Figures & data
Figure 2. Summary of the hPF phospholipid model. Left: CG representation of the DPPC phospholipid and solvent. Right: Outline of the two terms in the hPF Hamiltonian.
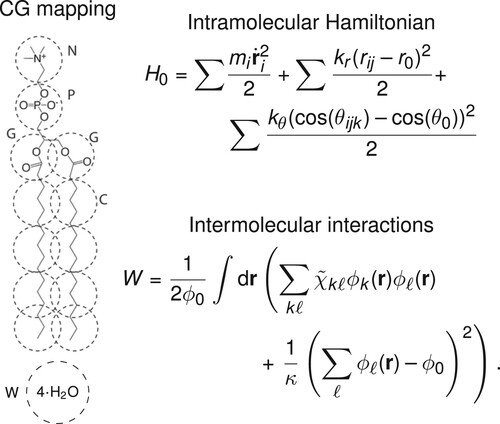
Figure 3. Density profiles and representative membrane snapshots from hPF-MD simulations of a DPPC bilayer using parameters [Citation21] (left), particle-based simulations using the MARTINI CG force field (centre), and hPF-MD
parameters (right). The table presents absolute deviations
in the density profiles between the F-H and BO parameter simulations, and the reference MARTINI profile. Percentage deviations
are given in parenthesis.
values are given in el./nm
.
![Figure 3. Density profiles and representative membrane snapshots from hPF-MD simulations of a DPPC bilayer using χ~F−H parameters [Citation21] (left), particle-based simulations using the MARTINI CG force field (centre), and hPF-MD χ~BO parameters (right). The table presents absolute deviations Sk in the density profiles between the F-H and BO parameter simulations, and the reference MARTINI profile. Percentage deviations Sp are given in parenthesis. Sk values are given in el./nm3.](/cms/asset/be4d674e-a047-44dc-80fc-6734b5a2fca5/tmph_a_1785571_f0003_c.jpg)
Figure 4. Left: Feature importance as ranked by the mutual information measure between the fitness and the individual parameters, for hPF-MD simulations of a DPPC bilayer with randomly sampled
matrices. Presented values are normalised relative to the most important parameter (
) (arbitrary units). Inset details the low relative MI values found for the last six matrix elements (error bars omitted).
parameters are included in order of decreasing feature importance (left). Right: Best fitness achieved (here using the average coefficient of determination,
, across all bead species) for each dimension of the parameter space subspace used in hPF-MD BO protocol runs on the DPPC bilayer system.
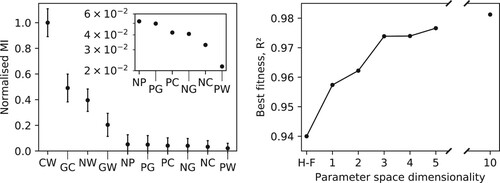
Figure 5. Top: Density profiles for hPF-MD DPPC bilayer simulations ran with Bayesian optimised parameter sets with four (left) and ten (right) included parameters. The four-parameter simulation uses
values for all but the
matrix elements with the highest feature importance, namely
,
,
, and
, c.f. column three of the table (bottom). Bottom: Resulting
matrices from the BO protocol applied to hPF-MD simulations of a DPPC bilayer. Results reported for selected subspaces of the full 10-dimensional parameter space, with the
s shown in red being fixed and not part of the optimisation run. All
values given in
. Mean percentage errors,
, associated with each set of optimised parameters is given in the last row.
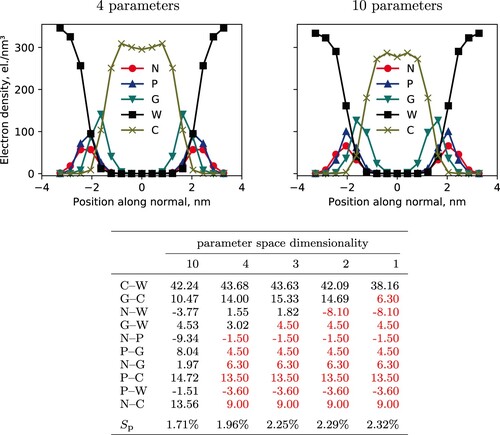
Table 1. Optimised 
-matrix parameters found by the BO scheme for hPF-MD simulations of DPPC, DMPC, DSPC, and DOPC bilayer systems. The 
matrix elements are given in order of decreasing feature importance. Reference parameters are the Flory-Huggins (
) parameters used in [Citation21]. All values given in units of 
.
Table 2. Mean absolute deviations in electron density, 
, with respect to the MARTINI reference density for the different lipids simulated with Bayesian optimised parameter sets on the different phospholipids (relative percentage deviations 
in parenthesis). Comparison with data from De Nicola, using the baseline 
parameter set [Citation21]. All 
values given 
.
Figure 6. Top left: The surrogate objective fitness surface (here using the average coefficient of determination, , across all bead species) in an example DPPC BO run with only the four parameters exhibiting the highest feature importance scores included (
,
,
, and
). Individual samplings with their associated fitnesses are represented as blue dots. A projection onto the subspace spanned by
and
shown. All
matrix elements are given in
. Top right: Best DPPC simulation membrane fitness (average
) for BO and random sampling with only the four parameters exhibiting the highest feature importance scores included. Comparison with the fitness achieved by the reference
parameter set. Inset details when BO and random sampling surpass the
parameter set in terms of
fitness. Bottom: Scatter matrices showing correlations between all pairs of
parameters in a BO run on a DPPC bilayer (left) compared with random sampling (right). Only the four parameters exhibiting the highest feature importance scores are included in the sampling. The matrix diagonal shows the density of sampled points for each individual
parameter. All
matrix elements are given in
.
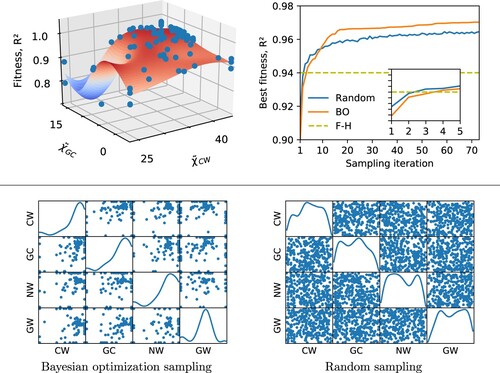
Data availability statement
The data that support the findings of this study are available from the corresponding author upon reasonable request.