Abstract
The semiconductor industry is a resource-intensive sector that heavily relies on energy, water, chemicals, and raw materials. Within the semiconductor manufacturing process, the diffusion furnace, ion implantation machine, and plasma etching machine exhibit high energy demands or operate at extremely high temperatures, resulting in significant electricity consumption, which is usually carbon-intensive. To address energy conservation concerns, the industry adopts batch production technology, which allows for the simultaneous processing of multiple products. The energy-efficient parallel batch scheduling problem arises from the need to optimise product grouping and sequencing. In contrast to existing heuristics, meta-heuristics, and exact algorithms, this paper introduces the Deep Q-Network (DQN) algorithm as a novel approach to address the proposed problem. The DQN algorithm is built upon the agent’s systematic learning of scheduling rules, thereby enabling it to offer guidance for online decision-making regarding the grouping and sequencing of products. The efficacy of the algorithm is substantiated through extensive computational experiments.
Acknowledgments
This research has received financial support from various sources, including the Ministry of Education of Humanities and Social Science Project [grant number 22YJC630050], the China Postdoctoral Science Foundation [grant number 2022M710996], the Educational Commission of Anhui Province [grant number KJ2020A0069], the Natural Science Foundation of Anhui Province [grant numbers 2108085QG291 and 2108085QG287], Anhui Province University Collaborative Innovation Project [grant number GXXT-2021-021], Science and Technology Plan Project of Wuhu [grant number 2021yf49, 2022rkx07], National Natural Science Foundation of China [grant numbers 72101071 and 72071056], the Key Research and Development Project of Anhui Province [grant number 2022a05020023].
Data availability statement
The data that support the findings of this study are available from the authors upon reasonable request.
Disclosure statement
No potential conflict of interest was reported by the author(s).
Additional information
Funding
Notes on contributors
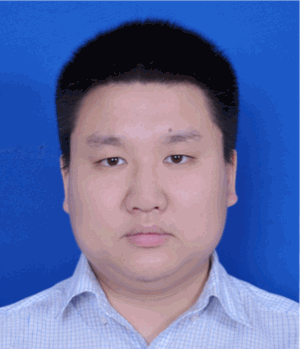
Min Kong
Min Kong received his Bachelor’s degree in Management from Hefei University of Technology, Hefei, China, in 2015. He received his Ph.D. degree in Management from the Hefei University of Technology in 2020. He serves as an associate professor in the School of Management, Anhui Normal University, China. His research interests include supply chain scheduling and the application of the Internet of Things. He has published papers in the Journal of Global Optimisation, the International Journal of Production Research, and Annals of Operations Research, etc.
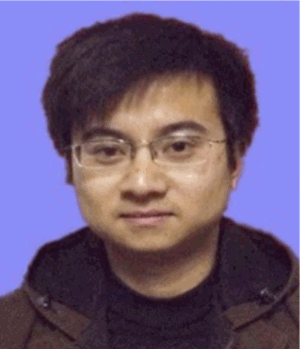
Weizhong Wang
Weizhong Wang received an M.Sc. degree in management science and engineering from the China University of Mining and Technology, Xuzhou, in 2014. He received a Ph.D. in Management Science and Engineering from the School of Economics & Management, Southeast University, in 2021. He serves as an associate professor at the School of Economics and Management, Anhui Normal University. His research results have been published in IISE Transactions, IEEE Transactions on Reliability, Safety Science, Computers & Industrial Engineering, International Journal of Production Research, and Information Fusion, among others. His current research interests include decision analysis, risk analysis, and energy transition.
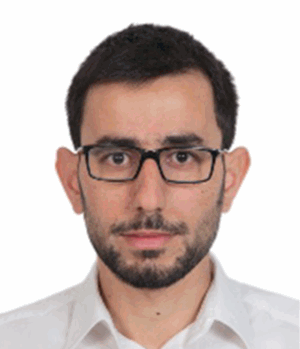
Muhammet Deveci
Dr. Muhammet Deveci is an Honorary Senior Research Fellow with the Bartlett School of Sustainable Construction, University College London, UK, and he is a Visiting Professor at Royal School of Mines in the Imperial College London, London, UK. Dr. Deveci is also an Associate Professor at the Department of Industrial Engineering in the Turkish Naval Academy, National Defence University, Istanbul, Turkey. Dr. Deveci has published over 170 papers in journals indexed by SCI/SCI-E papers at reputable venues. Dr Deveci has also been engaged with the wider community providing academic service through chairing/organising conferences, streams, tutorials, reviewing papers, and acting as Editorial Board Member of well-known journals including IEEE T-IV, INS, ASOC, EAAI, ESWA, AIR, and more. Dr Deveci is an internationally recognised outstanding scientist in intelligent decision support systems underpinned by computational intelligence, particularly uncertainty handling, fuzzy systems, combinatorial optimisation, and multicriteria decision making. His research and development activities are multidisciplinary and lie at the interface of Operational Research, Computer Science and Artificial Intelligence Science. Based on the 2020 and 2021 publications from Scopus and Stanford University, he is within the world's top 2% scientists in the field of Artificial Intelligence.
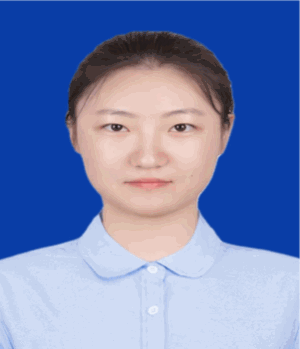
Yajing Zhang
Yajing Zhang received her Bachelor's degree in Management from Anhui Normal University, China, in 2017. She is currently pursuing an academic Master's degree in Business Administration at Anhui Normal University. Her research interests involve job scheduling, deep reinforcement learning, and human resource management.
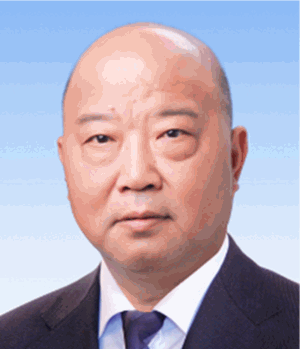
Xuzhong Wu
Xuzhong Wu received his Bachelor’s degree in Law from Anhui Normal University, Wuhu, China, in 1994. He received his Master’s degree in Law from Anhui Normal University in 2003. He received his Ph.D. in Economics from Xiamen University, Xiamen, China, in 2009. He serves as a professor at the School of Economics and Management, Anhui Normal University. His main research interests include Marxist political economy and property economics. He has published papers in journals such as the Chinese Journal of Political Economics and Information Technology Industrial Engineering, etc.
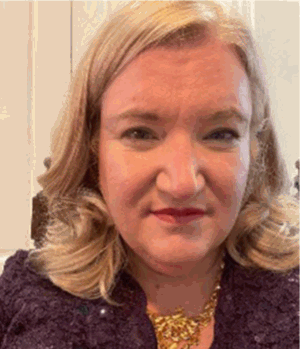
D'Maris Coffman
D'Maris Coffman is Professor of Economics and Finance of the Built Environment at University College London. From 2017 to 2023, she was Director and Head of Department of the School of Sustainable Construction. She has recently been promoted to Vice Dean, Innovation & Enterprise, of The Bartlett Faculty of the Built Environment. Her research interests are at the interstices of economic geography, economic history and infrastructure economics, and she has recently revived a longstanding interest in operations research.