Abstract
In this paper, the problem of distributed consensus estimation with randomly missing measurements is investigated for a diffusion system over the sensor network. A random variable, the probability of which is known a priori, is used to model the randomly missing phenomena for each sensor. The aim of the addressed estimation problem is to design distributed consensus estimators depending on the neighbouring information such that, for all random measurement missing, the estimation error systems are guaranteed to be globally asymptotically stable in the mean square. By using Lyapunov functional method and the stochastic analysis approach, the sufficient conditions are derived for the convergence of the estimation error systems. Finally, a numerical example is given to demonstrate the effectiveness of the proposed distributed consensus estimator design scheme.
Acknowledgements
We thank the editor and four anonymous reviewers for their constructive comments, which helped us to improve the manuscript.
Disclosure statement
No potential conflict of interest was reported by the authors.
Additional information
Funding
Notes on contributors
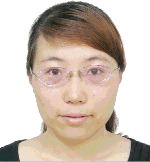
Zhengxian Jiang
Zhengxian Jiang received her BS degree in mathematics in 2000 and MS degree in applied mathematics in 2006, both from Soochow University, Suzhou, China. She is currently working toward the PhD degree in control theory and control engineering from Jiangnan University, Wuxi, China. She is currently a lecturer at Jiangnan University. Her current research interests include distributed parameter systems, wireless sensor and actuator networks.
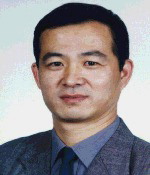
Baotong Cui
Baotong Cui received his PhD degree in control theory and control engineering from the College of Automation Science and Engineering, South China University of Technology, China, in July 2003. He was a postdoctoral fellow at Shanghai JiaoTong University, China, from July 2003 to September 2005, and a visiting scholar at Department of Electrical and Computer Engineering, National University of Singapore, Singapore, from August 2007 to February 2008. He joined Jiangnan University, China, in June 2003, where he is a full professor for the School of IoT Engineering. His current research interests include systems analysis, artificial neural networks and chaos synchronisation.
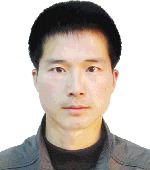
Xuyang Lou
Xuyang Lou received his PhD degree in control theory and control engineering from Jiangnan University, Wuxi, China, in 2009. In 2010, he joined the School of Communication and Control Engineering, Jiangnan University. From October 2007 to October 2008, he was a visiting scholar in the CSIRO Division of Mathematical and Information Sciences, Adelaide, Australia. From July to November 2010, he was a postdoctoral fellow in the Department of Electrical Engineering (ESAT-SCD/SISTA), Katholieke Universiteit Leuven. From July 2014 to February 2015, he visited the Computer Engineering Department, University of California, Santa Cruz, CA, USA. He is currently an associate professor at Jiangnan University. His current research interests include computational intelligence, hybrid systems and biological neural networks.