Figures & data
Table 1. Descriptive statistics of grazing heifers (n = 5,004) from 54 dairy herds that had puberty traits collected on three occasions at approximately 10 (visit 1; V1), 11 (visit 2; V2) and 12 months of age (visit 3; V3).
Figure 1. Proportion of Holstein (black), Friesian (grey) and Jersey (white) breeds represented in dairy heifers (n = 5,004) from herds (n = 54) in North Waikato, South Waikato, Taranaki, and North Otago regions included in a study of risk factors associated with the onset of puberty.
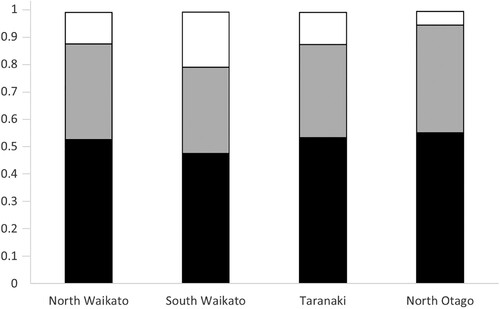
Table 2. Individual risk factors associated with the likelihood of an animal reaching puberty in a population of 5,004 grazing heifers across 54 herds. Fifteen separate multivariable logistic regression model outputs for the effects of liveweight, height, length, anogenital distance (AGD), or proportion of mature liveweight (PMLWT) measured at visit 2 (V2) are presented for the chance of an animal being pubertal or not by visit 1 (V1), visit 2 (V2) or visit 3 (V3), after adjusting for age at V2, and Holstein and Jersey breed proportions, with herd included as a random effect (for each model).
Table 3. Combined risk factors associated with the likelihood of an animal reaching puberty in a population of 5,004 grazing heifers across 54 herds. Multivariable logistic regression model outputs for the effects of age at visit 2 (V2), Holstein and Jersey breed proportions, liveweight, height, length, anogenital distance (AGD), and proportion of mature liveweight (PMLWT) on the chances of an animal being pubertal at visit 1 (V1), V2 or visit 3 (V3) with herd included as a random effect.
Table 4. Results of Cox proportional hazards model and HR (95% CI) for the effect of liveweight, proportion of mature liveweight (PMLWT), height, length and anogenital distance (AGD) on the hazard of puberty for dairy heifers (n = 5,004, from 54 herds). Age at visit 2 (V2; approximately 11 months of age) and proportion Holstein and Jersey were included in the model as potential confounders and herd was included as the random effect.
Figure 2. Cumulative percentage of heifers (n = 5,004) within each of the 58 heifer groups that had elevated blood progesterone (>1 ng/mL) indicating puberty attained by visit 1 (black; heifers approximately 10 months old), visit 2 (grey; heifers approximately 11 months old) or visit 3 (white; heifers approximately 12 months old).
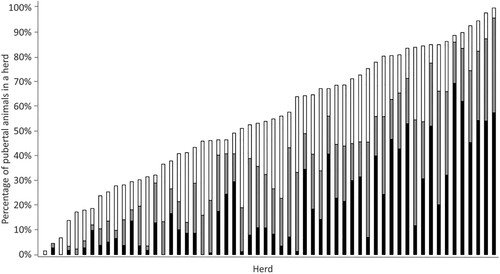
Table 5. Herd-level risk factors influencing the proportion of heifers in a dairy herd that had reached puberty when the mean age of heifers (n = 5,004, from 54 herds) was 299 days (at visit 1Table Footnotea). Partial least squares model outputs are presented including raw and standardised regression coefficients, and variable importance in projection (VIP) values for the first component.
Table 6. Herd-level risk factors influencing the proportion of heifers in a dairy herd that had reached puberty when the mean age of heifers (n = 5,004, from 54 herds) was 327 days (at visit 2Table Footnotea). Partial least squares model outputs are presented including raw and standardised regression coefficients, and variable importance in projection (VIP) values for the first component.
Table 7. Herd-level risk factors influencing the proportion of heifers in a herd that had reached puberty when the mean age of heifers (n = 5,004, from 54 herds) was 355 days (at visit 3Table Footnotea). Partial least squares model outputs are presented including raw and standardised regression coefficients, and variable importance in projection (VIP) values for the first component.
Figure 3. Correlation loading plots for the partial least squares (PLS) model of herd-level risk factors influencing the proportion of heifers in a herd that had reached puberty by (a) visit 1 (heifers approximately 10 months old), (b) visit 2 (heifers approximately 11 months old), and (c) visit 3 (heifers approximately 12 months old). Component 1 explains a proportion of the variation in the response, and the variables furthest from 0 along the x-axis (a greater X-loading value) have the greatest influence (represented by the vector arrows). Component 2 explains an additional proportion of the variation in the response and the variables furthest from 0 along the y-axis have the greatest influence (represented by the vector arrows). The vector arrows represent the X-loadings (at 4× scale for ease of interpretation) for each predictor that comprises each PLS component. The black dots represent the X-scores for each herd.
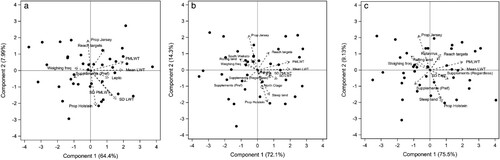