Figures & data
Table 1. R packages used by caret.
Table 2. Number of samples for Indian pines data.
Figure 1. Indian Pines AVIRIS hyperspectral data and classification results. The image was acquired 12 June 1992. The centre of the image is at approximately 40.46111° N, 87.01111° W, and the image is oriented with North up. (a) False colour composite of blue = band 28, green = band 65, and red = band 128. (b) The reference class map. (c) The SVM classification using a balanced training data set and feature selection. (d) The RF classification using a balanced training data set and feature selection.
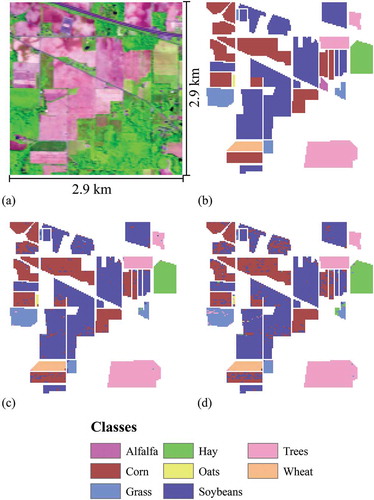
Table 3. Number of samples for urban GEOBIA data.
Table 4. Classification results for Indian pines data.
Table 5. Classification results for urban GEOBIA data.
Table 6. Classification of Indian pines data using RF and original training data.
Table 7. Classification of Indian pines data using RF and balanced training data.
Table 8. Classification of Indian pines data using SVM and original training data.
Table 9. Classification of Indian pines data using SVM and balanced training data.
Table 10. User-defined parameter examples.
Figure 2. Impact of parameters on classification performance evaluated by comparison against validation data. Note that the y-axis scaling for (c) and (d) differ from that of the other graphs.
(a) GEOBIA k–NN, (b) Indian pines k–NN, (c) GEOBIA DT, (d) Indian pines DT, (e) GEOBIA RF, (f) Indian pines RF, (g) GEPBIA SVM, (h) Indian pines SVM.
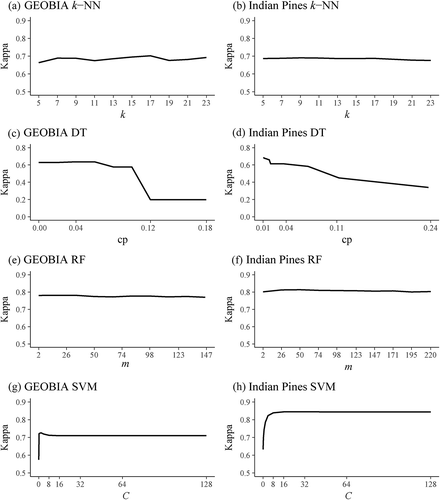
Table 11. Training/Tuning and prediction times.
Table 12. Software implementation of machine learning.