Figures & data
Figure 1. A pipeline of the proposed method has been shown here. A group of feature maps with the size of the input is obtained after the feature extractor, and the light attention learner is used to highlight the foreground and finally output five sets of heatmaps. This method is called DASR. The final result can be decoded after the SSG loss to correct the angle and diagonal length based on the short side of the object
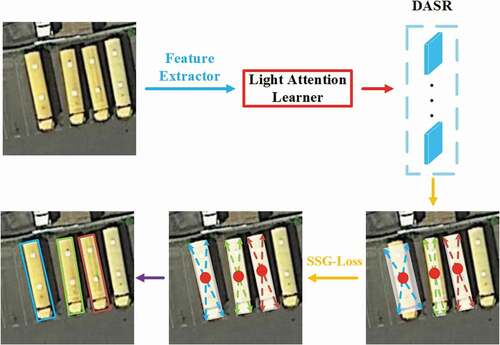
Figure 2. The network architecture of Dual-Det. A deformable convolution(DCN) is applied between downsampling and upsampling, which is shown as the Orange feature map in the figure. The light yellow heatmap is the output involved in the loss calculation. Conv Block is a small convolution module with detailed structure in .Section 3.4
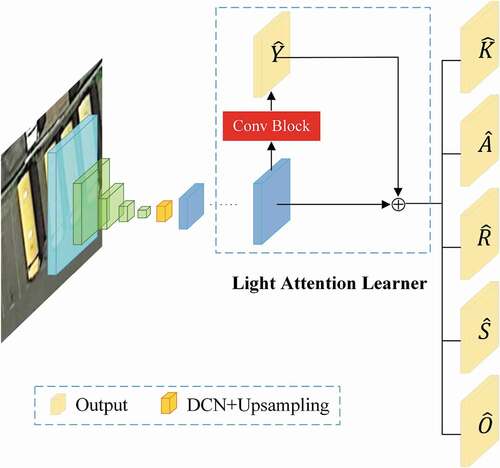
Figure 3. Illustration of the proposed regression strategy for an oriented object. We utilize to represent the box
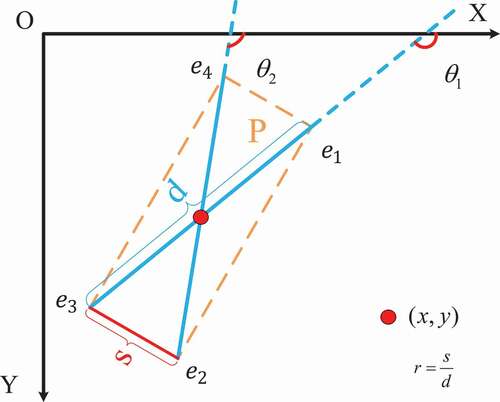
Figure 4. Comparison of the errors between dual-angle prediction and single-angle prediction. The red box is the ground truth,
is the error box and the green area is the intersection of the two boxes. See Section 3.2 for details
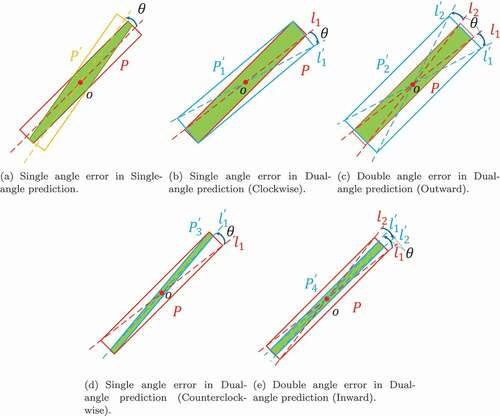
Table 1. Different results for short-side-based, long-side-based and the baseline
Table 2. Ablation studies of each component
Table 3. Ablation studies of each component (the details of each category are given in ). LAL is the acronym for the
Figure 6. Comparison of using the DASR (right) and baseline (left) in the harbour and large vehicle categories
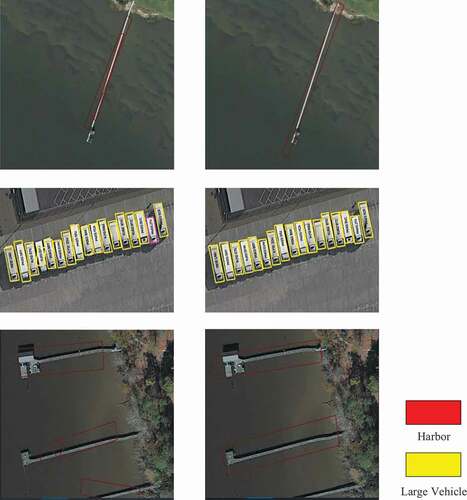
Table 4. Comparison of different settings for the SSG loss
Table 5. Comparison of the speed and memory of each component
Table 6. Comparison with state-of-the-art detectors on DOTA
Table 7. Comparisons of the accuracy and speed with the state-of-the-art methods on HRSC2016. * means that the VOC12 AP metric is used
Table 8. Comparison with the state-of-the-art detectors on UCAS-AOD (Zhu et al. Citation2015). * means that the VOC12 AP metric is used