Figures & data
![Figure 1 An example of triplet trajectories from speakers F02 and M02 over natural time. F(emale)02 tonal sequence: 4-5-1, M(ale)02 tonal sequence: 2-1-4; Mandarin Chinese rhyme sequences [oŋ-@-iou] and [ien-in-], respectively. See supplementary material for full contextual covariate information.](/cms/asset/9a5b3044-cdb6-460a-9b3c-07370ae0fe51/uasa_a_1006729_f0001_b.gif)
Table 1 Covariates examined in relation to F0 production in Taiwanese Mandarin. Tone variables in a 5-point scale representing tonal characterization, 5 indicating a toneless syllable, with 0 representing the fact that no rhyme precedes the current one (such as at the sentence start). Reference tone trajectories are shown in the supplementary material section: Linguistic Covariate Information
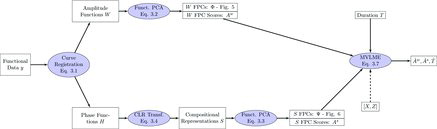
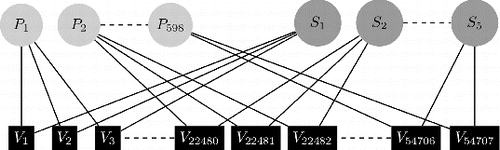
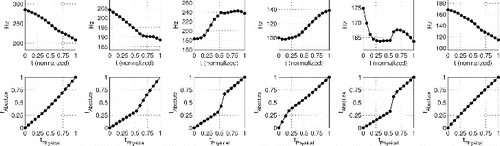
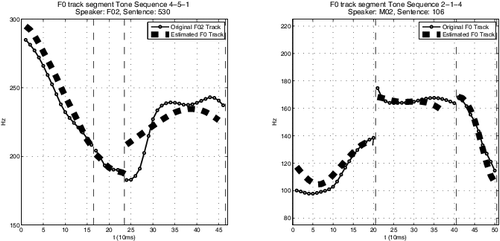
![Figure 6 W (amplitude) eigenfunctions Φ: mean function ([0.05, 0.95] percentiles shown in gray) and first, second, third, fourth, fifth, and sixth functional principal components (FPCs) of amplitude.](/cms/asset/28794b5a-0919-423e-8184-54e1160dbc52/uasa_a_1006729_f0006_b.gif)
Table 2 Percentage of variances reflected from each respective FPC (first 9 shown). Cumulative variance in parenthesis
Table 3 Actual deviations in Hz from each respective FPC (first 9 shown). Cumulative deviance in parenthesis. (Human speech auditory sensitivity threshold ≈ 10 Hz)
![Figure 7 (Phase) eigenfunctions Ψ: mean function ([0.05, 0.95] percentiles shown in gray) and first, second, third, fourth, fifth, and sixth functional principal components (FPCs) of phase. Roughness is due to differentiation and finite grid; the corresponding warping functions in their original domain are given in Figure S.1 in the supplementary material.](/cms/asset/d5614e81-8c4b-4a8a-ac1f-a72ad1b3de71/uasa_a_1006729_f0007_b.gif)
Table 4 Random effects std. deviations
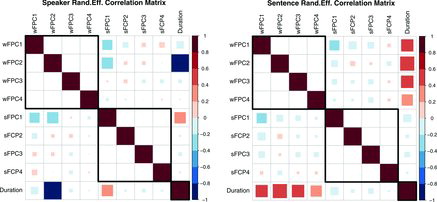
Supplemental material