Abstract
With the advent of mass customisation, solving the assembly sequence planning (ASP) problem not only involves a non-convex optimisation problem that is hard to solve but also requires a high-speed response to the changes of assembly resources. This paper proposes a deep reinforcement learning (DRL) approach for the ASP problem, aiming at promoting the response speed by exploiting the reusability and expandability of past decision-making experiences. First, the connector-based ASP problem is described in a matrix manner, and its objective function is set to minimise assembly cost under the precedence constraints. Secondly, an instance generation algorithm is developed for policy training, and a mask algorithm is adopted to screen out impracticable assembly operations in each decision-making step. Then, the Monte Carlo sampling method is used to evaluate the ASP policy. The policy is learned from an actor–criticbased DRL algorithm, which contains two networks, policy network and evaluation network. Next, the network structures are introduced and they are trained by a mini-batch algorithm. Finally, four cases are studied to validate this method, and the results are discussed. It is demonstrated that the proposed method can solve the ASP problem accurately and efficiently in the environment with dynamic resource changes.
Disclosure statement
No potential conflict of interest was reported by the author(s).
Additional information
Funding
Notes on contributors
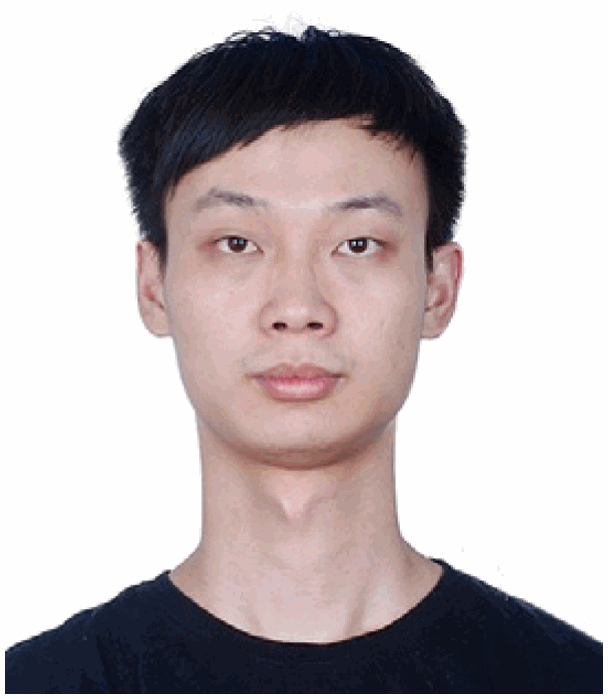
Wenbo Wu
Wenbo Wu is a PhD student of the School of Mechanical Science and Engineering from the Huazhong University of Science and Technology (HUST), People’s Republic of China. In 2014, he received his B.S. degree in Mechanical manufacturing and automation from the Wuhan University of Science and Technology. His research interest focusses on solving the computer-aided process planning problem by using AI/ML methods. He is the author and co-author of about 8 publications in relevant international journals, regarding machining feature recognition, setup planning and resources allocation.
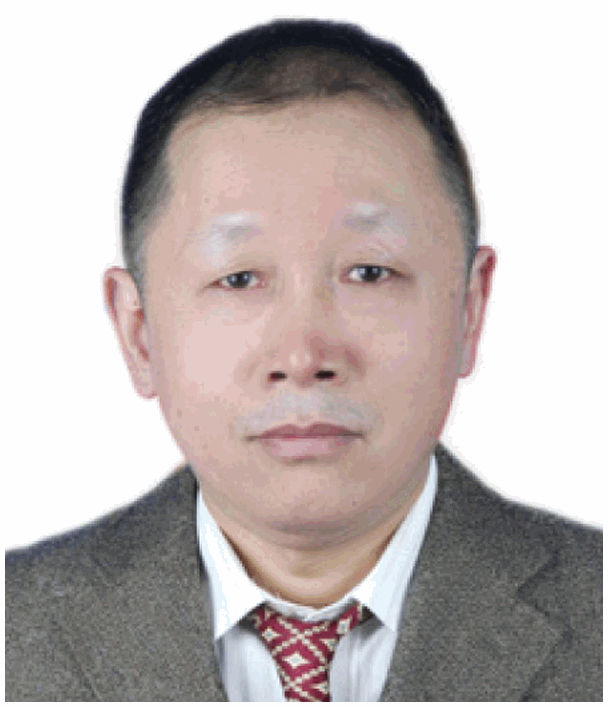
Zhengdong Huang
Zhengdong Huang is a professor in the CAD centre at the Huazhong University of Science and Technology (HUST), People’s Republic of China. He worked as a post-doctor in the ERC for Reconfigurable Machining Systems at the University of Michigan, Ann Arbor, from 1998 to 2002. He received his BS degree in Computational Mathematics from Wuhan University, his MS degree in Applied Mathematics from Zhejiang University and his PhD degree in Mechanical Engineering from HUST. His research interests include computer graphics, geometrical modelling, design optimisation and computer-aided process planning.
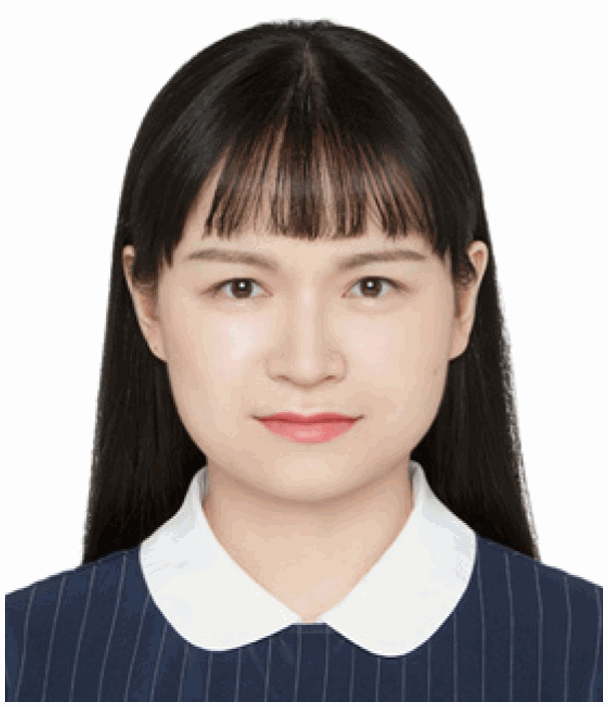
Jiani Zeng
Jiani Zeng is a PhD student at the Huazhong University of Science and Technology (HUST), People’s Republic of China. She achieved the Bachelor Degree in engineering from HUST. Her research topics include mechanical analysis and structural optimisation of composite structures.
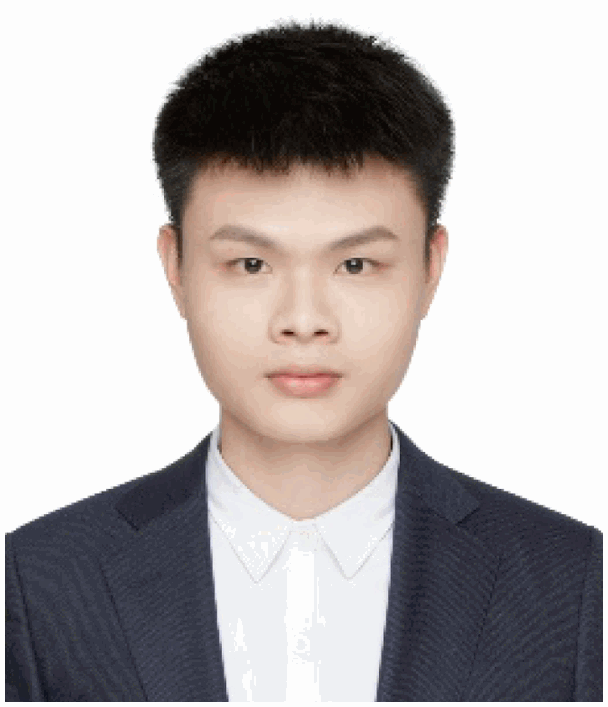
Kuan Fan
Kuan Fan is a PhD student of the School of Mechanical Science and Engineering from the Huazhong University of Science and Technology. He received his bachelor’s degree from Jilin University in 2017. His research interests include engineering optimisation, isogeometric analysis and parallel computing.