ABSTRACT
A new void fraction estimation method for gas–liquid two-phase flow combining two differential pressure (DP) signals acquired from a single Venturi tube and based on Empirical Mode Decomposition (EMD) and Artificial Neural Networks (ANN) was experimentally investigated. In order to study gas–liquid distribution in horizontal pipes, two DP signals from the top and bottom sections of the Venturi tube are acquired and EMD is adopted to extract stable and fluctuating components of the DP signals. Experimental data revealed that fluctuating index increases nearly linearly with increasing void fraction when void fraction is less than 0.4. When void fraction is larger than 0.4, this near-linearity ceases. A combination of ANN method and the fluctuating index of DP signals is developed to estimate void fraction. Experimental results show that void fraction based on DP signal from top section of Venturi tube is overestimated because of clustering of bubbles and the scarcity of liquid information when gas–liquid mixture velocity is low. Void fraction is underestimated when the mixture velocity is high. A high gas–liquid slip ratio results in void fraction underestimation. Void fraction prediction performance is satisfactory when void fraction is less than 0.4 and fluctuating index of DP signal less than 1.2.
Additional information
Funding
Notes on contributors
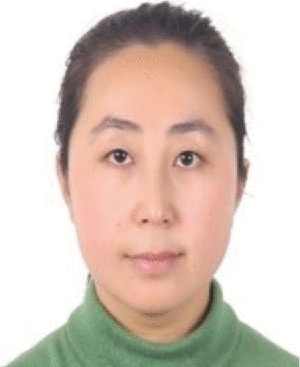
Weiwei Wang
Weiwei Wang is an associate professor of information and control engineering at China University of Petroleum (East China), Qingdao, China. She received her Ph.D. degree in industrial control technology from Zhejiang University, Hangzhou, China. Her current research interests include multiphase flow measurement and analysis, data mining and data fusion, and signal processing.
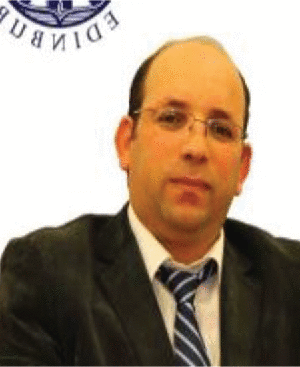
Khellil Sefiane
Khellil Sefiane has M.Sc. and Ph.D. degrees in Chemical Engineering. He presently holds the Chair of Thermophysical Engineering at the University of Edinburgh. He is also ExxonMobil Fellow at the University of Edinburgh. In 2015 he was appointed Honorary Professor at Kyushu University.
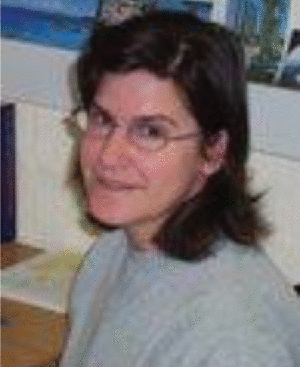
Gail Duursma
Gail Duursma is a Lecturer in Chemical Engineering at the University of Edinburgh. She obtained her D.Phil. from the University of Oxford and after post-doctoral study at the University of Oxford, she joined the University of Edinburgh. She is a Member of the IChemE.
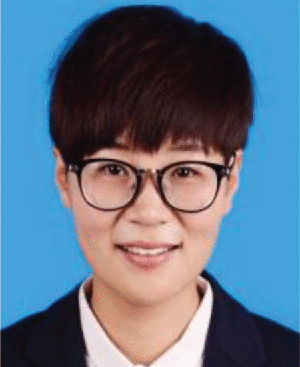
Xiao Liang
Xiao Liang is a Masters student of Engineering at the College of Information and Control Engineering, China University of Petroleum (East China), Qingdao, China. She is under the supervision of Prof. Weiwei Wang. Her current research interests are focused on two-phase flow measurement and signal processing.
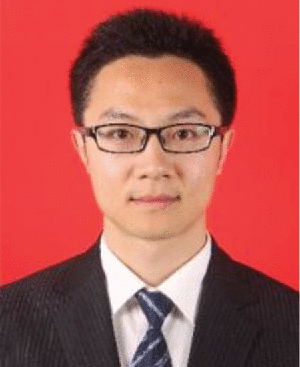
Yu Chen
Yu Chen is a Masters student of Engineering at the College of Information and Control Engineering, China University of Petroleum (East China), Qingdao, China. He is under the supervision of Prof. Weiwei Wang. His current research interests are focused on two-phase flow analysis and data fusion.