University in 1986 and 1988, respectively, and his PhD in Industrial Engineering with a specialisation in Manufacturing Systems Engineering from Texas A&M University in 1993. His areas of expertise include Supply Chain Management, Manufacturing Management and Strategy and Open Business Model