Abstract
Two of the major problems Traditional Manufacturing (TM) supply chains face are setting requisite reactive strategies to address the uncertainties in demand and the optimal placement of these buffering capacities in order to be both responsive and cost-effective. With Additive Manufacturing (AM) stepping into large-scale production at different firms, we address the aforementioned supply chain dilemmas by considering the potential role of AM in a TM supply chain network where AM facilities can act as a recourse to the TMs and, also, as a dedicated source providing responsive and cost-effective sourcing alternatives. We develop an analytical allocation rule based on cost differentials, which provides optimal sourcing decisions through sequential demand replenishment and facilitates an efficient performance evaluation of possible network configurations. We first model the scenario as a three-stage stochastic optimisation problem. We then solve it using the allocation rule and present an illustration of our analysis and the optimal supply chain network configuration. Furthermore, we derive some insights as to how different problem characteristics affect the value and usage of AM.
Data availability statement
The data that support the findings of this study are available from the corresponding author, HSH, upon reasonable request.
Disclosure statement
No potential conflict of interest was reported by the author(s).
Additional information
Notes on contributors
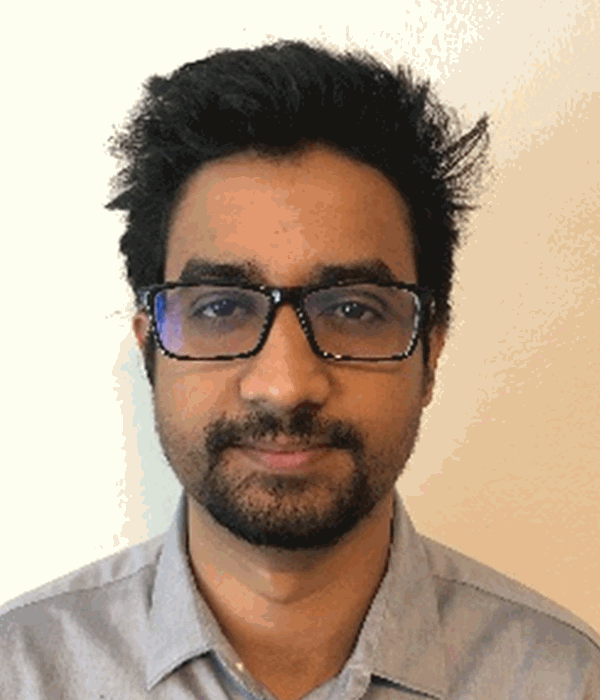
Ramin Ahmed
Ramin Ahmed received his Master's and Ph.D. degrees in Industrial Engineering from North Carolina State University in 2018 and 2021, respectively. He has a B.Sc. degree in Industrial and Production Engineering from Khulna University of Engineering and Technology, Bangladesh (2015). His research interests include Supply Chain, Production, and Logistics Systems analysis, modelling, and optimisation.
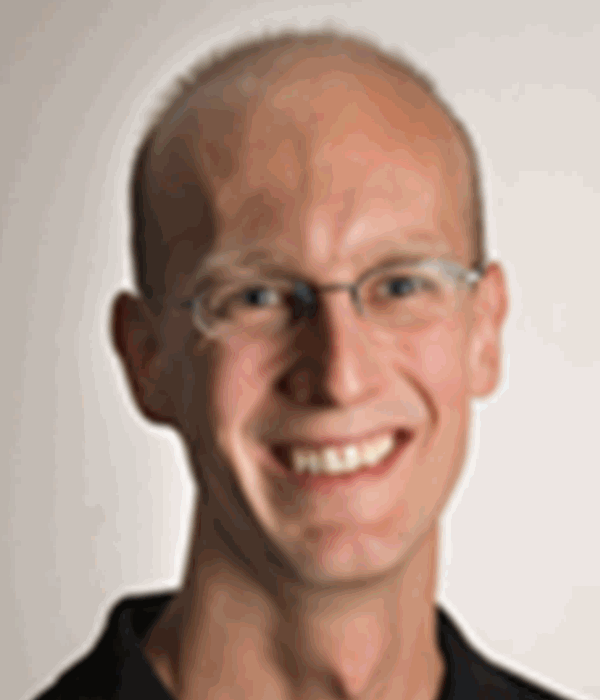
H. Sebastian Heese
Hans Sebastian (Seb) Heese holds the Owens Distinguished Professorship of Supply Chain Management at NC State University. His research aims at increasing the efficiency of production and service operations systems, and it often addresses the consequences of interest conflict in decentralised decision-making. His work has been widely published in the leading international academic journals. Before joining NC State University, Seb Heese was Dean of EBS Business School in Wiesbaden, Germany, where he also served as Executive Director of the Institute for Supply Chain Management and held the SGL Carbon Endowed Chair of Supply Chain Management. Prior to EBS, he served on the faculty at the Kelley School of Business at Indiana University, Bloomington, USA. Sebastian Heese holds a Ph.D. from the University of North Carolina at Chapel Hill, USA.
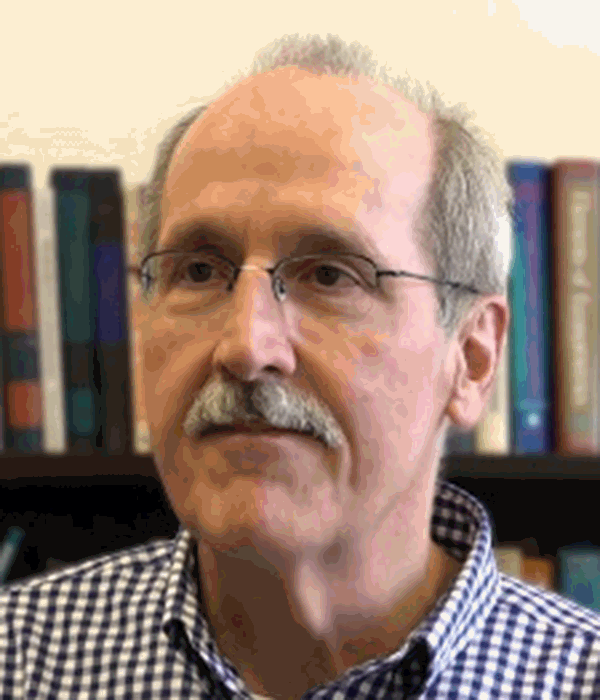
Michael Kay
Michael G. Kay is director of Integrated Manufacturing Systems Engineering and interim director of the Graduate Program in Operations Research at North Carolina State University. He did his doctoral studies at NC State's Center for Robotics and Intelligent Machines and teaches courses in production system design, logistics engineering, and operations research. His research is currently focused on the development of public logistics networks.