Figures & data
Table 1. Demographics of sample
Table 2. Model fit across latent profile analyses specifying from 1 to 5 latent classes
Table 3. Characterizing the latent commitment classes
Table 4. Differences across latent commitment classes on background, demographic and outcome factors
Figure 1. Latent relationship structures extracted by LPA. S = subjects referring to themselves; P = subjects’ perceptions of their romantic partners; Monog = Monogamy; EDSA = extra dyadic sexual activity; MONOG – min EDSA = Monogamous relationships with minimal amounts of recent extra-dyadic sexual activity; MONOG – low EDSA = Monogamous relationships with low amounts of recent EDSA; OPEN – CNM = Open relationships that could be classified as consensual nonmonogamy.
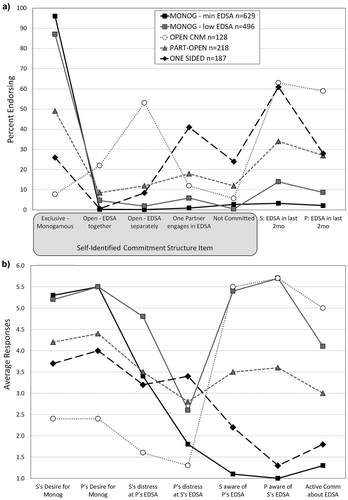
Figure 2. The COMMIT4: a four-item reconstruction of latent classes derived from decision tree analyses. Decision tree analyses (a form of machine learning) were conducted using the DecisionTreeClassifier from the scikit-learn package (Pedregosa et al., Citation2012). The tree was constrained to five leaf nodes corresponding to the five desired latent classes (See the methods section for the full range of responses offered for question 2). The COMMIT4 classification system was derived via a machine learning decision tree algorithm to represent the most parsimonious method of recreating the 5 latent classes with the fewest items. It was therefore not intended to represent substantive findings (nor should it be substantively interpreted), but instead represents a practical application for future research.
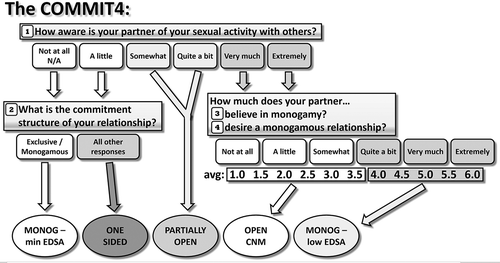