Abstract
This paper studies a common planning problem encountered in steelmaking production. The problem is to group different customer orders into a set of batches to accommodate the mass production mode of steelmaking furnaces. We formulate the problem as a novel mixed-integer programming model by considering the practical technological requirements. To solve the problem, we propose a cooperative coevolution framework in which an effective decomposition scheme based on graph partitioning is developed. The decomposition scheme first explores the problem structure by considering the production process rules and then exploits the batch information of the best-so-far solution to identify the potentially better decompositions. To solve each decomposed subcomponent, we propose a new differential evolution algorithm which incorporates a subpopulation-based classification mechanism and local search with an external archive strategy to balance the abilities of exploration and exploitation. Computational tests on a set of real production data as well as on a more diverse set of randomly generated problem instances show that our method is effective and efficient in practical application and outperforms other benchmark algorithms.
Disclosure statement
No potential conflict of interest was reported by the author(s).
Additional information
Funding
Notes on contributors
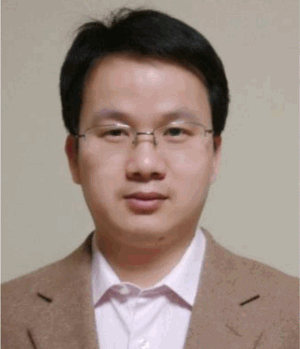
Gongshu Wang
Gongshu Wang received the B.Eng. degree in automation, the M.Eng. degree in systems engineering and the Ph.D. degree in logistics optimisation & control from Northeastern University, Shenyang, China, in 2002, 2005, 2008, respectively. He is an associate professor at the Key Laboratory of Data Analytics and Optimisation for Smart Industry at Northeastern University, China. His major research interests are in the areas of production planning and scheduling in the steel industry and integer and combinatorial optimisation methods. He has published several articles in academic journals such as Operations Research, Naval Research Logistics, Omega, Journal of the Operational Research Society, Computers & Operations Research and Interfaces.
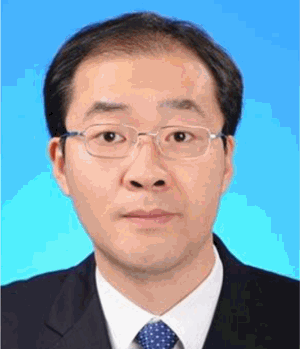
Qingxin Guo
Qingxin Guo received the B.Eng. degree in automation, the M.Eng. degree and the Ph.D. degree in systems engineering from Northeastern University, Shenyang, China, in 2003, 2006, 2016, respectively. He is an associate professor at the Key Laboratory of Data Analytics and Optimisation for Smart Industry at Northeastern University, China. His major research interests are in the areas of production planning and scheduling in the steel industry and computational intelligence optimisation algorithms. He has published several articles in academic journals including International Journal of Production Research, Computers & Industrial Engineering, Applied Soft Computing and ISIJ international.
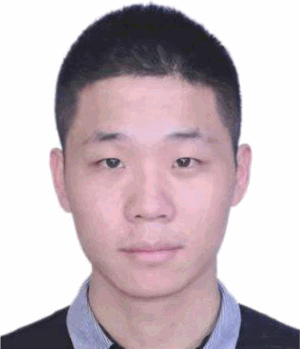
Wenjie Xu
Wenjie Xu received the B.Eng. degree in automation and the Ph.D. degree in systems engineering from Northeastern University, Shenyang, China, in 2012, 2018, respectively. He visited Texas A&M University in 2015–2017 sponsored by China Scholarship Council. He currently is an algorithm engineer at JD Intelligent Supply Chain Y. His major interests are retail product categories, product innovation, inventory optimisation, and data mining and optimisation in e-commerce.
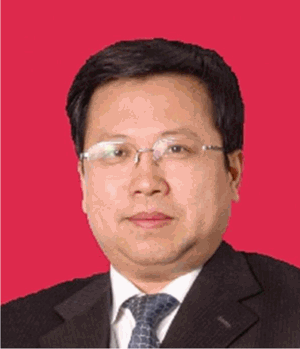
Lixin Tang
Lixin Tang received the B.Eng. degree in industrial automation, the M.Eng. degree in systems engineering and the Ph.D. degree in control theory and application from Northeastern University, Shenyang, China, in 1988, 1991, 1996, respectively. He is a member of the Chinese Academy of Engineering, the Director of Key Laboratory of Data Analytics and Optimisation for Smart Industry, Ministry of Education, China, and the Chair Professor of Centre for Industrial Intelligence and Systems Optimisation, Northeastern University. His research interests cover industrial intelligence and systems optimisation theories and methods, including data analytics and machine learning, deep learning and evolutionary learning, reinforcement learning and dynamic optimisation, convex and sparse optimisation, integer and combinatorial optimisation, computational intelligence-based optimisation. Meanwhile, he applies the above theories to engineering applications in manufacturing, logistics and energy systems. He has published more than 127 papers in international journals such as Operations Research, Manufacturing & Service Operations Management, INFORMS Journal on Computing, IISE Transactions, and Naval Research Logistics, et al. His paper published on IISE Transactions received the Best Applications Paper Award of 2017. He currently serves as an Associate Editor of IISE Transactions, IEEE Transactions on Evolutionary Computation, IEEE Transactions on Cybernetics, Journal of Scheduling, International Journal of Production Research, and Journal of the Operational Research Society. Meanwhile, he is on the Editorial Board of Annals of Operations Research and serves as an Area Editor of the Asia-Pacific Journal of Operational Research.