Figures & data
Table 1. Differences in related work.
Figure 2. Quality score distribution of the selected studies. (a): Box plot with quantiles, median, and mean values. (b): Histogram with density, median, and mean values.
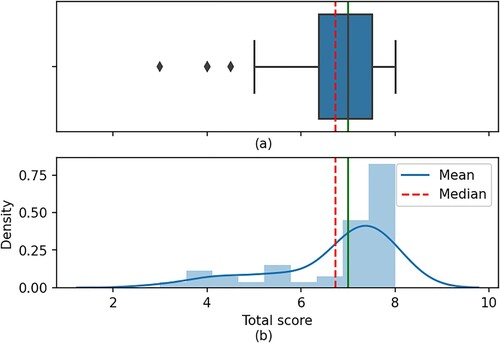
Table 2. Top-5 values of keywords occurrence.
Table 3. Top-5 values of occurrence for key motivation.
Table 4. Top-5 values of occurrence for crops.
Table 5. Top-5 values of occurrence for data features.
Table 6. Values of occurrence for data features groups.
Table 7. Values of occurrence for datasets.
Table 8. Values of occurrence for all implementation frameworks.
Table 9. Values of occurrence for all categories.
Table 10. Top-5 values of occurrence for algorithms.
Table 11. Values of occurrence for algorithms’ performance.
Table 12. Values of occurrence for evaluation approaches.
Table 13. Values of occurrence for challenges and proposed solutions reported in primary studies.