Figures & data
Figure 1. Agent–environment interaction; Sutton and Barto (Citation2017).
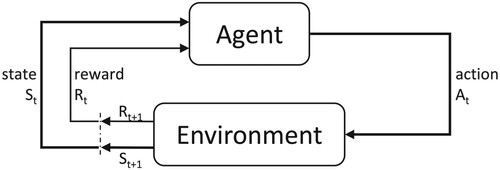
Table 1. Taxonomy framework of the SLR.
Table 2. Defined keywords for the SLR.
Figure 4. Analysis of yearly deep RL publications, 2021 includes Jan./Feb. (a) Yearly and accumulated deep RL publications (b) Yearly deep and non-deep RL publications.
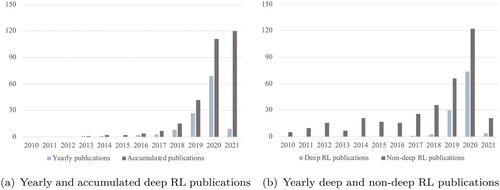
Table 3. Summary of deep RL applications in process control.
Table 4. Summary of deep RL applications in production scheduling, dispatching, and (intra-) logistics.
Table 5. Summary of deep RL applications in assembly and robotics.
Table 6. Summary of deep RL applications in maintenance, energy management, and (process) design.
Table 7. Summary of deep RL applications in quality control and further applications.
Table 8. Summary of the key findings from the review analysis.