Figures & data
Figure 1. (a) Conventional approaches and (b) the proposed approach to multi-domain tool wear prediction.
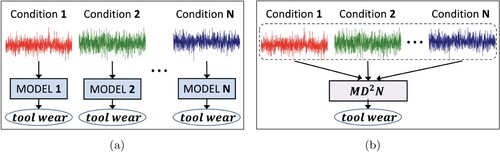
Figure 2. MDN: the proposed model architecture.
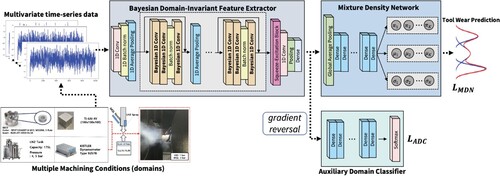
Figure 3. The detailed architectural structure of BDIFE.
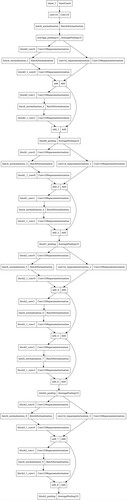
Figure 4. The experimental setup for the milling process.
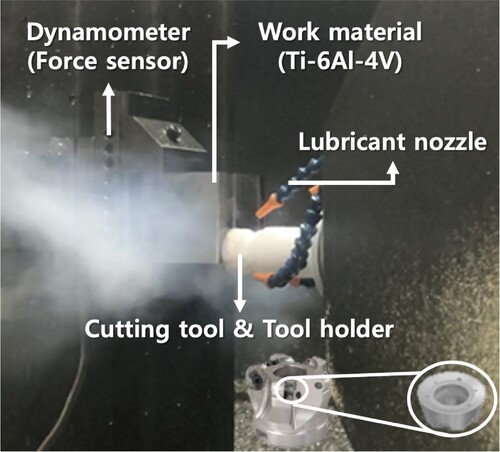
Figure 5. The schematic diagram of the experimental setup.
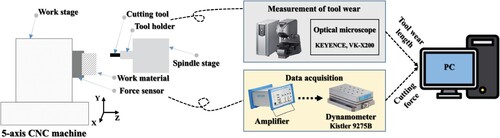
Table 1. Milling experiment conditions.
Table 2. Descriptive statistics of datasets.
Figure 6. Visualisation of sensor measurements.
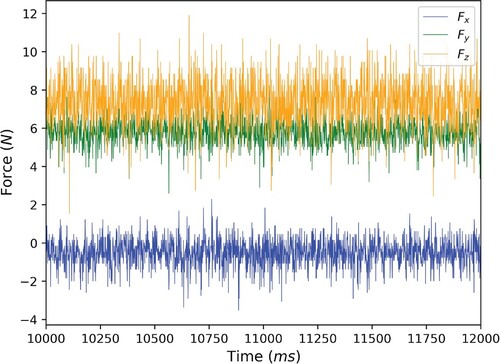
Figure 7. Measured tool wear of (a) Experiment 2 and (b) Experiment 6.
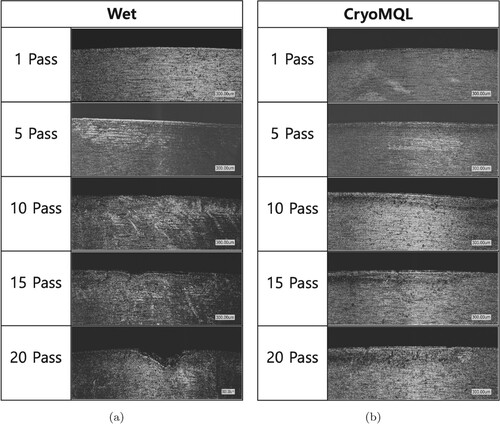
Table 3. Prediction performance for wet and CryoMQL setting data.
Table 4. Prediction performance for both settings data.
Figure 8. Tool wear prediction performance of MDN on all datasets under wet and CryoMQL settings. (a) Dataset 1. (b) Dataset 2. (c) Dataset 3. (d) Dataset 4. (e) Dataset 5. (f) Dataset 6. (g) Dataset 7 and (h) Dataset 8.
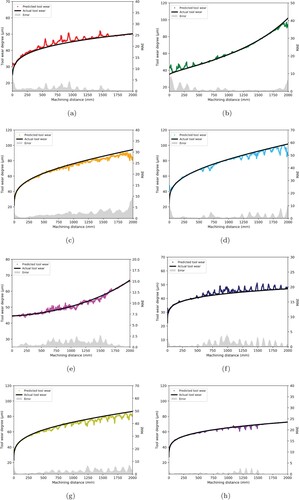
Figure 9. t-SNE visualisation of the latent space of MDN: (a) before training, (b) after 100 epochs, (c) after 200 epochs, (d) after 300 epochs, and (e) after training.
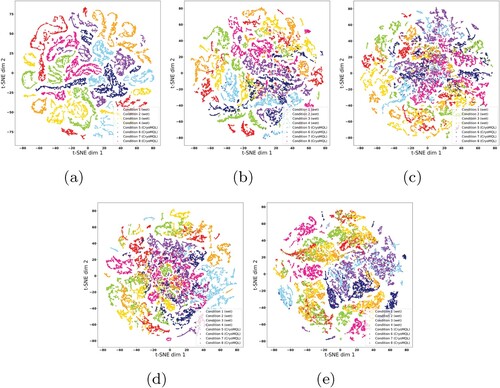
Table 5. Performance comparison with existing models.
Table 6. Performance comparison with state-of-the-art methods.
Data availability statement
The data that support the findings of this work are available from the corresponding author, [SL], upon reasonable request.