Figures & data
Fig. 1 Left: Molars from two suborders of the primates: Haplorhini and Strepsirrhini. The Haplorhini suborder has genera Tarsius (yellow) and Saimiri (grey). The Strepsirrhini suborder has genera Microcebus (blue) and Mirza (green). Right: Relationship between the four primate genera. Tarsier molars exhibit additional high cusps (highlighted in red). A similar figure was published in Wang et al. (Citation2021).
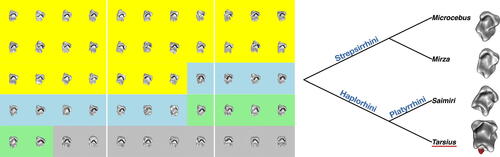
Fig. 2 Consider the two-dimensional shape in the left panel. For each pair of ν and t, the equation
represents a straight line (or a hyperplane in a high-dimensional space). The subset
denotes the region below this line. Let
, then
. The right panel presents the function
, where
is identified by
through
. Procedures for generating the shape K and the right panel are given in Appendix D.1.
![Fig. 2 Consider the two-dimensional shape K∈S2 in the left panel. For each pair of ν and t, the equation x·ν=t−R represents a straight line (or a hyperplane in a high-dimensional space). The subset Ktν denotes the region below this line. Let ϕν(x)=x·ν+R, then Ktν={x∈K|ϕν(x)≤t}. The right panel presents the function (ν,t)↦SECT(K)(ν,t), where ν∈S1 is identified by θ∈[0,2π] through ν=( cos θ, sin θ). Procedures for generating the shape K and the right panel are given in Appendix D.1.](/cms/asset/79897b2d-1db8-4207-9cf2-9682813c5b6d/uasa_a_2353947_f0002_c.jpg)
Table 1 Rejection rates (from 1000 experiments) for different indices ε (significance ).
Table 2 P-values of Algorithms 1, 2, and 4 for the dataset of mandibular molars.
Fig. 3 (Left panel) The relationship between ε and the rejection rates computed via Algorithms 1, 2, 3 (see ), and 12 existing fdANOVA methods (see in Appendix J for details on the existing fdANOVA methods). The (red) dashed line presents the significance level . (Right panel) The shapes in the first row are from
, and the shapes in the second row are from
.
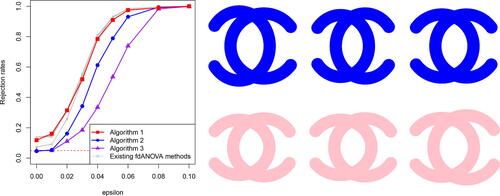
Supplementary_Materials.pdf
Download PDF (5.6 MB)acc_form.pdf
Download PDF (81.3 KB)Data Availability Statement
The source code for implementing the simulation studies and applications is publicly available online at https://github.com/JinyuWang123/TDA.git.