Abstract
Rescheduling in a hybrid flowshop holds significant importance in modern industries that face uncertain events. Moreover, real-world manufacturing scenarios often utilise lot streaming to enhance market competitiveness. In light of escalating energy demands and their consequential environmental impacts, contemporary manufacturing companies are placing a heightened emphasis on energy efficiency. This study addressed a green hybrid flowshop rescheduling problem with consistent sublots (GHFRP_CS) in the context of urgent lot insertion. Initially, we establish an optimisation model aimed at minimising the makespan, total energy consumption, and system stability. To tackle this NP-hard multi-objective optimization problem, we develop a constructive heuristic generating promising solutions based on lot split, sequence, and local search rules. Further improvement is achieved through a multi-objective discrete artificial bee colony algorithm (MDABC). MDABC decomposes the problem into sub-problems, initiating solutions with the constructive heuristic and refining them through employed bee, onlooker bee, and scout bee phases. Computational experiments compare MDABC with other multi-objective evolutionary algorithms (MOEAs) on small- and large-scale problems. Results demonstrate MDABC's superiority, achieving fourfold accuracy and efficiency enhancement for small-scale instances and sixfold improvement for large-scale problems at low cost compared to other MOEAs.
Disclosure statement
No potential conflict of interest was reported by the author(s).
Date availability
The data that support the findings of this study are available from the corresponding author, upon reasonable request.
Additional information
Funding
Notes on contributors
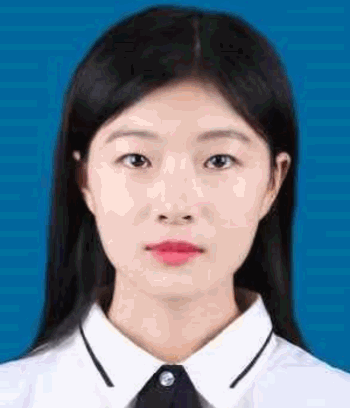
Weiwei Wang
Weiwei Wang received the B.Eng. degree from the School of Computer Science, Shandong Institute of Petroleum and Chemical Technology, Dongying, China. She is currently pursuing the M.Eng. degree with Liaocheng University, Liaocheng, China. Her current research interests include intelligent optimisation and scheduling.
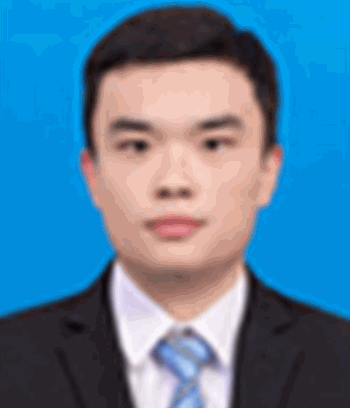
Biao Zhang
Biao Zhang is currently a lecturer in the School of Computer Science in at Liaocheng University, Liaocheng, China. He received the B.S. and Ph.D. degrees from Shandong University of Technology, Zibo, China and Huazhong University of Science and Technology, Wuhan, China, in 2012 and 2019, respectively. His current research interests include machine learning and intelligent optimisaiton. He has authored more than 40 refereed papers. He is a guest editor at Symmetry.
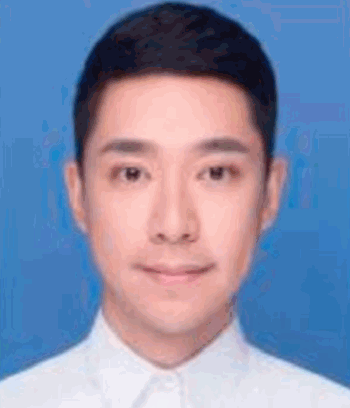
Xuchu Jiang
Xuchu Jiang is currently a lecturer in the School of statistics and mathematics at Zhongnan University of Economics and Law, China. He received his Ph.D. degree in mechanical engineering from Huazhong University of Science and Technology in 2019. Now, his research field is data mining and analysis in industry.
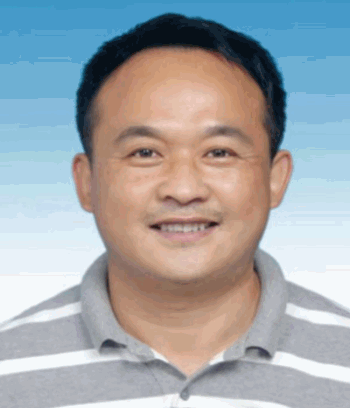
Baoxian Jia
Baoxian Jia is currently an associate professor in the the School of Computer Science, Liaocheng University. He received his Ph.D. degree in educational big data from Tsinghua University in 2019. Now, his research areas are education big data and e-commerce.
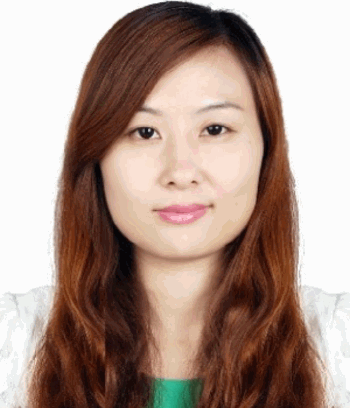
Hongyan Sang
Hongyan Sang received the M.S. degree from the School of Computer Science, Liaocheng University, Liaocheng, China, in 2010, and the Ph. D. degree in industrial engineering from Huazhong University of Science Technology, Wuhan, China, in 2013. Since 2003, she has been with the School of Computer Science, Liaocheng University, where she became a Professor in 2021.
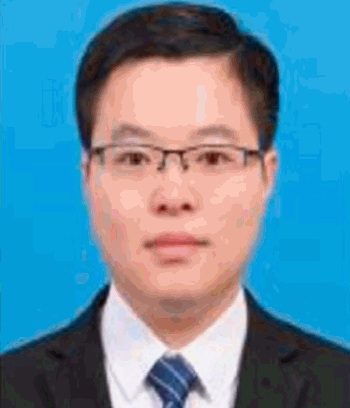
Leilei Meng
Leilei Meng is currently a lecturer in the School of Computer Science in at Liaocheng University, Liaocheng, China. He received the B.S. degree in mechanical engineering from Chang’an University, Xian, China, in 2014, and the Ph.D. degree in the School of Mechanical Science and Engineering at Huazhong University of Science and Technology, Wuhan, China, in 2020. His research mainly focuses on modelling, optimisation of scheduling problems, tool wear prediction and sustainable manufacturing.