ABSTRACT
As the core link of intelligent manufacturing, the process planning of aviation parts still faces the challenges such as relying on manual experiences for process decision-making and lack of linkage between process design and manufacturing for process optimisation. Process knowledge could support scientific decision-making on process issues, while twin data, namely high-fidelity simulation data and feedback information of manufacturing site, could further verify the process plans and optimise process parameters, so as to continuously improve the quality of process plans. Consequently, this paper proposes a general framework for twin data and knowledge-driven intelligent process planning (TDKIPP) of aviation parts, and analyses four standard procedures that support the above-mentioned reference framework, namely mechanism-data fusion process digital twin model, dynamic process knowledge base, process decision-making and evaluation, machining quality prediction and process feedback optimisation. A thus constructed test bed of TDKIPP and its four application examples about the process planning of a micro turbojet engine integral impeller demonstrate the feasibility and effectiveness of the proposed approach.
Acknowledgements
This work was supported by the [National Key Research and Development Program of China] under Grant [2018YFB1702400], and [National Natural Science Foundation of China] under Grant [number 51975463], and [China National Postdoctoral Program for Innovative Talents] under Grant [number BX2021244].
Disclosure statement
No potential conflict of interest was reported by the author(s).
Additional information
Funding
Notes on contributors
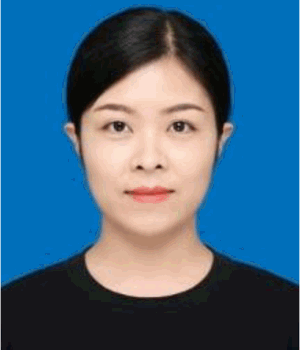
Jingjing Li
Jingjing Li is currently pursuing the Ph.D. degree in mechanical engineering with the School of mechanical engineering, Xi’an Jiaotong University, Xi’an, China. Her main research interests are related to intelligent process planning, knowledge-driven decision-making, and intelligent manufacturing systems.
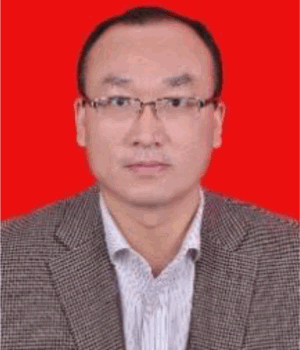
Guanghui Zhou
Guanghui Zhou received the B.E., M.E. and Ph.D. degree in mechanical engineering from Xi’an Jiaotong University, Xi’an, Shaanxi, in 1996, 1999 and 2003. From 2010 to 2011, he was a visiting scholar with the Department of Industrial and Manufacturing Systems Engineering, Florida State University. He is currently the Professor with the School of Mechanical Engineering, Xi’an Jiaotong University. His research interests include service-oriented networked digital manufacturing, low-carbon manufacturing, intelligent manufacturing and knowledge-based manufacturing systems. He is the author of two monographs, more than 100 journal and conference papers, and 20 inventions. His research results won the first prize of the Natural Science of the Ministry of Education of China.
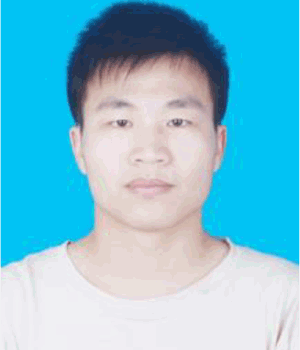
Chao Zhang
Chao Zhang received the B.E. degree in mechanical engineering and automation from Sichuan University, Chengdu, China, in 2015, and Ph.D degree in mechanical engineering from Xi’an Jiaotong University, Xi’an, Shaanxi, in 2020. He is currently an Assistant Professor with the School of Mechanical Engineering, Xi’an Jiaotong University. He has authored a monograph, 20 journal and conference papers, and 10 inventions. His research interests include intelligent manufacturing systems, knowledge-driven decision-making, and deep learning.