Abstract
Managing blood donations is a challenging problem due to the perishability of blood, limited donor pool, deferral time restrictions, and demand uncertainty. The problem addressed here combines two important aspects of blood supply chain management: the inventory control of blood products and the donation schedule. We propose a stochastic scenario-based reformulation of the blood donation management problem that adopts multicomponent apheresis and utilises donor pool segmentation into here-and-now and wait-and-see donors. We propose a flexible donation scheme that is resilient against demand uncertainty. This scheme enables more flexible donation schedules because wait-and-see donors may adjust their donation schedules according to the realised values of demand over time. We propose a column generation-based approach to solve the associated multi-stage stochastic donation tailoring problem. The numerical results show the effectiveness of the proposed optimisation model, which provides solutions with less than a 7% optimality gap on average with respect to a lower bound. It also improves the operational cost of the standard donation scheme that does not use wait-and-see donors by more than 18% on average. Utilising multicomponent apheresis and flexible wait-and-see donations are suggested for donation organisations because they yield significant cost reductions and resilient donation schedules.
Acknowledgments
The writers express their gratitude to two anonymous reviewers whose feedback was instrumental in enhancing the quality of this paper.
Data Availability Statement
The data that support the findings of this study are available on request from the corresponding author.
Disclosure statement
No potential conflict of interest was reported by the author(s).
Additional information
Notes on contributors
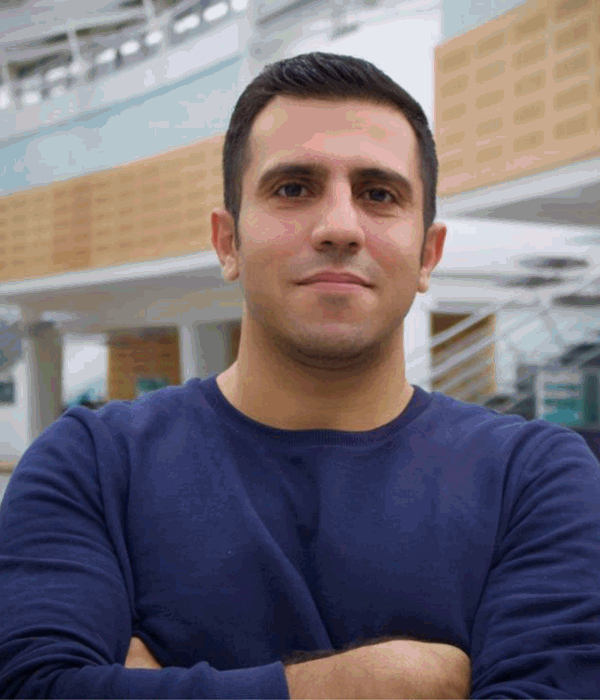
Milad Elyasi
Dr. Milad Elyasi is a Postdoctoral Fellow at HEC Montreal.Prior to HEC Montreal, he was a Postdoctoral Fellow at IMT Atlantique. He earned his Ph.D. in Industrial Engineering from Ozyegin University, Istanbul, Turkey, where he focussed on the application of large-scale optimization methods in scheduling and routing problems. Prior to this, he completed his M.Sc. in Industrial Engineering from K.N. Toosi University of Technology, Tehran, Iran, and his B.Sc. from the University of Tabriz, Tabriz, Iran. His research interests encompass transportation and logistics system planning, optimization under uncertainty, manufacturing systems, decomposition algorithms for large-scale optimization problems, combinatorial optimization techniques, and the application of machine learning methodologies.
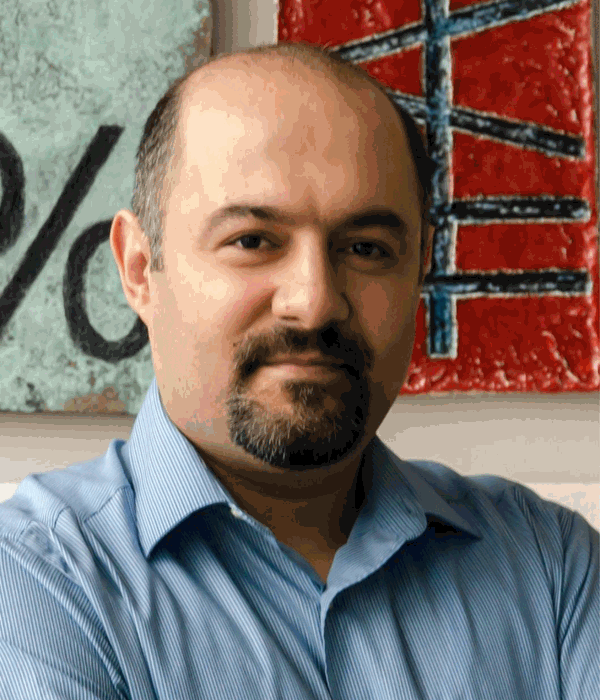
Okan Örsan Özener
Dr. Okan Örsan Özener is a Professor of Industrial Engineering at Ozyegin University in Turkey. His research interests are in integer/combinatorial optimization, data-driven optimization, machine learning, non-cooperative/cooperative game theory and their applications in supply chain management primarily on transportation, distribution, and logistics systems planning and control, production and scheduling.
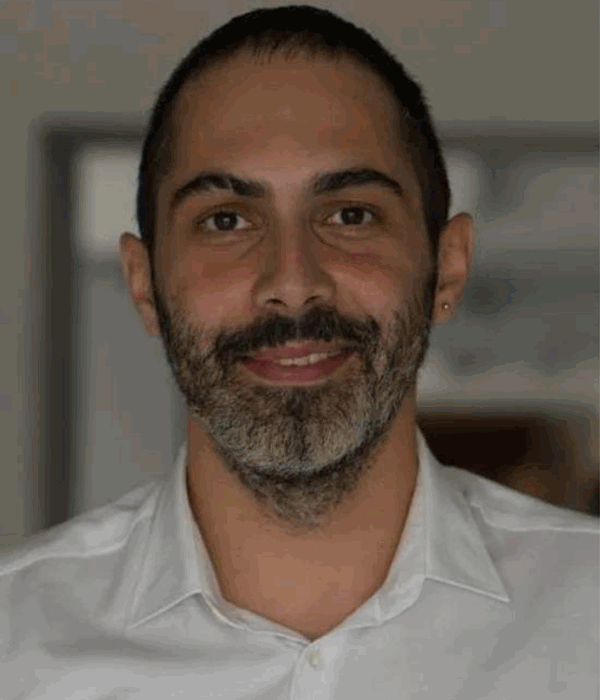
İhsan Yanıkoğlu
Dr. İhsan Yanıkoğlu earned his Bachelor of Science (B.Sc.) degree in Industrial Engineering from Bilkent University in 2007, followed by a Master of Science (M.Sc.) degree in the same field in 2009. He then went on to complete a Master of Philosophy (M.Phil.) degree in Operations Research and Econometrics at Tilburg University in 2010, and he obtained his Doctor of Philosophy (Ph.D.) degree from the same institution in 2014. His primary research interests are centred around optimization under uncertainty, focussing on robust and stochastic optimization. In recent years, he has dedicated his research efforts to developing practical optimization methodologies and their real-world applications.
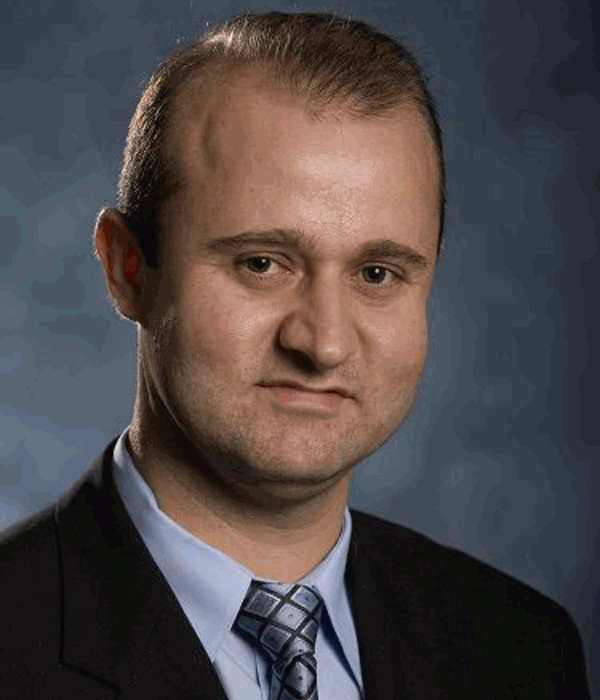
Ali Ekici
Dr. Ali Ekici is a Professor of Industrial Engineering at Ozyegin University in Turkey. His research interests include routing problems in both commercial and humanitarian settings, packing and scheduling. He specifically develops algorithms for practical variants of inventory routing and bin packing problems.
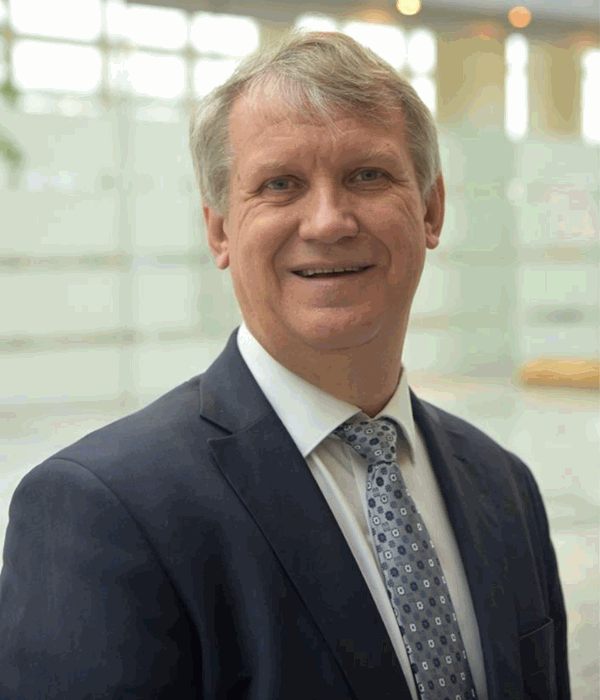
Alexandre Dolgui
Dr. Alexandre Dolgui is an IISE Fellow, Distinguished Professor and the Head of Automation, Production and Computer Sciences Department at the IMT Atlantique. His research and teaching activities focuse on manufacturing line design, production planning and supply chain optimization. He is the co-author of 5 books, the co-editor of 25 books or conference proceedings, the author of over 300 refereed papers in international journals. He is the Editor-in-Chief of the International Journal of Production Research, an Area Editor of Computers & Industrial Engineering. He is an Active Fellow of the European Academy for Industrial Management, Member of the Board of the International Foundation for Production Research, Chair of IFAC TC 5.2 Manufacturing Modelling for Management and Control, Member of IFIP WG 5.7 Advances in Production Management Systems, IEEE System Council Analytics and Risk Technical Committee, he has been Scientific Chair of many leading scientific conferences.