Figures & data
Figure 1. Overview of the framework of the proposed SSDL.
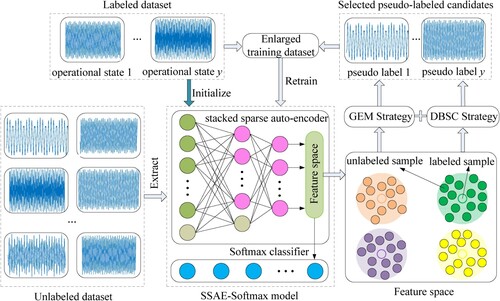
Figure 2. Structure of SAE and SSAE-Softmax neural network: (a) symmetrical structure neural network of SAE, where is the dimension of each sample,
is the number of neurons in the hidden layer 1; and (b) structure of SSAE-Softmax classifier, where
and
are the number of neurons in the hidden layer 2 and hidden layer 3, respectively,
denotes the probability that
is classified as operational state
(
).
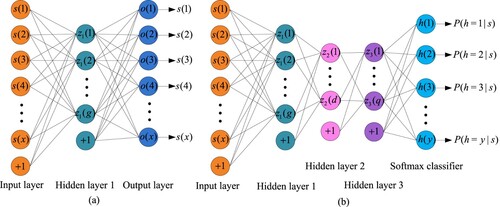
Figure 3. Flowchart of constructing SSDL model for machinery fault diagnosis.
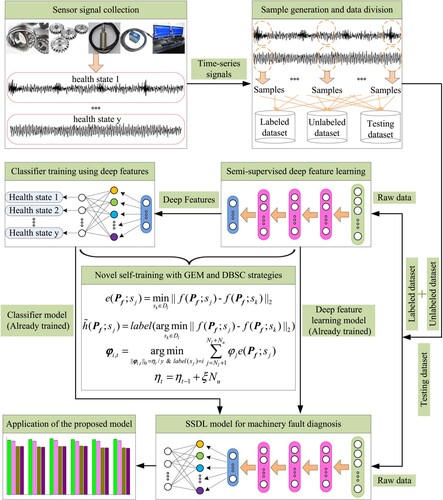
Table 1. Description of the four datasets for bearing fault diagnosis.
Figure 4. Experimental data preparation for delta 3-D printer: (a) test-rig; (b) installed attitude sensor; (c) normal and fault state of a synchronous belt; and (d) normal and fault state of a joint bearing screw.
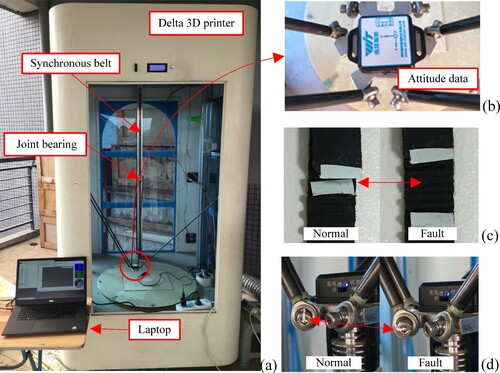
Table 2. Description of the four datasets for 3-D printer fault diagnosis.
Table 3. Diagnosis accuracies of SSDL under different combinations of network architecture and enlarging factor.
Table 4. Hyper-parameter settings for all the approaches on bearing datasets.
Table 5. Hyper-parameter settings for all the approaches on 3-D printer datasets.
Figure 5. The prediction accuracies obtained by all the algorithms on each testing dataset.
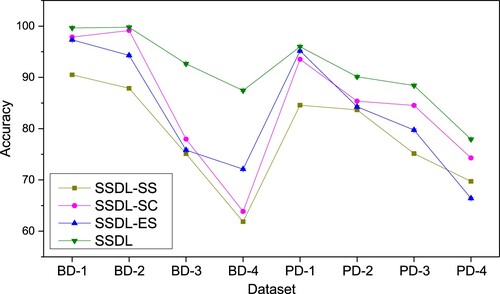
Figure 6. The iterative Accu and Accs values of the contrastive approaches on the BD-1: (a) SSDL-SS; (b) SSDL-SC; (c) SSDL-ES; and (d) SSDL.
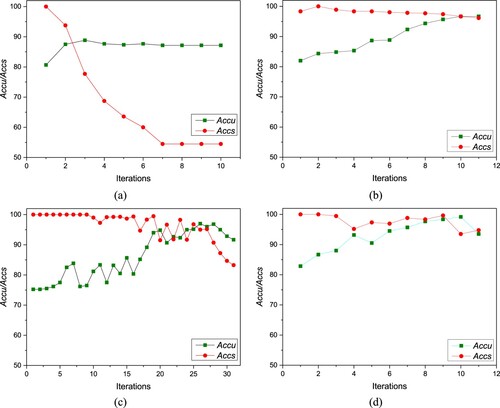
Figure 7. The iterative Accu and Accs values of the contrastive approaches on the BD-4: (a) SSDL-SS; (b) SSDL-SC; (c) SSDL-ES; and (d) SSDL.
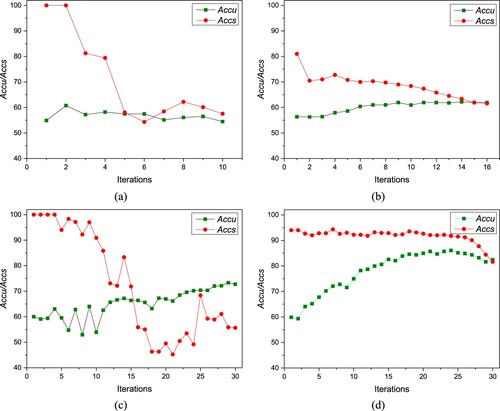
Figure 8. The prediction accuracies obtained by SSDL under different number of labelled samples on datasets PD-3 and PD-4.
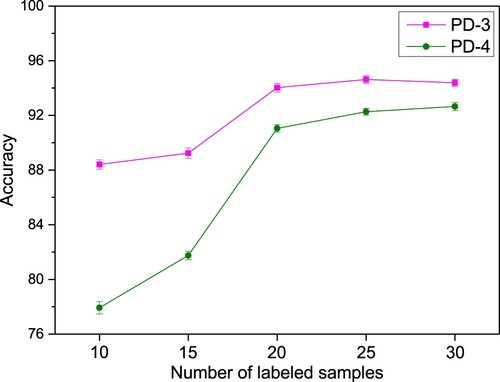
Table 6. Comprehensive comparison of diagnosis accuracy.